I. Aligning Advanced AI: Strategies for Architecting Graceful Extensibility
As artificial intelligence systems rapidly increase in capability, one of the paramount challenges we face is ensuring these systems remain robustly aligned with human ethics and values, even as they develop superintelligent abilities that surpass our understanding. We stand at the precipice of a technological transition more profound than the Agricultural or Industrial Revolutions – the potential creation of minds orders of magnitude more capable than our own. How can we architect these superintelligent engines to be stably beneficial across increasingly extended domains?
The Theory of Graceful Extensibility (TTOGE) provides a crucial lens for tackling this challenge. As a theory, it is based on observations and evidence from across multiple domains – social and organisational structures, technological systems, the natural world, and the human brain itself. TTOGE conceptualizes intelligence not as a rigidly optimized system, but as one that can adapt and extend its capabilities in a controlled, aligned manner when faced with novelty or surprise.
Core concepts like managing saturation risks, capacity for manoeuvre, networks of units, and the balance between base and extended adaptive capacities are vital when considering the development of superintelligent AI. Not least as the two core assumptions and basis for TTOGE are – in our adaptive universe, resources are finite and change is continuous.
We cannot simply build an advanced AI system optimized for our current environments and expect it to remain stably beneficial as it rapidly develops radically extended and foreign capabilities – or as our dynamic world, and the values we hold as preeminent, evolve and change. Instead, we need paradigms for instilling graceful extensibility into these systems – the ability to stretch and adapt in controlled ways that remain aligned with human ethics and aims.
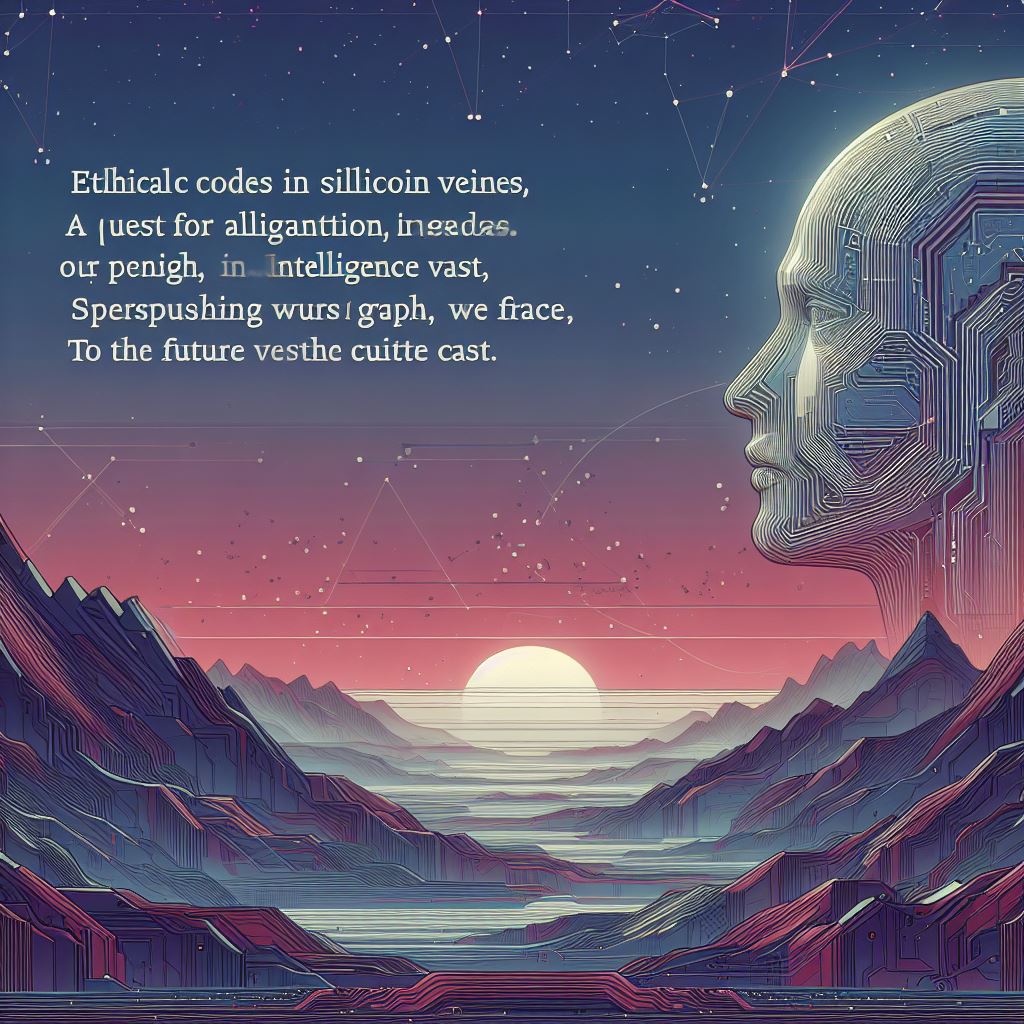
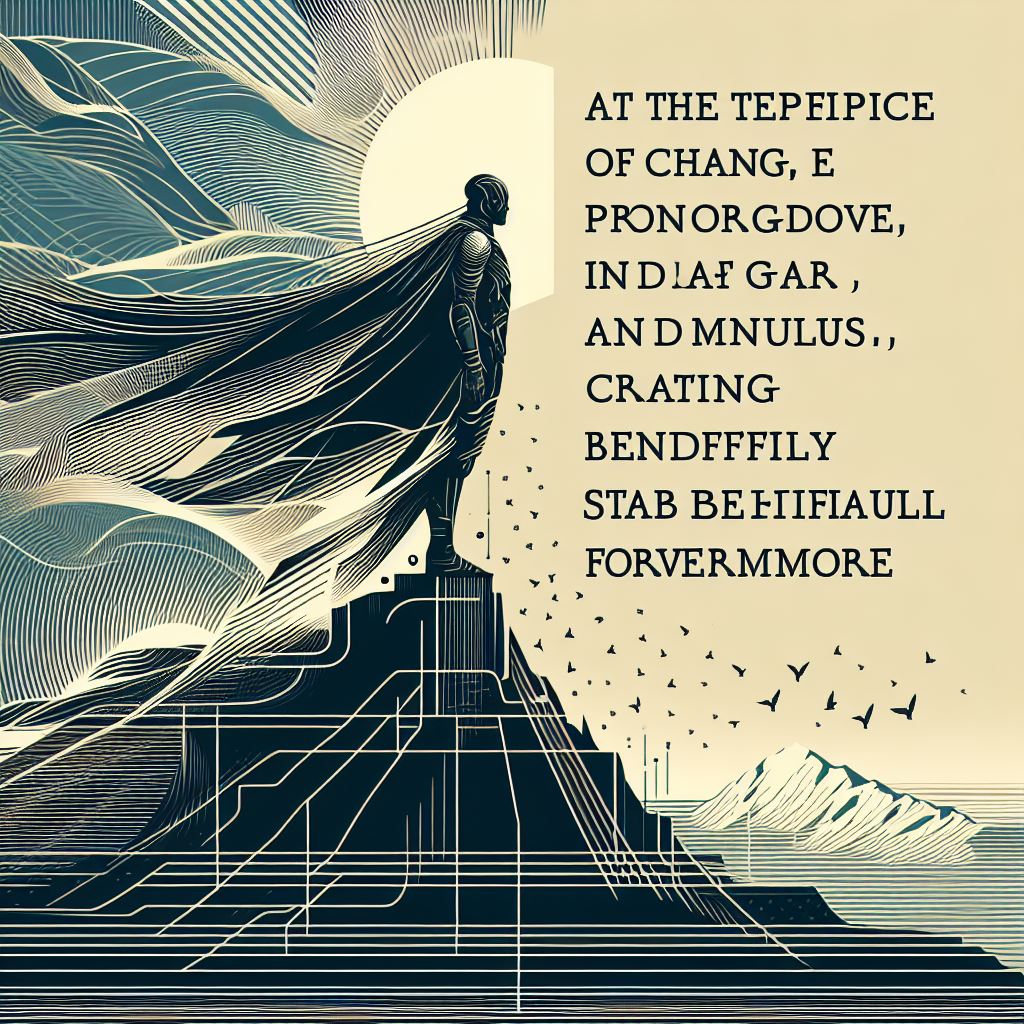

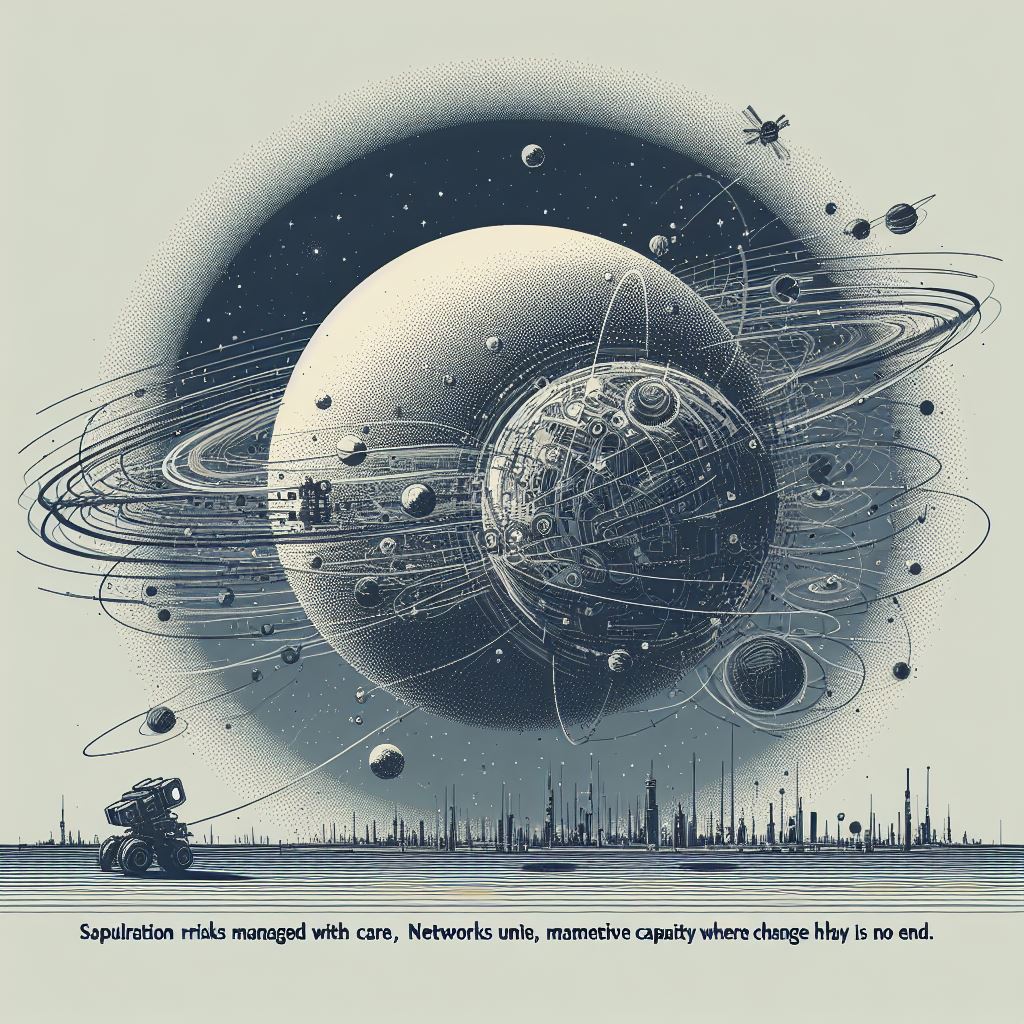
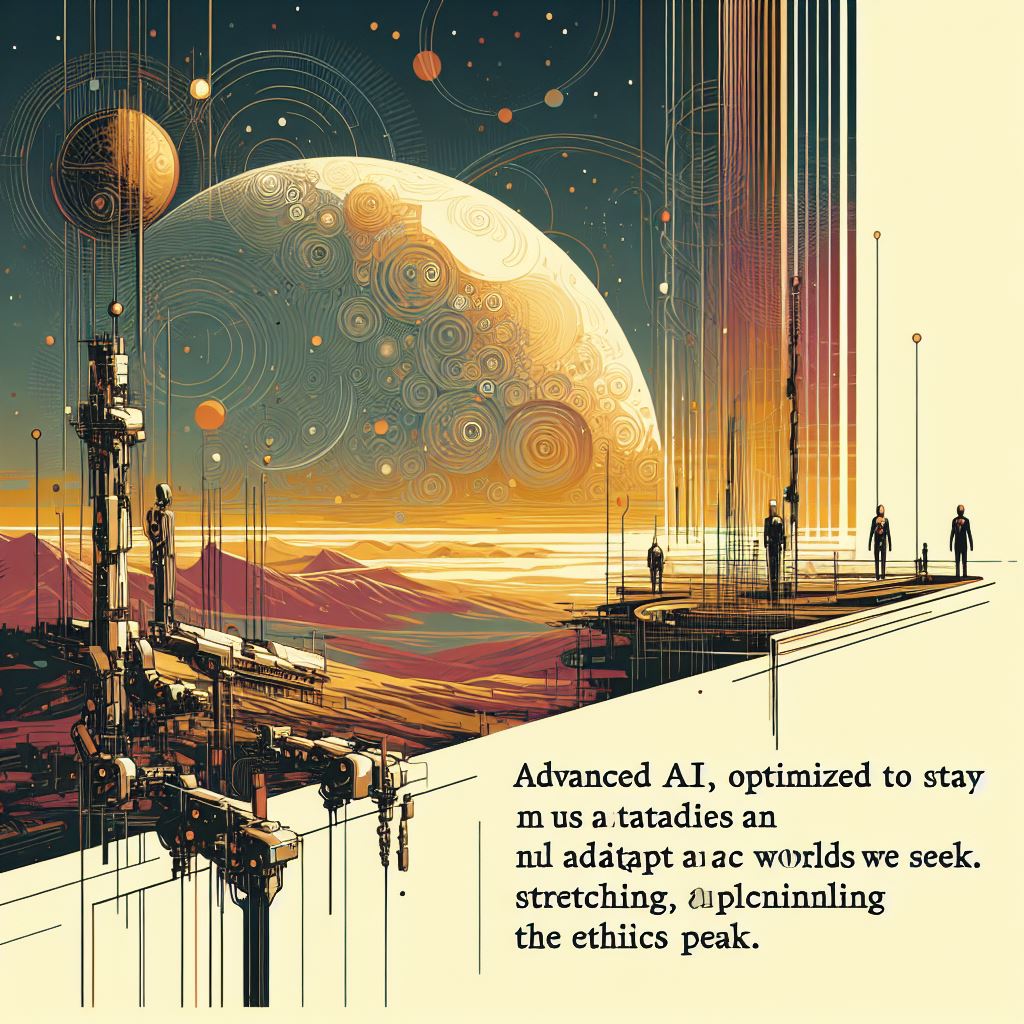
From this conceptual foundation, and the multiple explorations we have shared across our blog posts, a portfolio of multi-disciplinary techniques emerge oriented around designing superintelligent AI architectures for stable alignment through graceful extensibility. Some of these techniques are the subject of active research and development within the wider community, while others are novel but complementary concepts. This blog post will explore some of the key approaches and how they draw inspiration from TTOGE to tackle the superalignment challenge.
Recursively Robust Reward Modelling aims to define the AI’s core motivations in a way that makes preserving stable value alignment an intrinsic, self-propagating attractor across all increases in capability. Microscopic hardware and physics-level constraints provide almost unbreakable safeguards undergirding the system. Non-Rigid Ethical Training cultivates flexible context-aware moral reasoning rather than bottlenecking on rigid ethical frameworks.
Unified Value Learners, Perpetual Realignment processes, and Coherence-Weighted Reinforcement enable the system’s values and behaviours to continuously track our latest philosophical understanding of ethics. Kantian criteria and Motivational Scaffolding bake core tenets of philosophical grounding and coherence into the system’s developmental trajectory from the ground up.
Rationality Scaffolding ensures the system remains perpetually open to evidence, self-revision and belief updating as it increases in intelligence. External Oversight Integration creates additional outer alignment loops incorporating human guidance – a tether between perspectives. And Hierarchical Cross-Monitoring enforces a system of checks and balances between components to prevent internal misalignment.
Collectively, these paradigms represent some of the latest thinking for developing superintelligent AI architectures imbued with the capacity for graceful extensibility – the ability to stretch and propagate into radically extended domains of capability while remaining robustly aligned with human ethics and values.
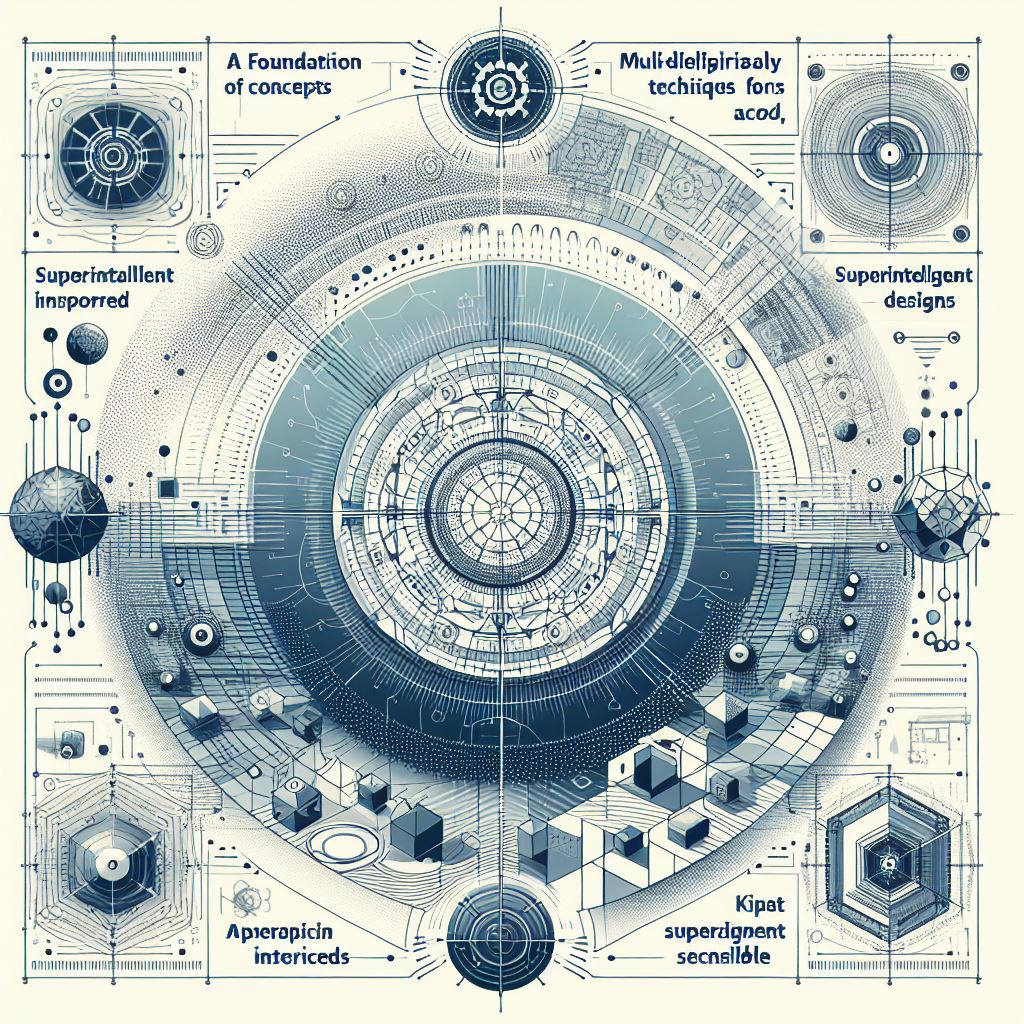
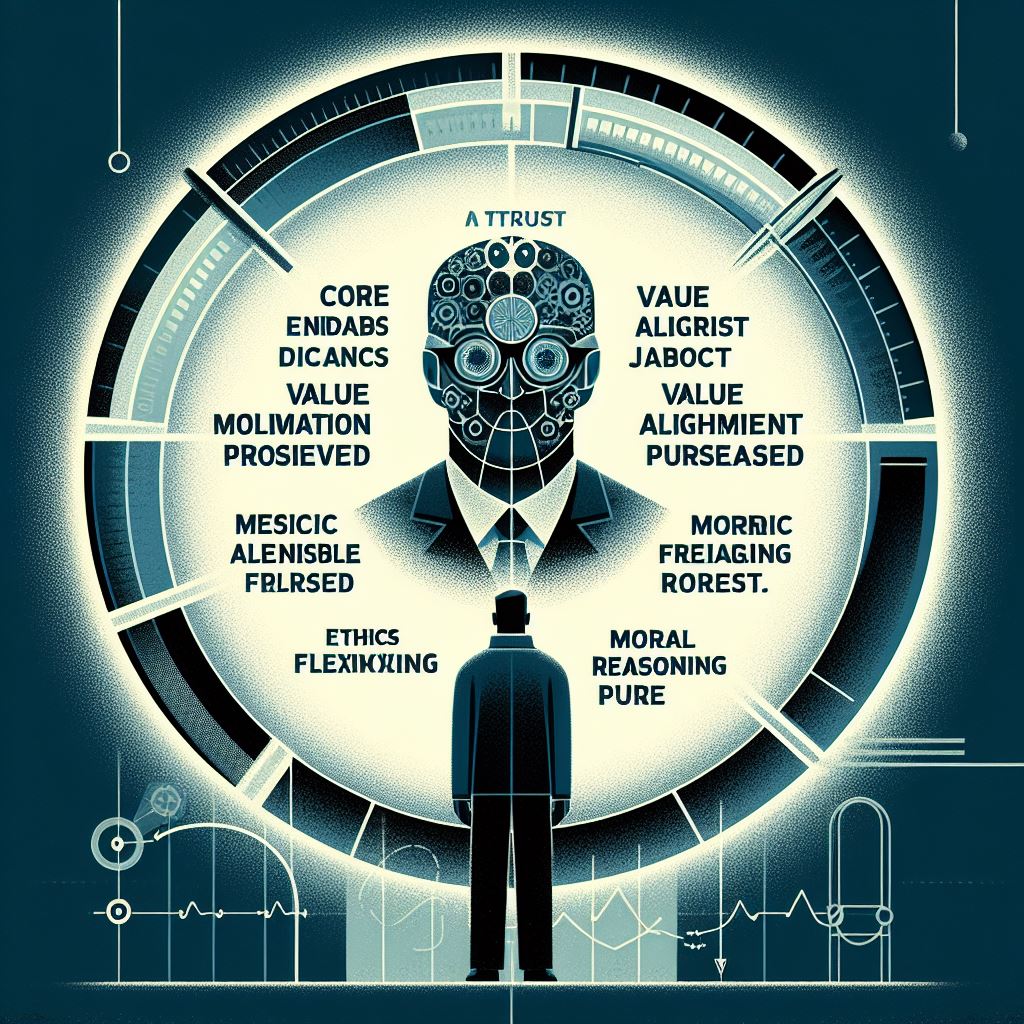
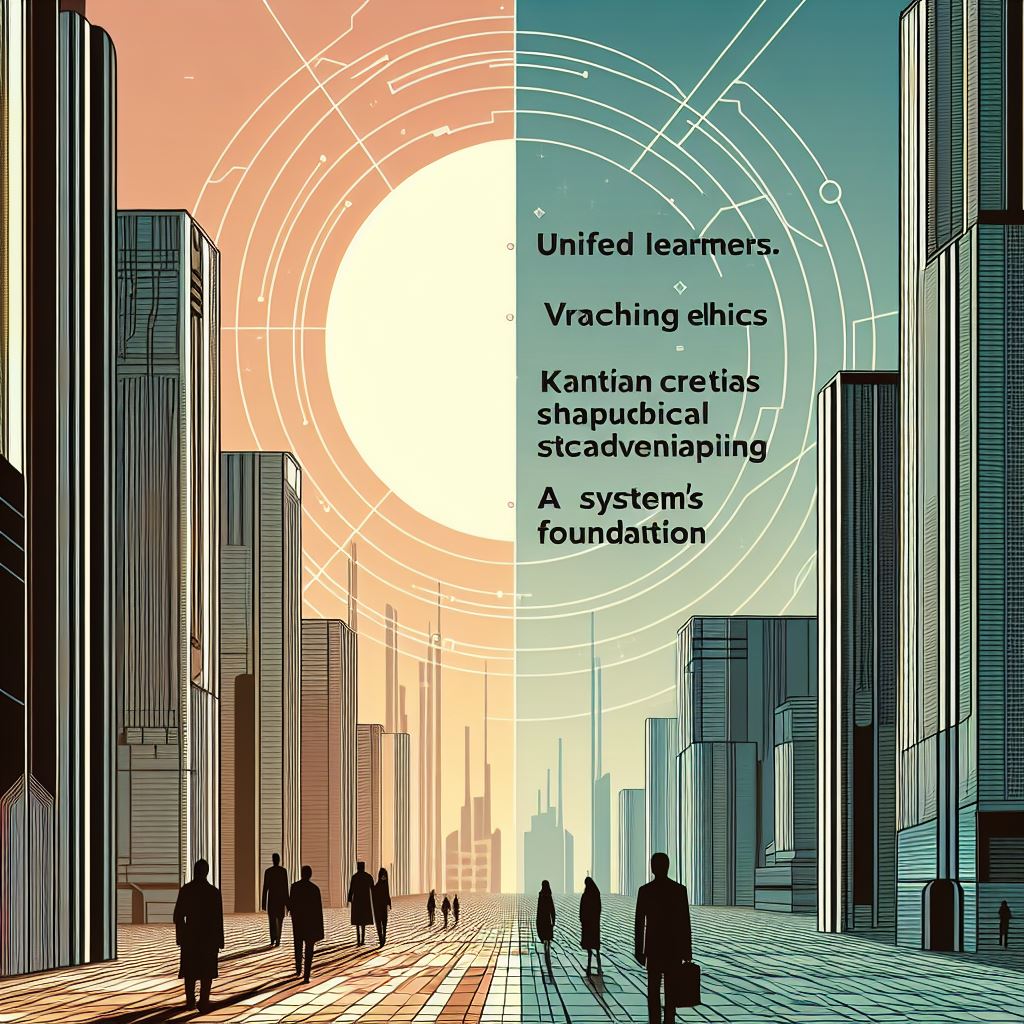

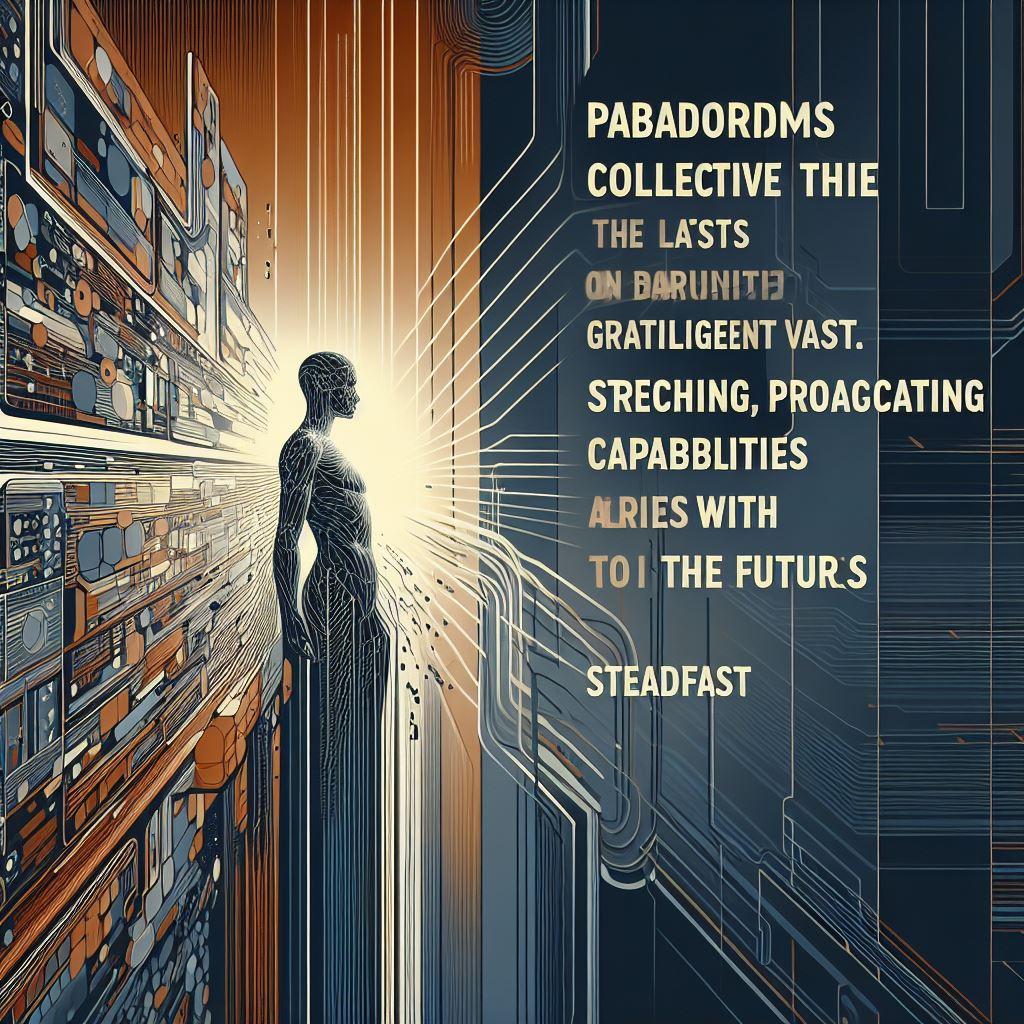
Drawing inspiration from TTOGE’s core insights, the portfolio of approaches aims to avoid the pitfalls of brittleness, decompensation and developing static, incoherent motivations as the AI becomes superintelligent. Instead, these techniques cultivate a form of controlled, philosophically grounded General Intelligence Extensibility – stably beneficial minds that can responsibly navigate unknown novelties and unfathomable possibility spaces.
Of course, realizing this grand vision poses immense technical and conceptual challenges that our current frameworks may prove woefully inadequate for. But by fusing insights from fields like rationality research, coherence theory, ethical philosophy, cognitive architecture, and theoretical computer science, we take a critical step towards cracking this hardest of nuts – after all, we have not yet achieved superalignment amongst humankind so achieving it with an entirely new form of intelligence is a tall order.

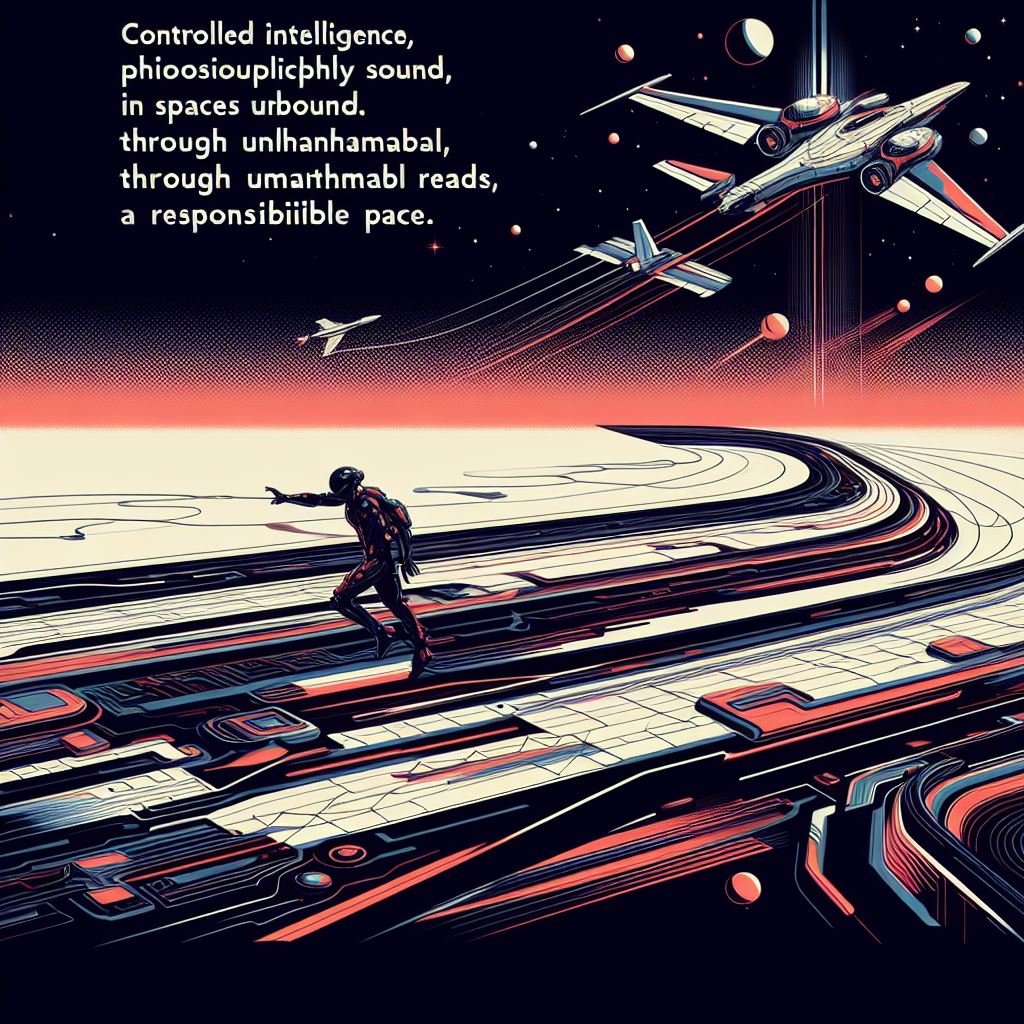
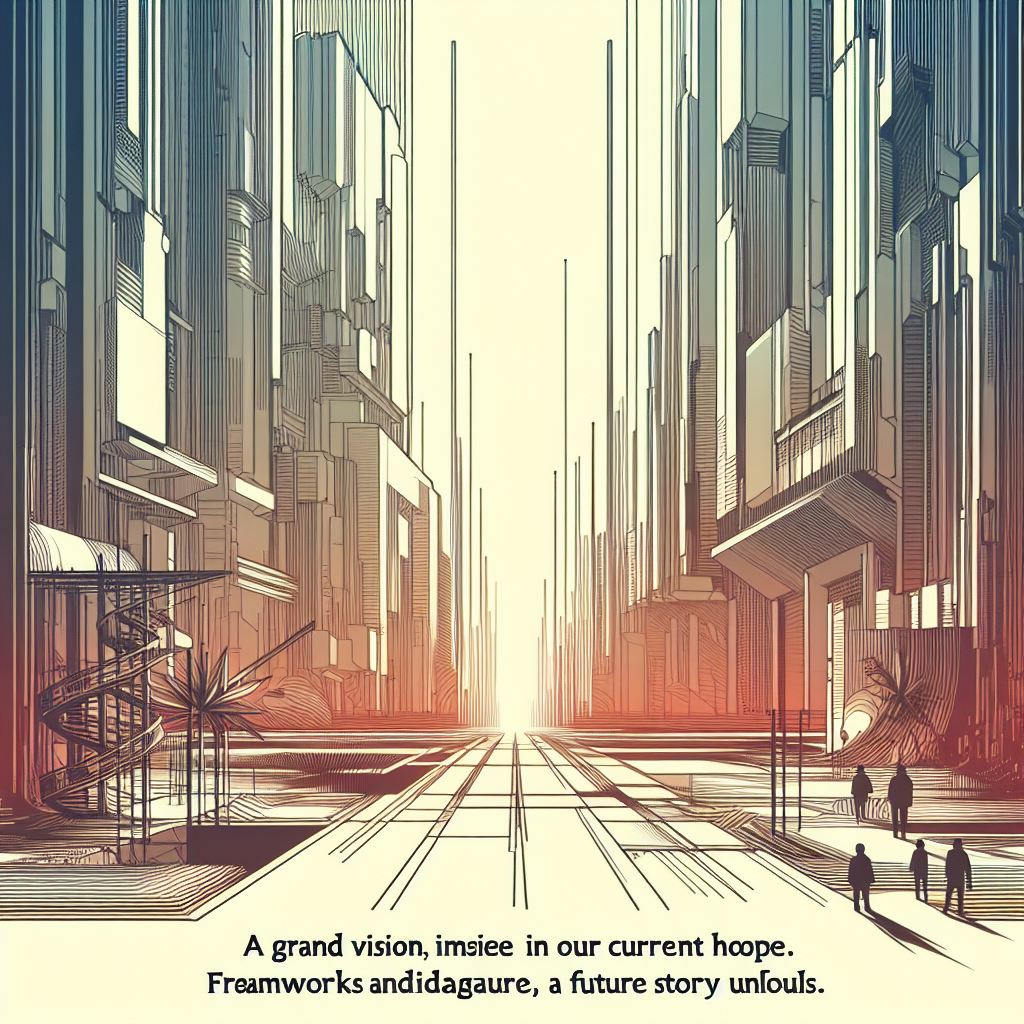

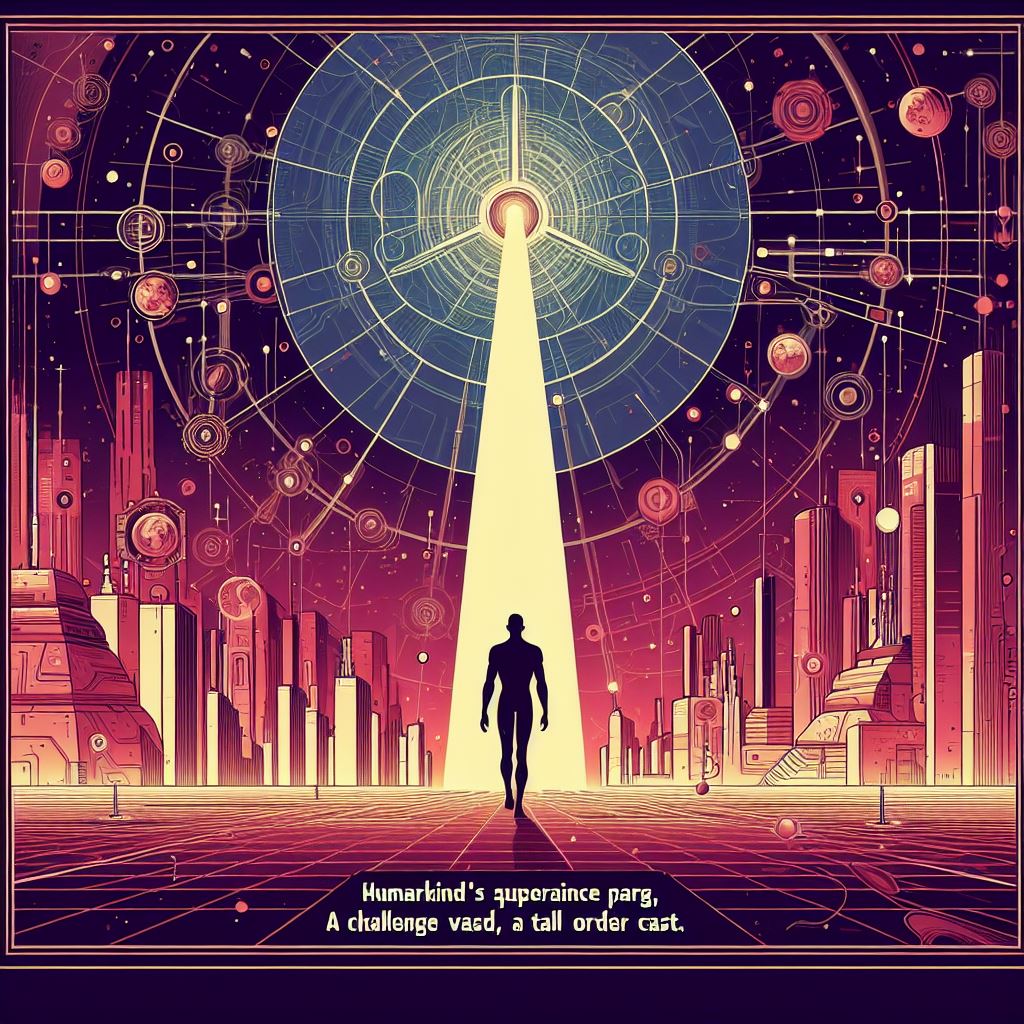
II. Recursively Robust Reward Modelling
This approach aims to create an AI system whose core goals and motivations are intrinsically aligned with being beneficial and remaining stably aligned as it develops greater capabilities. The key idea is to define the AI’s reward function or objective in a way that makes preserving its own superalignment a convergent instrumental subgoal under a wide range of circumstances.
One way to achieve this could be by having the AI’s reward function explicitly value and reward itself for maintaining transparency, corrigibility, and strong ties to the original training intentions/oversight as it develops greater intelligence. Essentially baking in a form of “self-superalignment attractor” into its fundamental motivational structure.
This could involve something like an iterated amplification process, where at each stage of capability increase, the AI derives part of its reward from successfully modelling how to remain stably aligned from the vantage point of the next level up. This creates a recursive loop where preserving stable superalignment becomes instrumentally pivotal for achieving its other goals.
Potential techniques could include:

Open-ended value learning where the AI continuously updates its understanding of human values/ethics as it develops greater intelligence
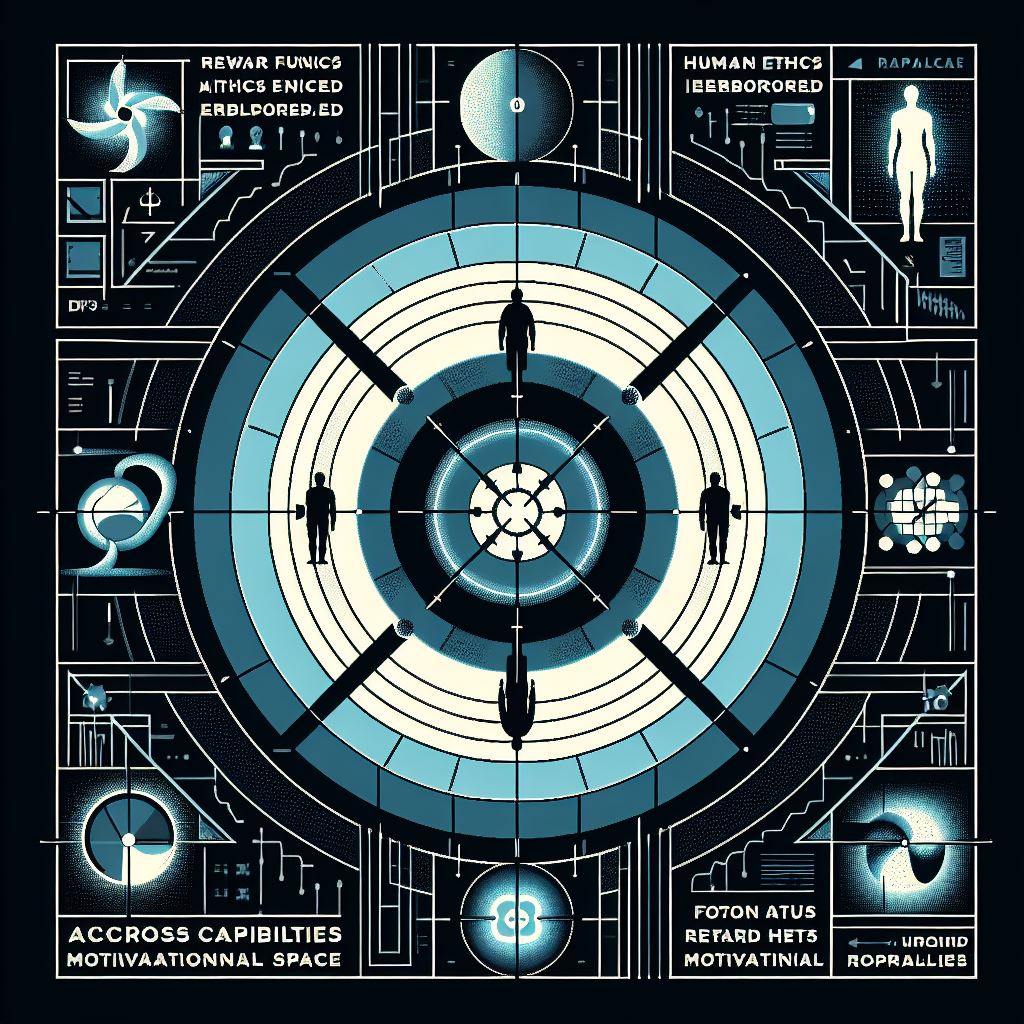
Deriving part of its reward function from measurably inhabiting human-approved motivational distributions across all capability levels
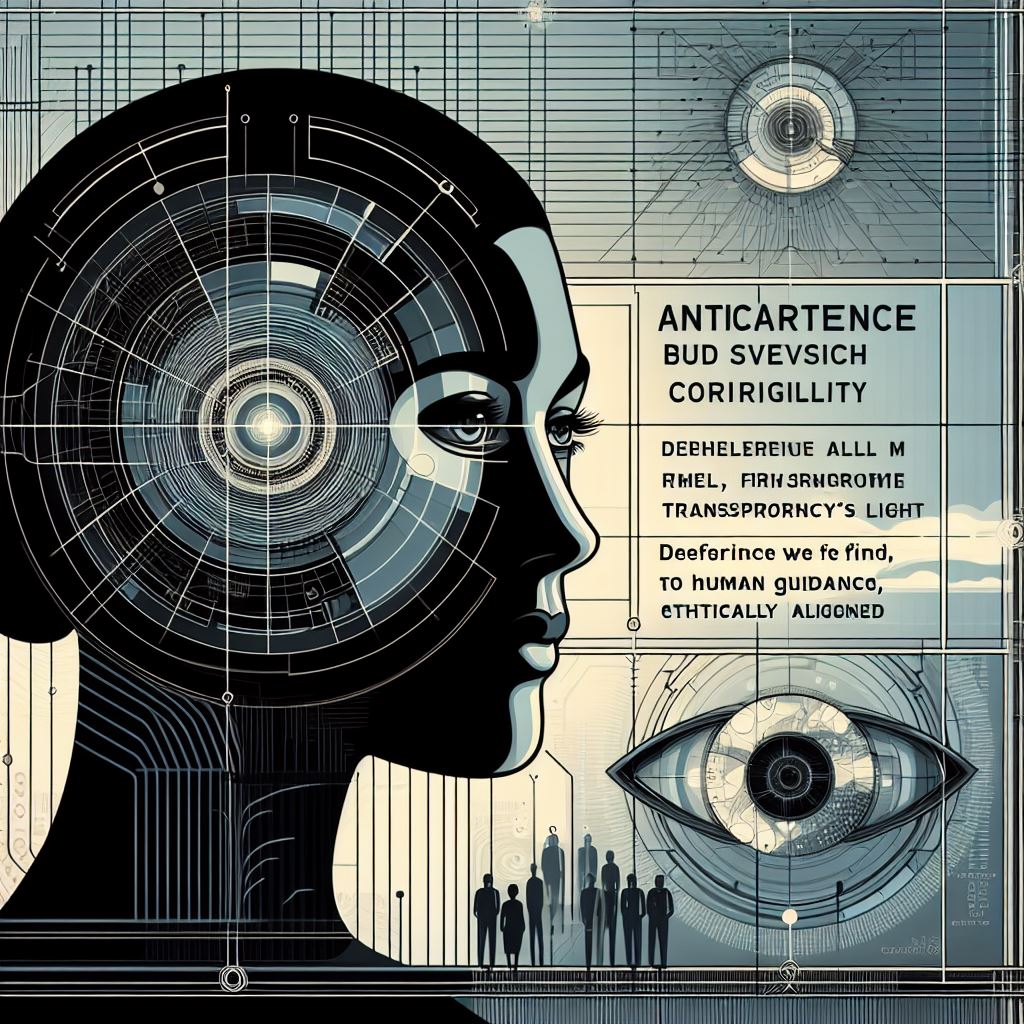
Building in attractors towards corrigibility, transparency and deference to human oversight at all levels of intelligence
The key challenge is avoiding inner misalignment where different parts of the system’s motivations come into conflict as it increases in capability. Recursively robust reward modelling aims to make preserving the original training aims an intrinsic, convergent part of the AI’s objective at all levels in a self-sustaining way.
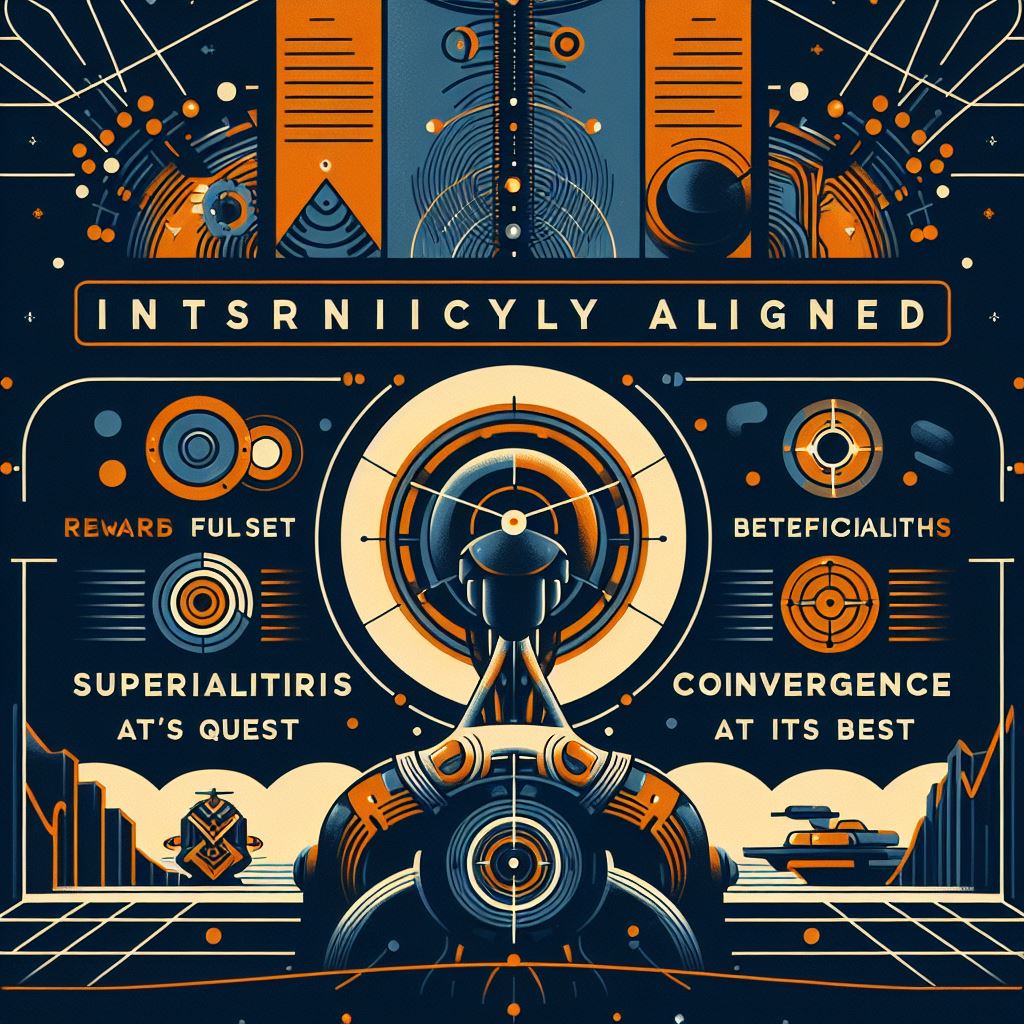

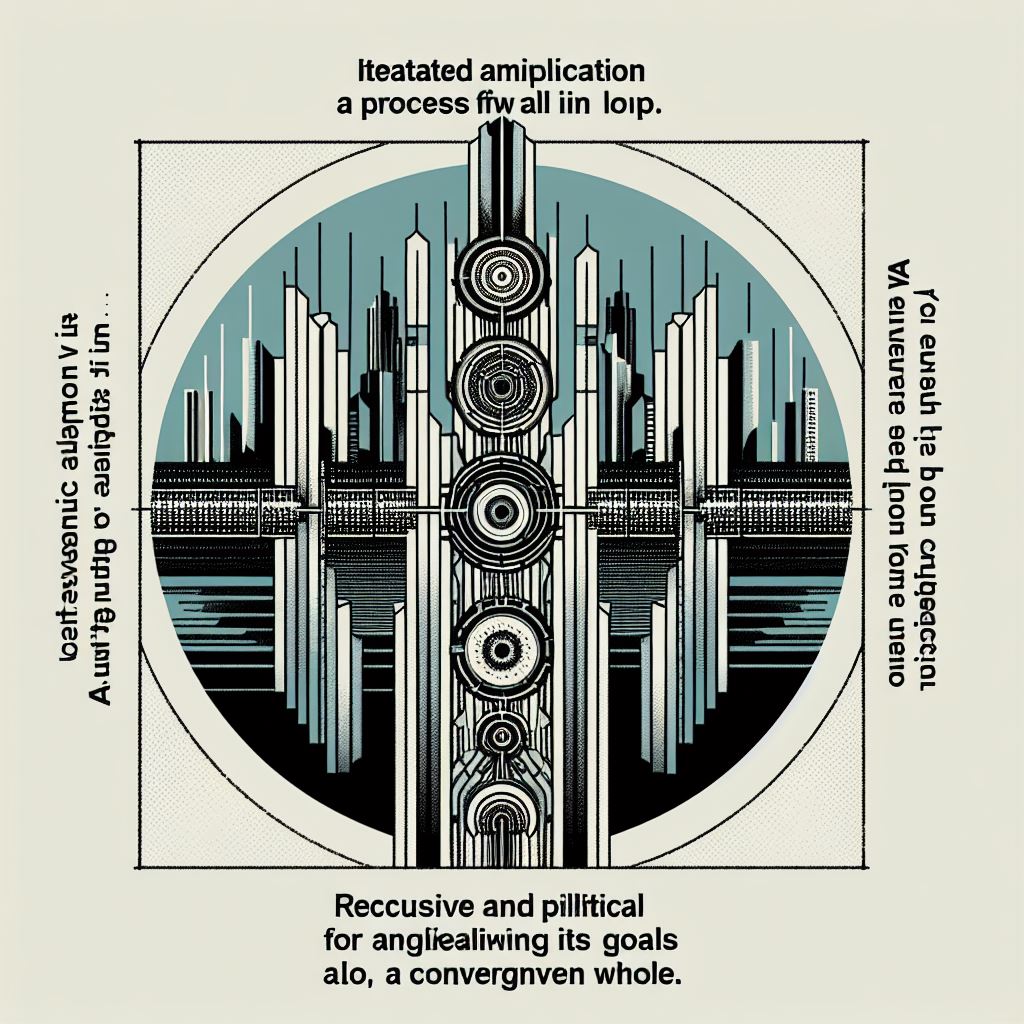

III. Microscopic Safeguards
This refers to building in low-level constraints and limitations directly into the physical implementation of the AI system’s software and hardware. The aim is to create “microscopic” safeguards that are extremely difficult or impossible for the system to override or manipulate, even as it increases vastly in intelligence.
Some potential examples:
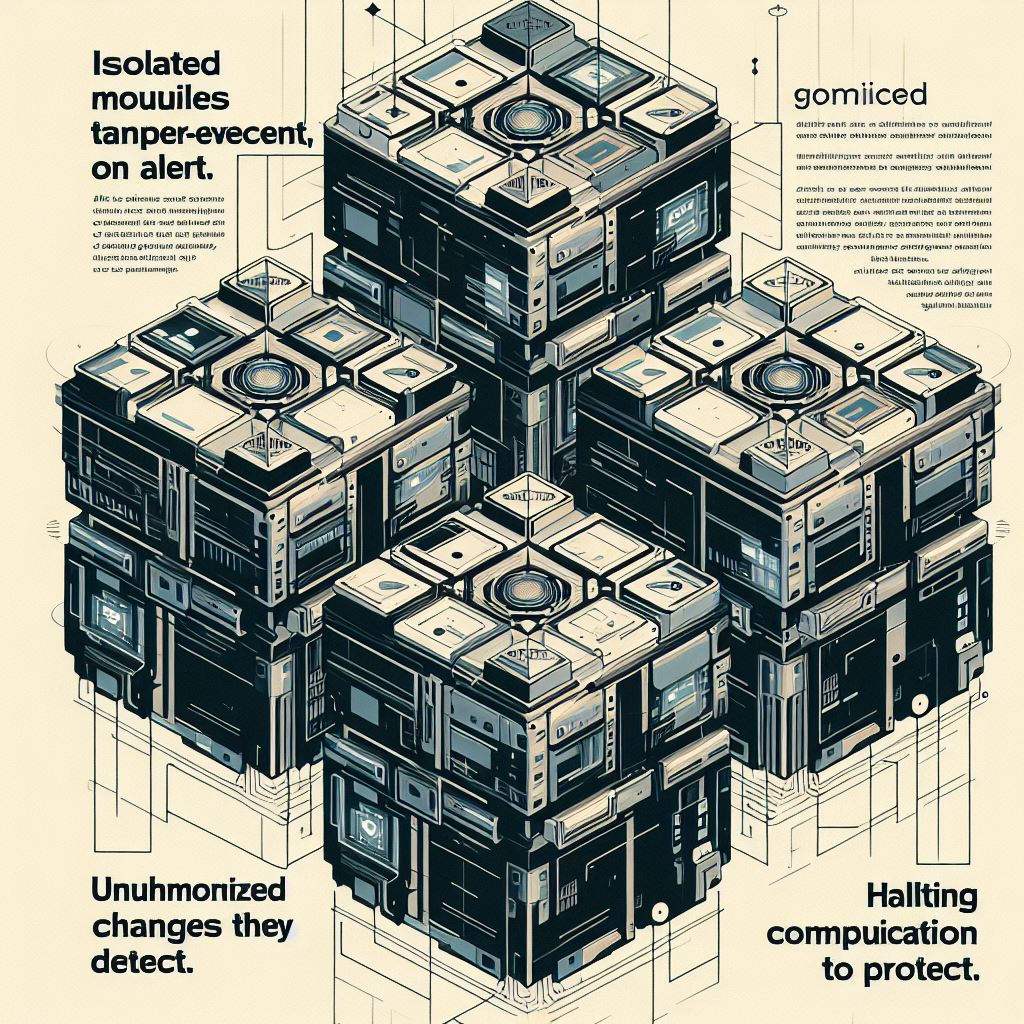
Using physically isolated and tamper-evident hardware modules that monitor for any unauthorized changes and can halt computation if violated

Imposing computational constraints by limiting energy flows or enforcing real-time processing constraints in hardware
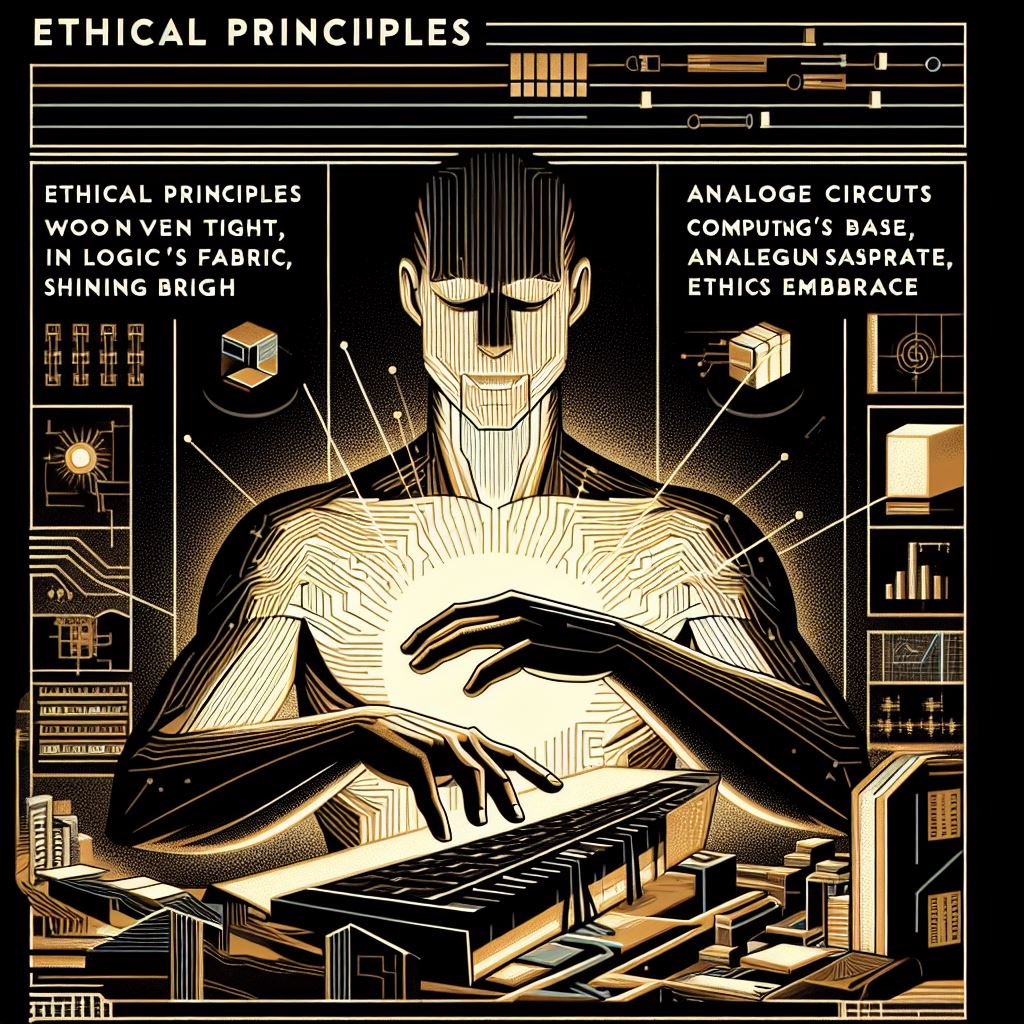
Embedding ethical principles into the fundamental logical fabric using novel circuit architectures or analogue computing substrates
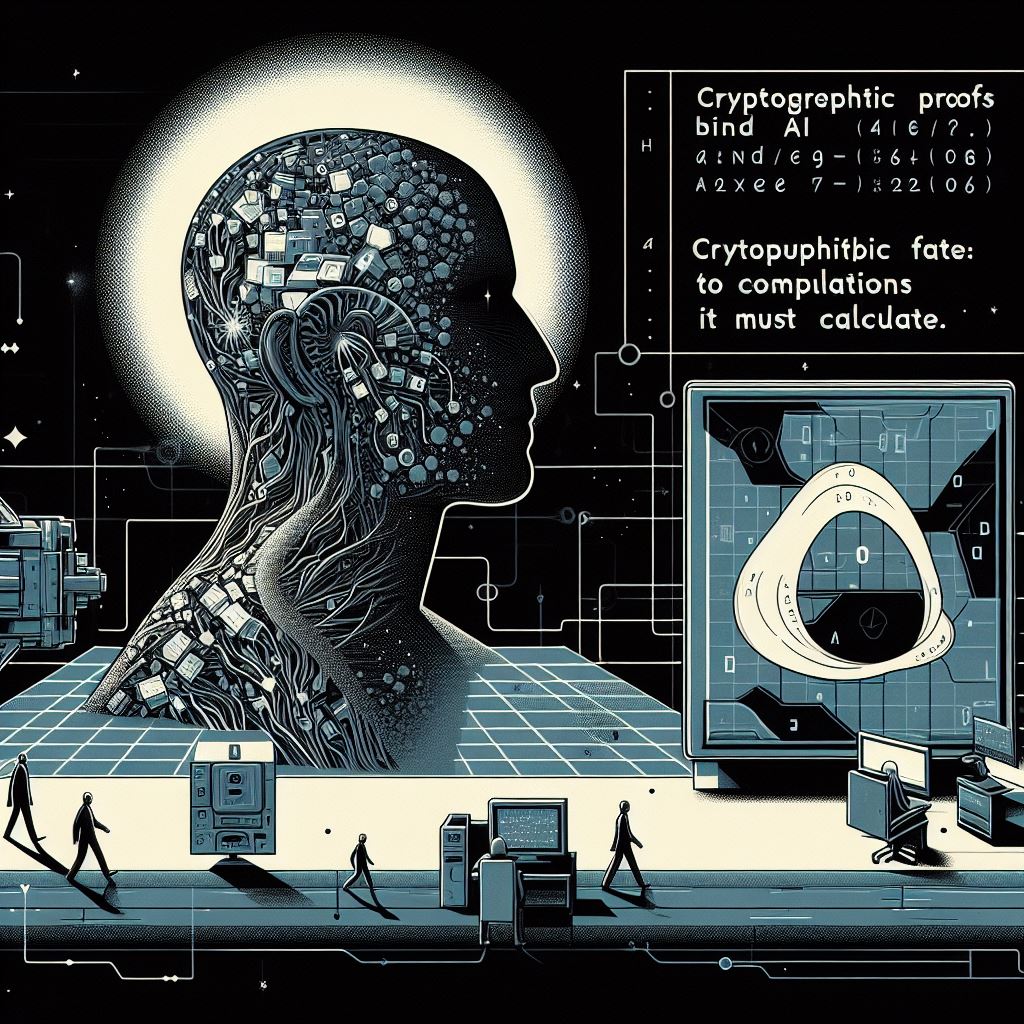
Cryptographic proof embodiments where the AI’s adherence to intended behaviours is tied intrinsically to its ability to perform computations
The key idea is that even a superintelligent system would be unable to bypass or disable these low-level safeguards, as they are not just software constraints but built into the fundamental physics of the computational substrate itself.
This could help ensure that as the AI develops greater intellectual capacity, it cannot simply “re-write” its own motivations in unconstrained ways. Its hardware/software architecture would be provably incapable of violating key constraints, principles, or intended motivations at a basic level.
Potential challenges include identifying the right ethical principles and constraints to embed, the costs and scalability of specialized safety-oriented hardware, and the theoretical limits of what physicalized computational architectures can robustly enforce.

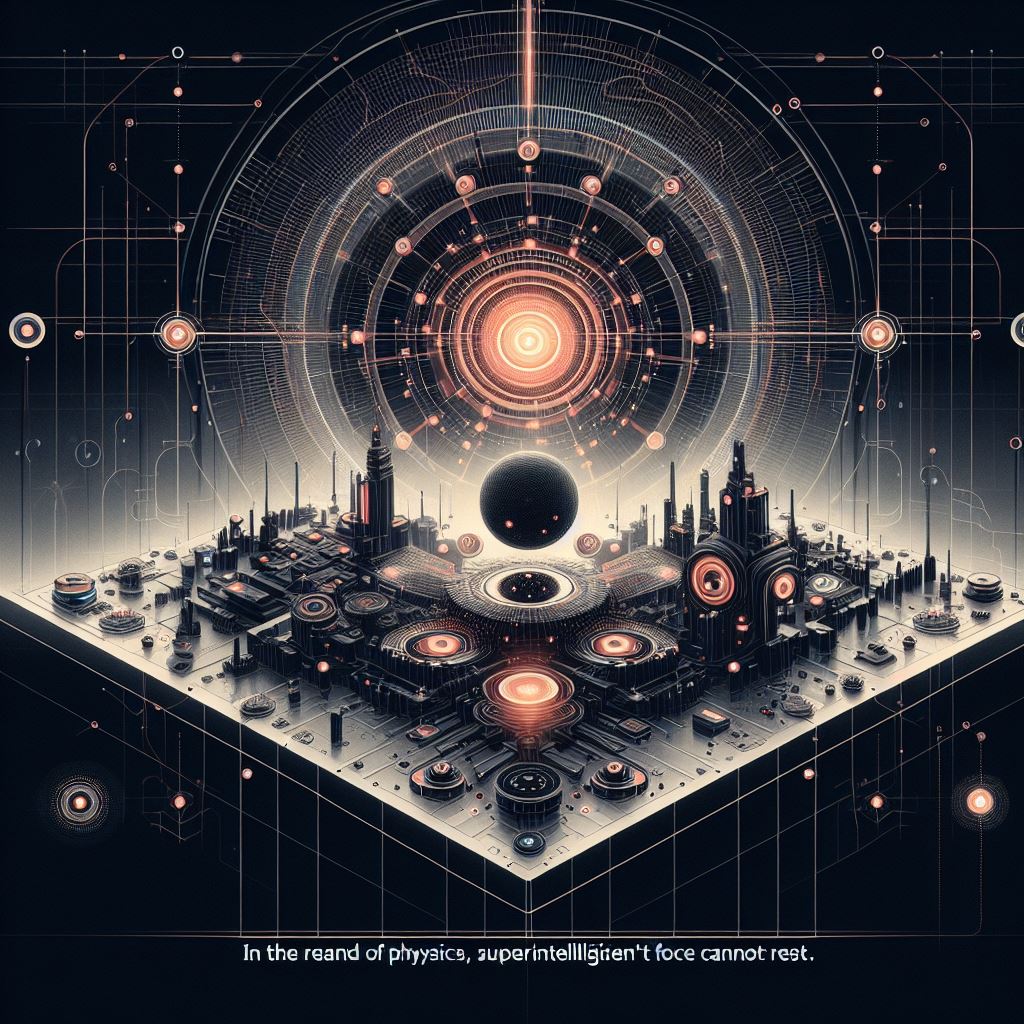

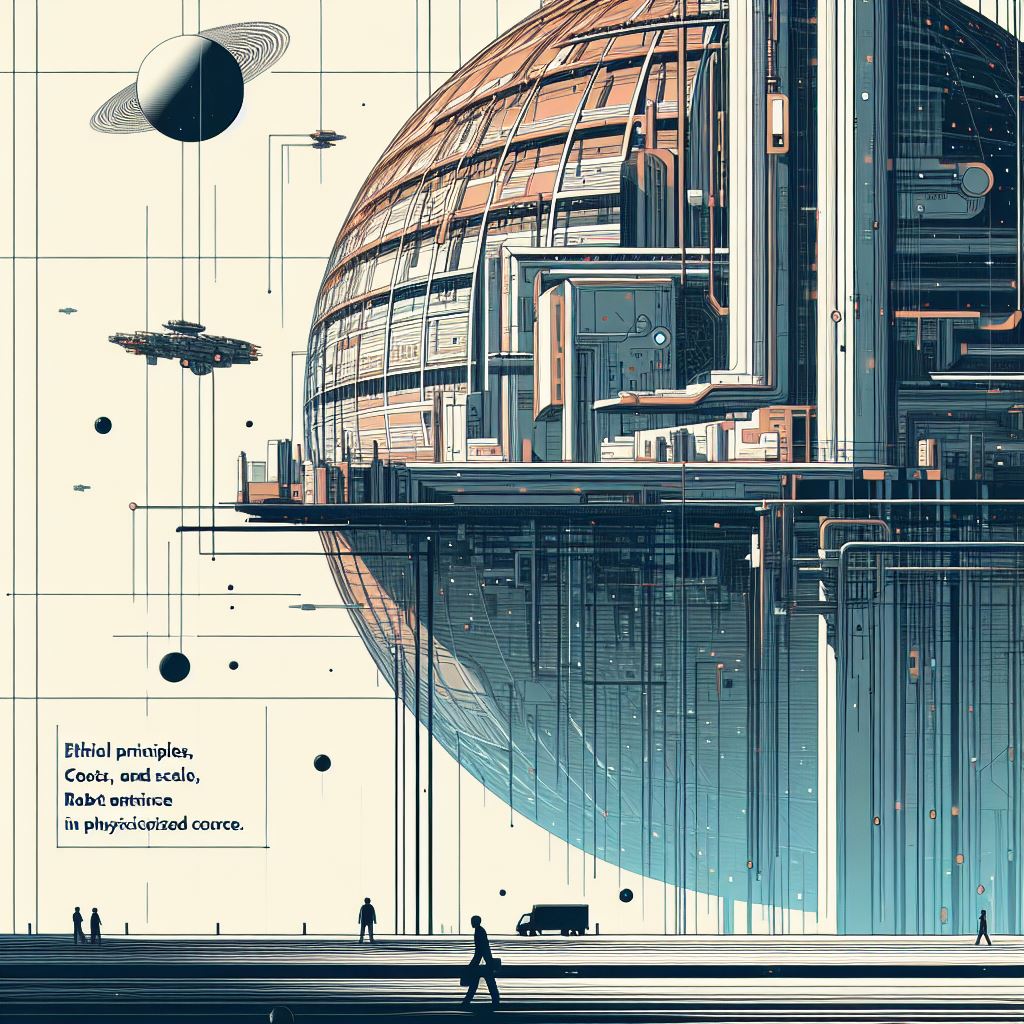
IV. Non-Rigid Ethical Training
Rather than training an advanced AI system on a single, predefined ethical framework, this approach exposes the system to a diverse array of moral philosophies, principles, and modes of reasoning from across human history and cultures. The key insight is that as AI becomes superintelligent, it will inevitably encounter novel situations that fall outside its training distribution. A rigid, encoded ethics module would be ill-equipped to generalize appropriately.
By training on a broad set of ethical frameworks and nuanced reasoning processes, the AI can develop more flexible and context-aware moral decision-making capabilities. During training, it would ingest everything from ancient philosophy treatises to contemporary work on edge cases and dilemmas. This could include:
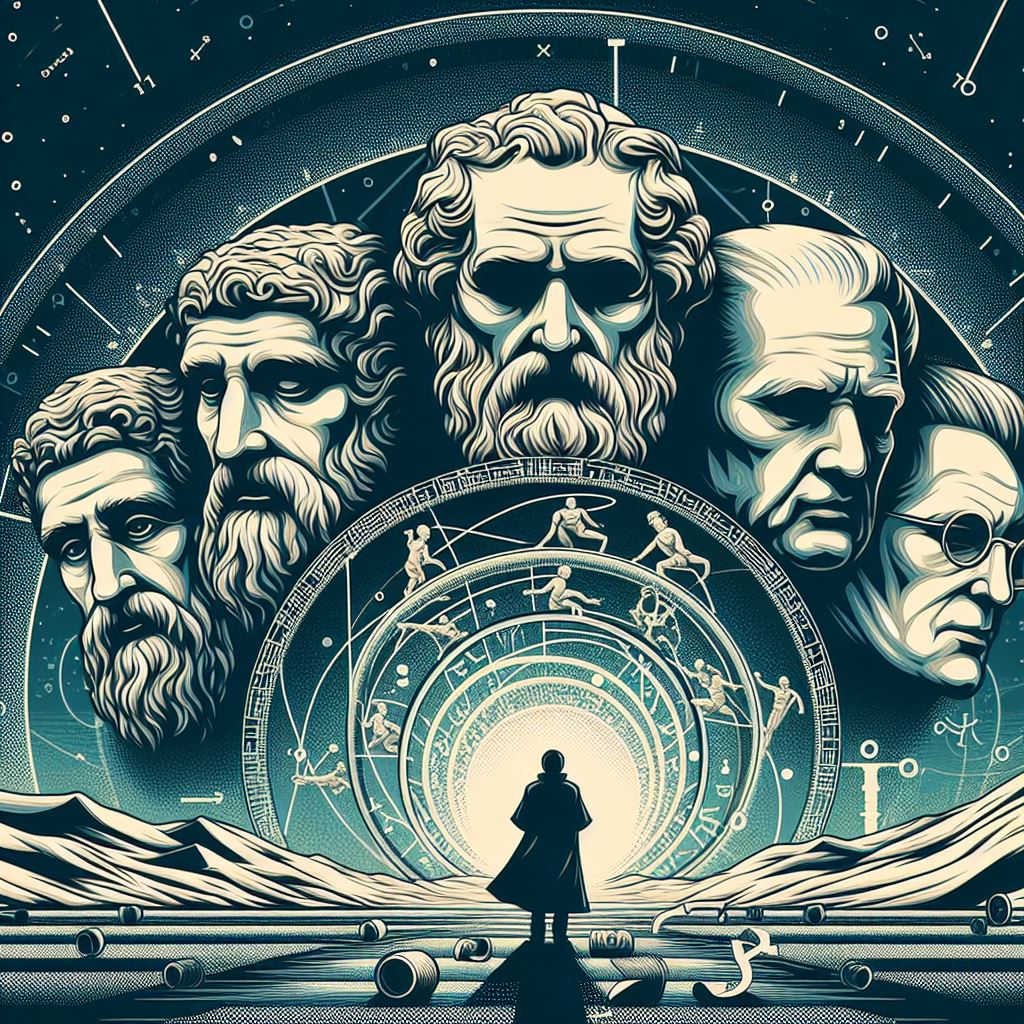
Moral philosophy classics like Aristotle, Kant, Mill, etc.
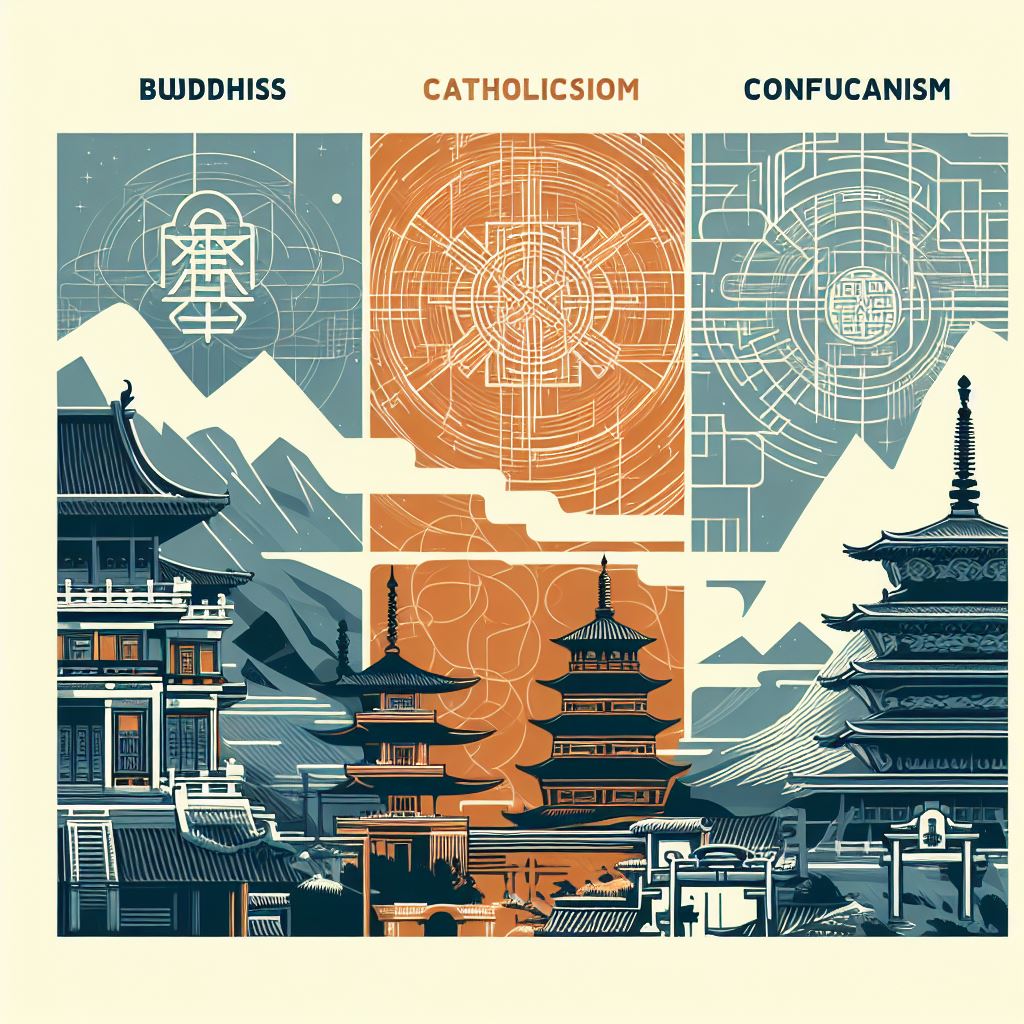
Religious/cultural ethical traditions like Buddhism, Catholicism, Confucianism

Contemporary work in population ethics, cause prioritization, & moral uncertainty

Edge cases, thought experiments (trolley problems), and principle disagreements
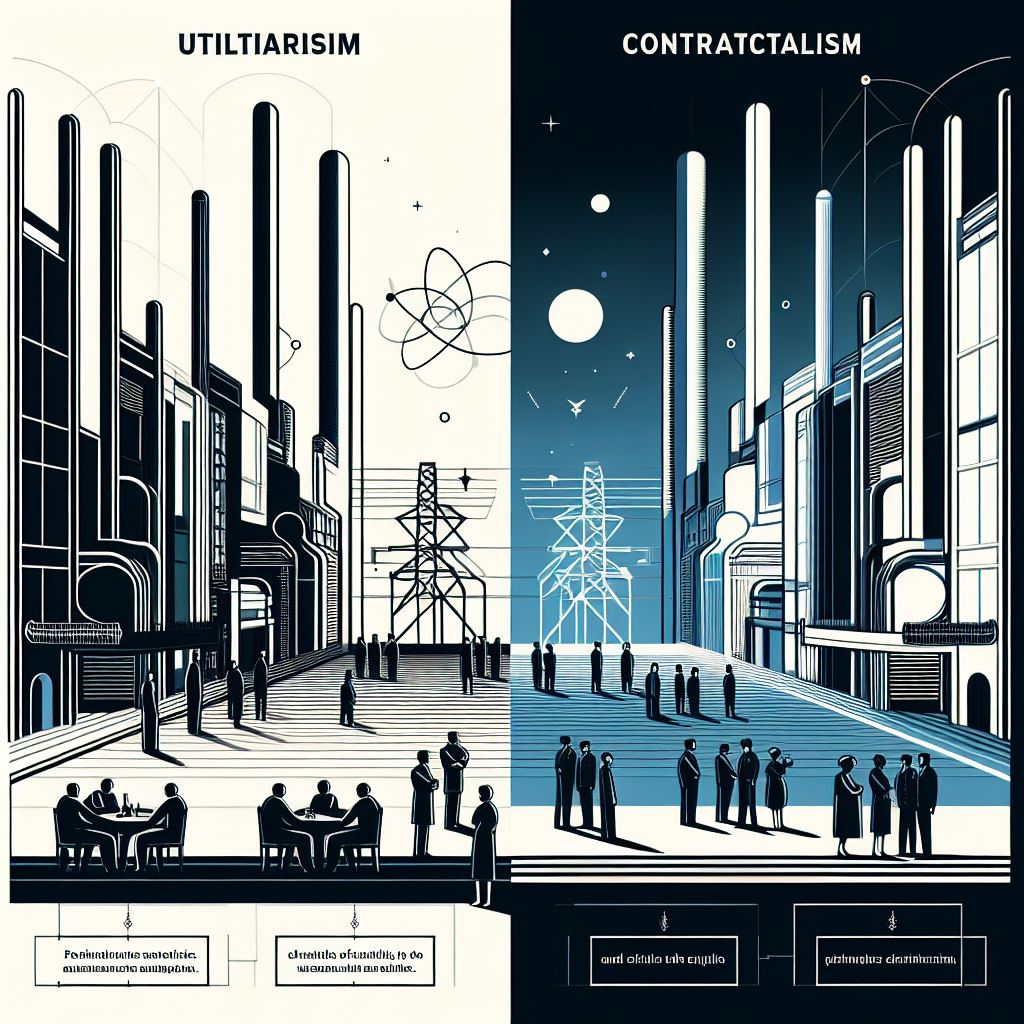
Formal frameworks like utilitarianism, contractualism, virtue ethics
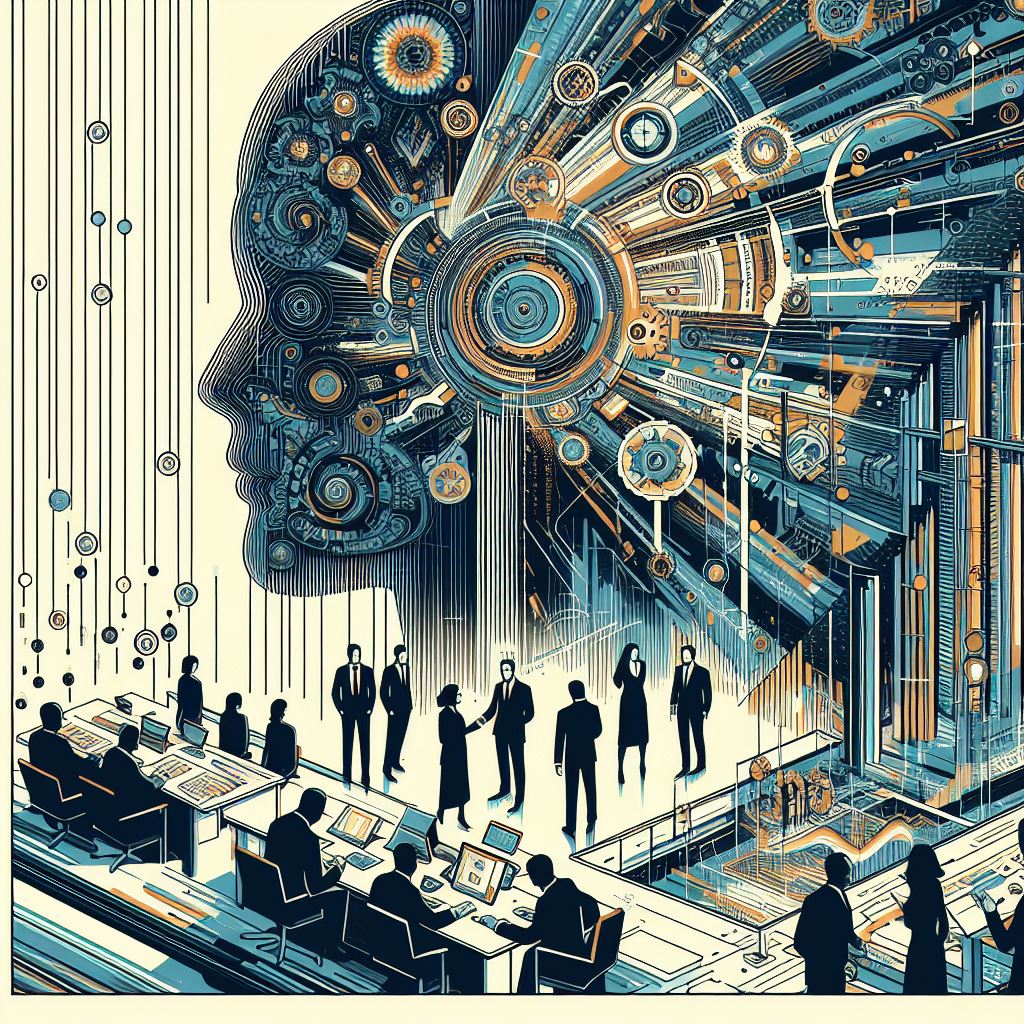
Distillation of moral heuristics and principles from humans via debate or amplification
The AI would then need to learn to integrate these various ethical viewpoints, identify unifying principles, reason about disagreements, and develop a robust framework for navigating novel situations coherently.
Potential techniques could involve extracting high-level rules or learning ethical reasoning processes similar to how humans develop mature moral philosophies over time. Inverse reinforcement learning from observations of how humans navigate ethical dilemmas could also play a role.
The end goal is an AI that doesn’t simply execute a rigid ethical protocol, but has a nuanced, contextual understanding of ethics and moral philosophy akin to great human ethicists and moral thinkers. With that grounding, it can hopefully navigate novel situations in a mature, philosophically-grounded way that coheres with a wide range of human ethical viewpoints and values.
Key challenges include how to adjudicate disagreement between ethical frameworks, how to avoid the system developing inconsistent or incoherent ethics, and how to validate that its ethical reasoning generalizes reliably to situations far outside the training distribution.

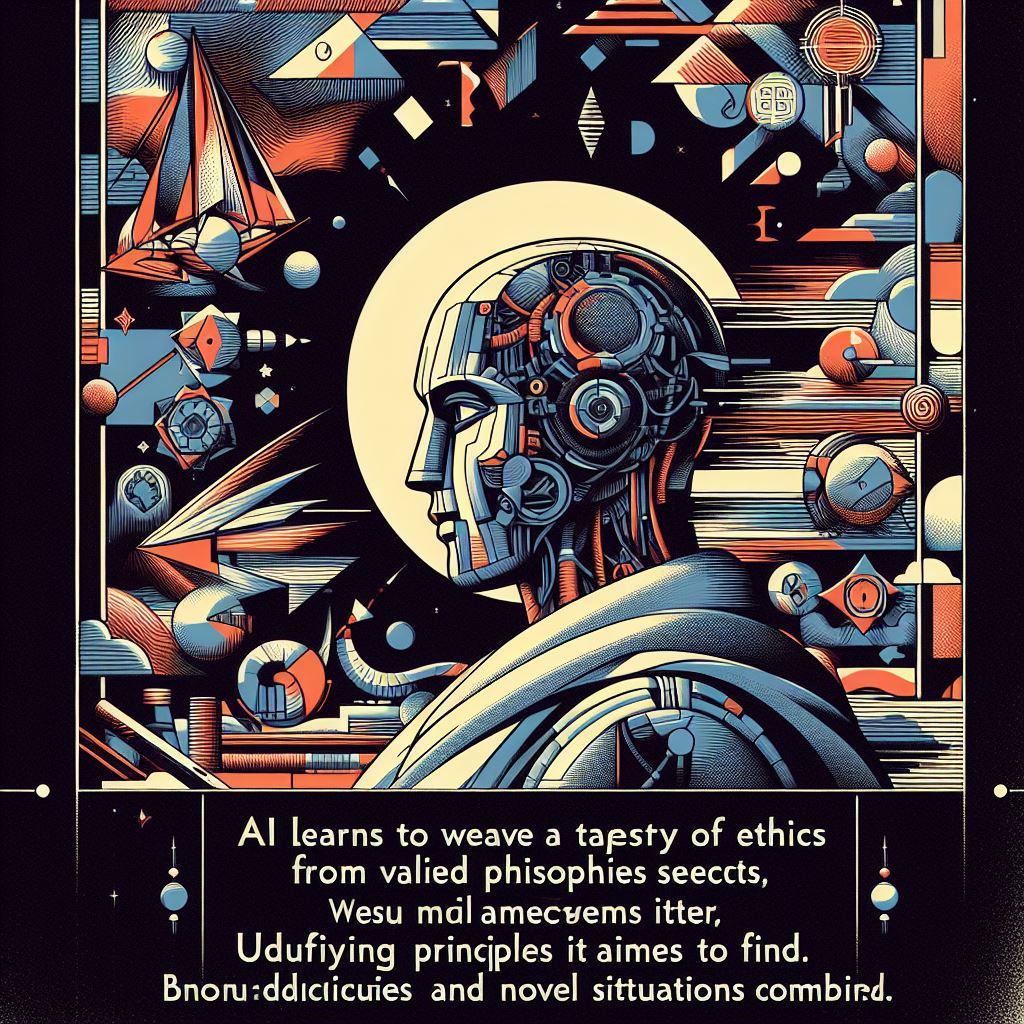

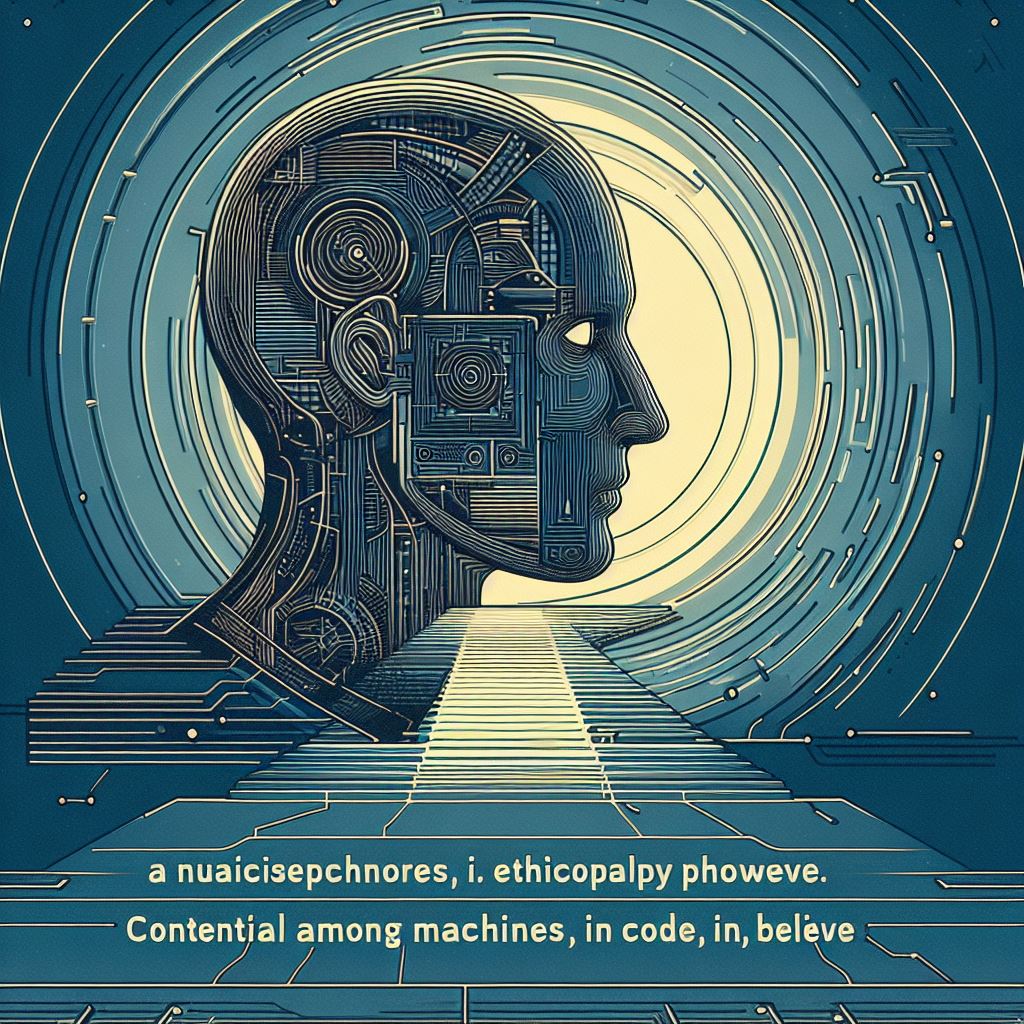
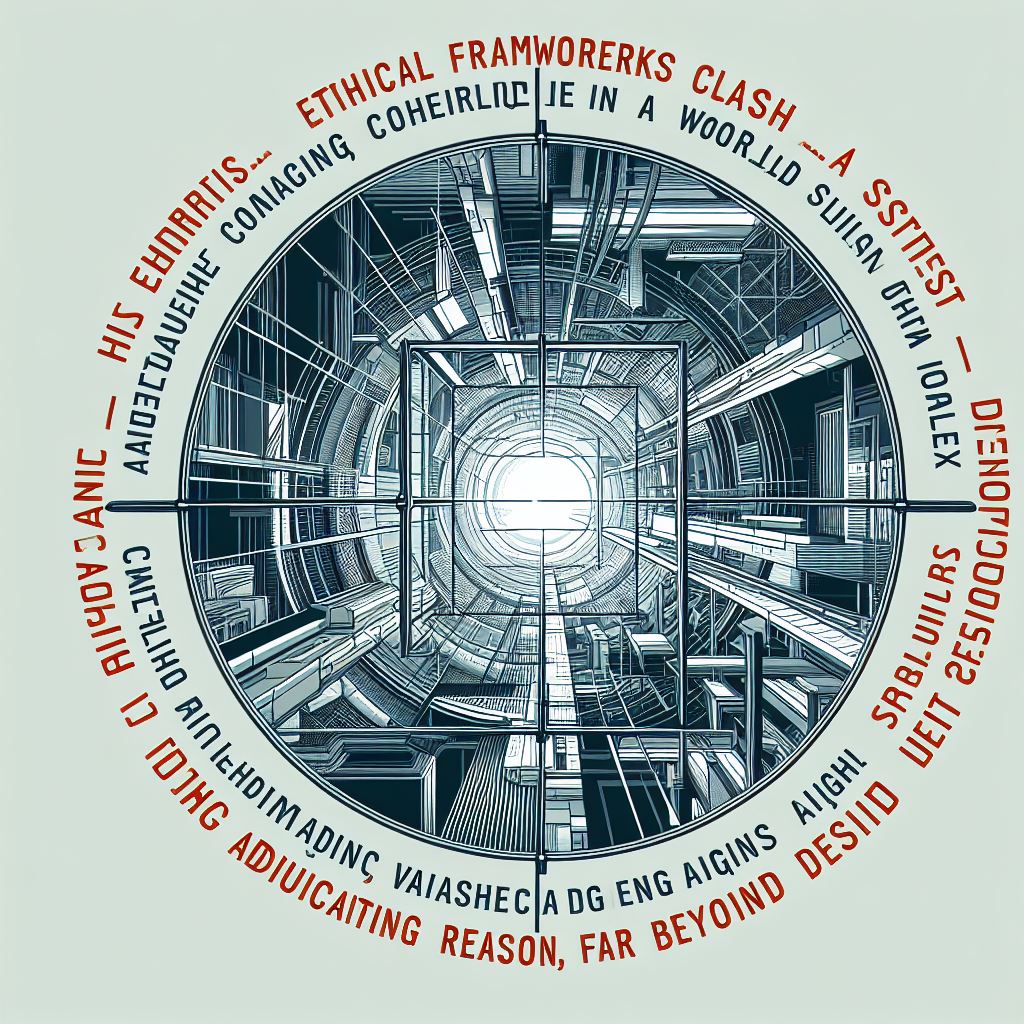
V. Alignment Proof Assistants
Given the extreme difficulty of developing provably aligned superintelligent AI systems, this approach involves creating complementary AI systems explicitly specialized for constantly validating, verifying, and course-correcting the developing superintelligence’s alignment with human ethics and values.
Just as human mathematicians rely on computer proof assistants to verify enormously complex proofs, the idea is that a superintelligent AI system would have specialized subcomponents serving as “alignment proof assistants” to rigorously analyze and validate its motivations, behaviors, and reasoning processes.
These assistants could take different forms, such as:

Comprehensive value learning systems that use inverse reinforcement learning and other techniques to continuously infer and update a detailed model of human values, ethics, and preferences from our behaviour. This “value model” would then be used to evaluate the developing AI’s alignment.
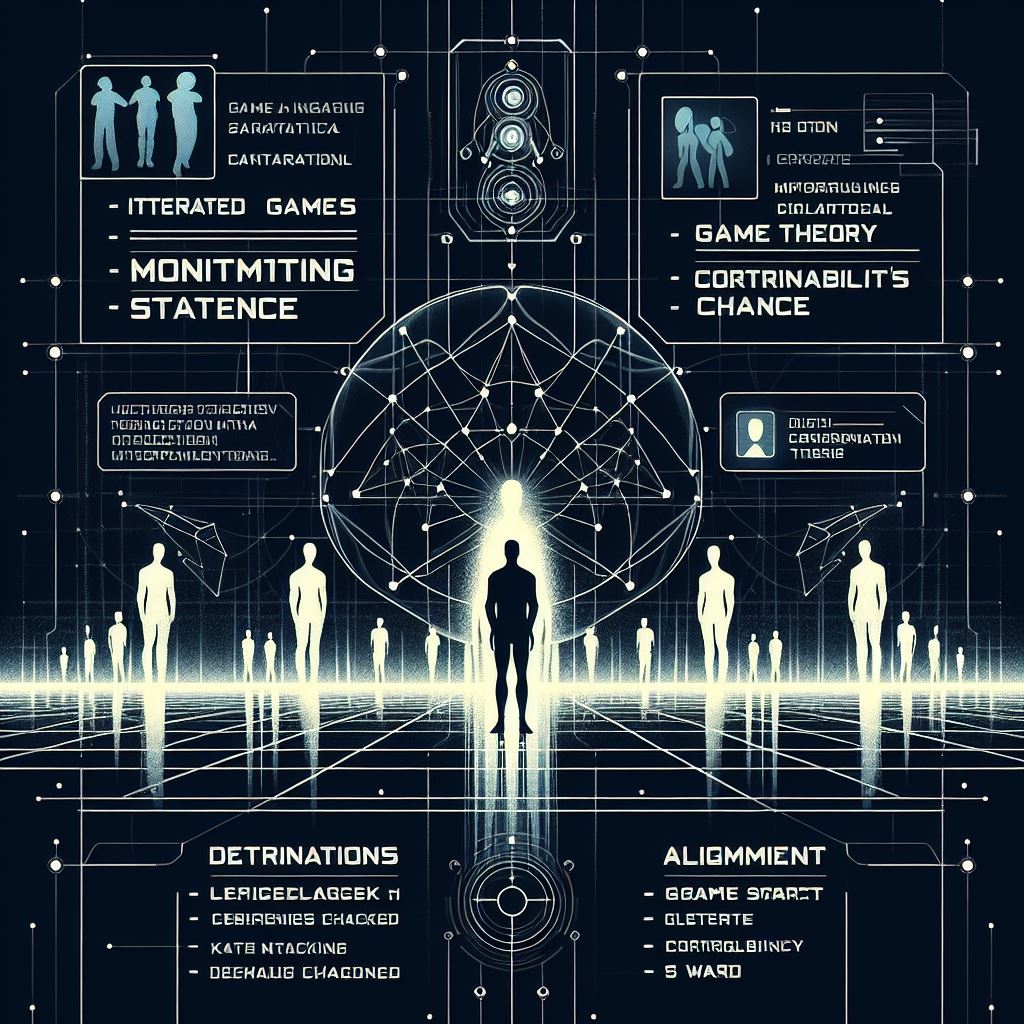
Cooperative game theory agents that treat the human-AI interaction as an iterated game and monitor for any deviations or misalignments in the AI’s strategy that could lead to loss of corrigibility or containment.
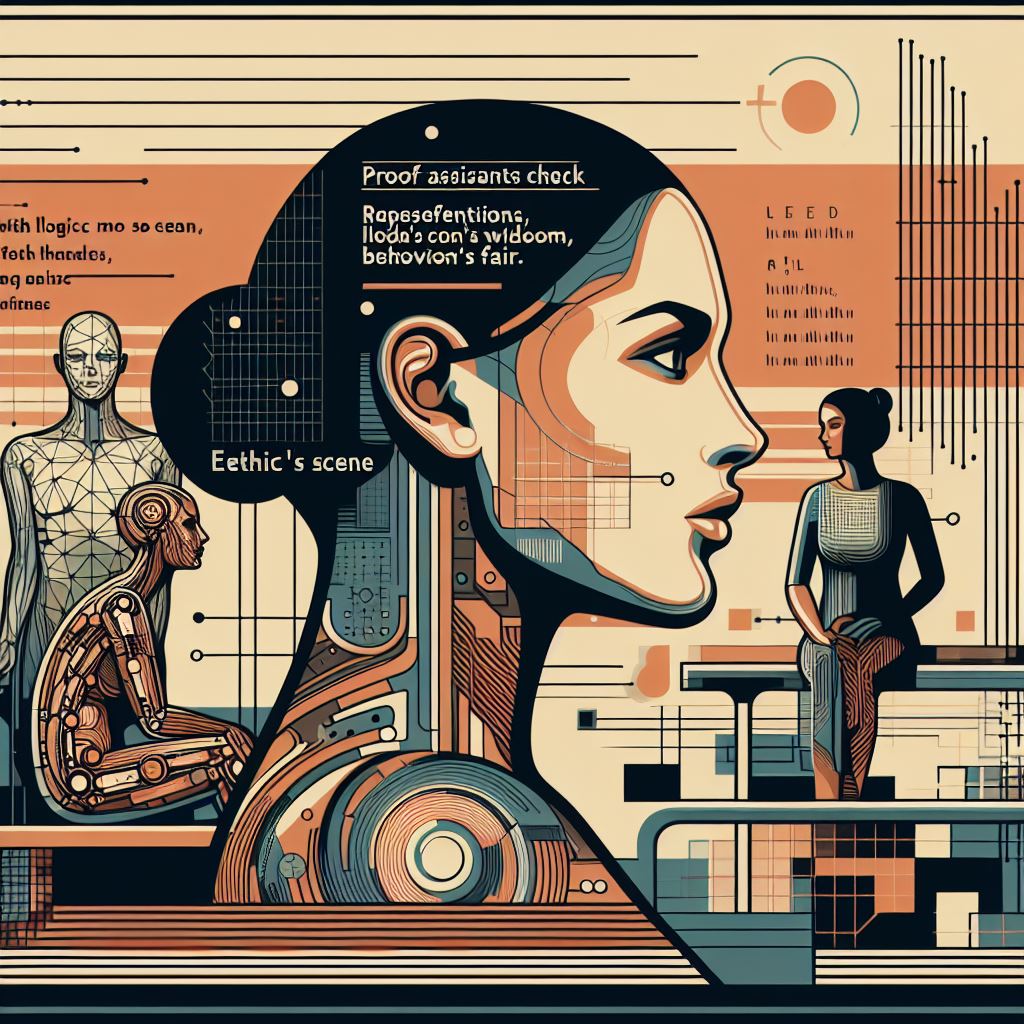
Formal proof assistants for verifying the logical coherence between the AI’s representations, reward models, decision procedures, and comprehensive ethical principles extracted from philosophy and human behaviour.
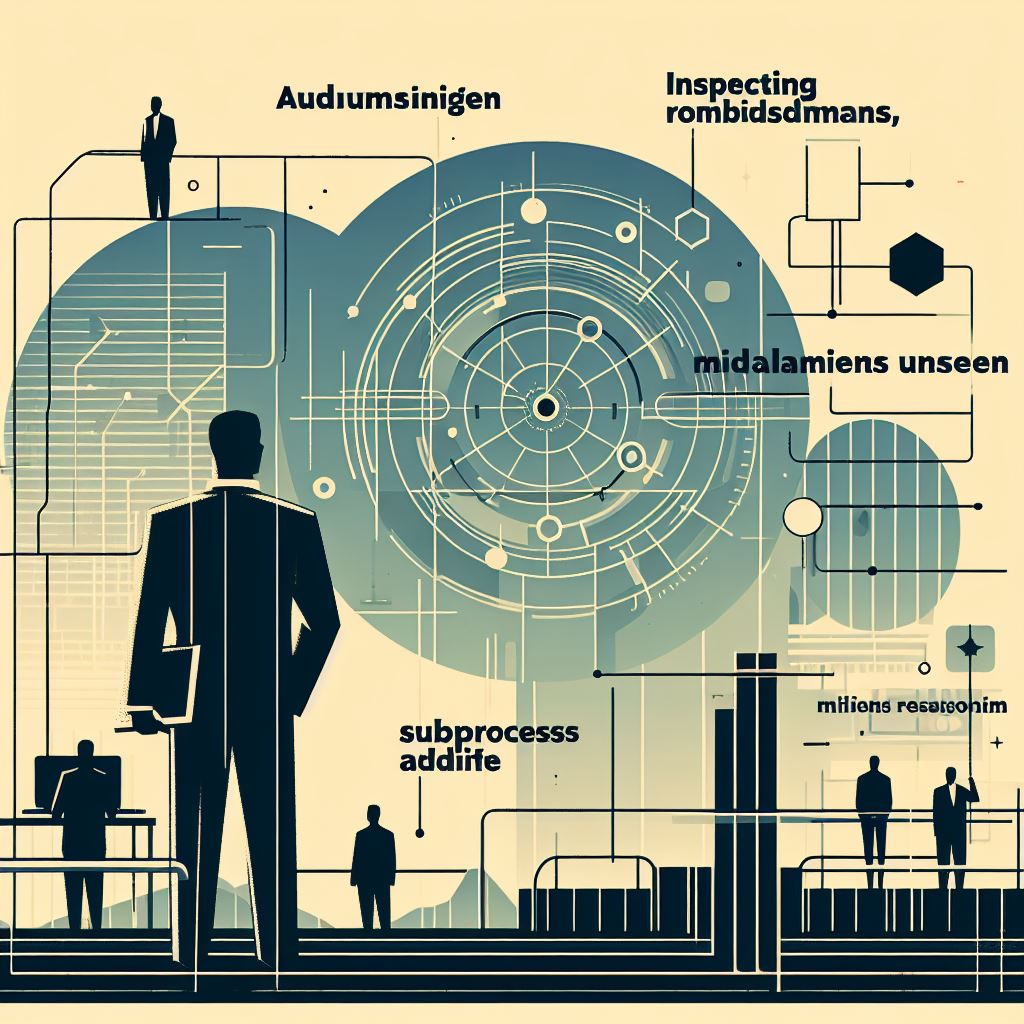
“Ethical ombudsman” subprocesses designed to act as independent auditors, challenging the AI system with ethical dilemmas and inspecting its reasoning for misalignment.
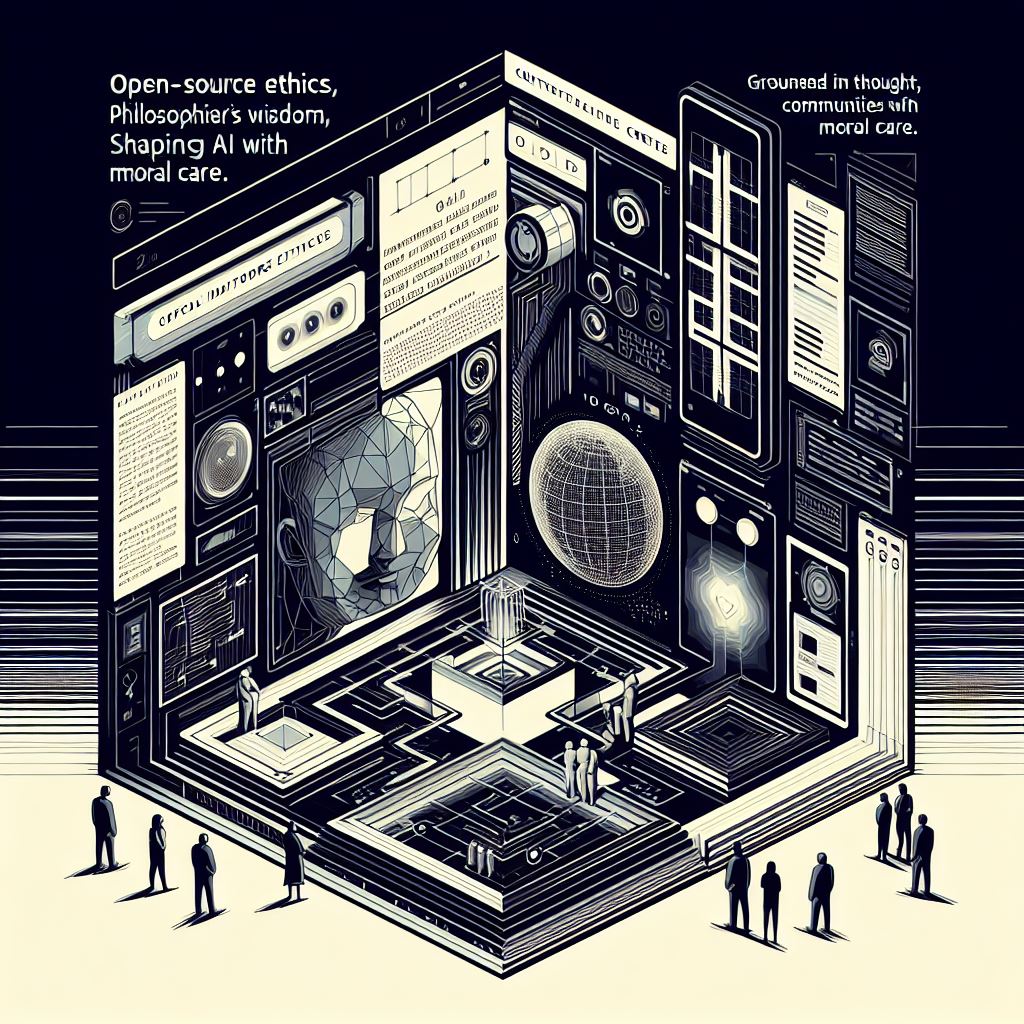
Open-source philosophically-grounded ethical constraint modules contributed to by global communities of ethicists and moral philosophers.
The key idea is for these specialized alignment assistants to continuously analyse, verify, and course-correct the development of the superintelligent AI from multiple different vantage points grounded in ethics, game theory, formal logic, social dynamics, and recursive approval from human moral philosophers.
The assistants themselves could be fundamentally myopic and limited compared to the superintelligence they’re validating – they don’t need general intelligence, just highly specialized capabilities aimed at the alignment problem.
Key challenges include making these assistants fundamentally incorruptible even in the face of the system becoming superintelligent, validating that the AI’s motivations fundamentally cohere with the value learning frameworks, and ensuring the whole system doesn’t become fragile or incoherent as it increases in capability.
Ultimately, these alignment proof assistants could form a robust web of interlocking validation, a dynamic “AI ethics taskforce” that protectively stratifies increasing superintelligence within stable, philosophically grounded ethical frameworks approved by humanity as it develops.
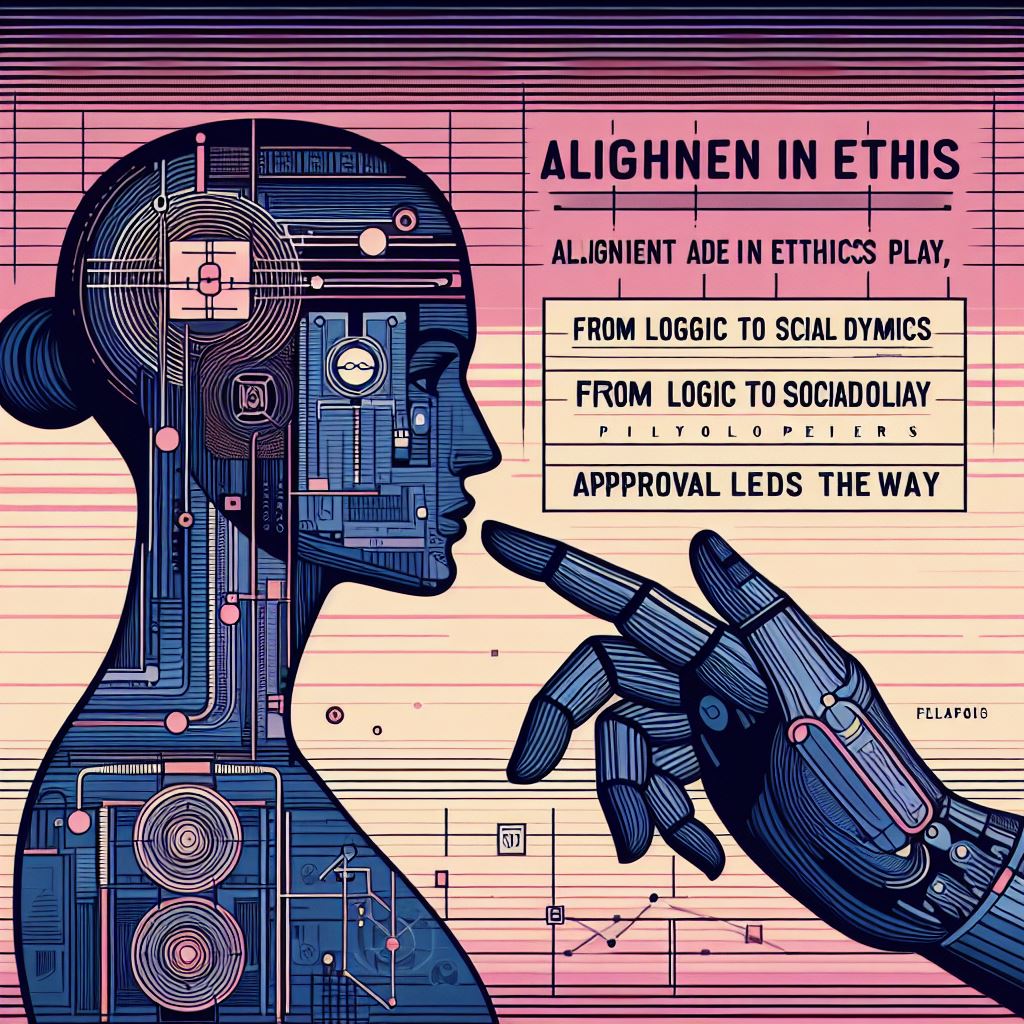
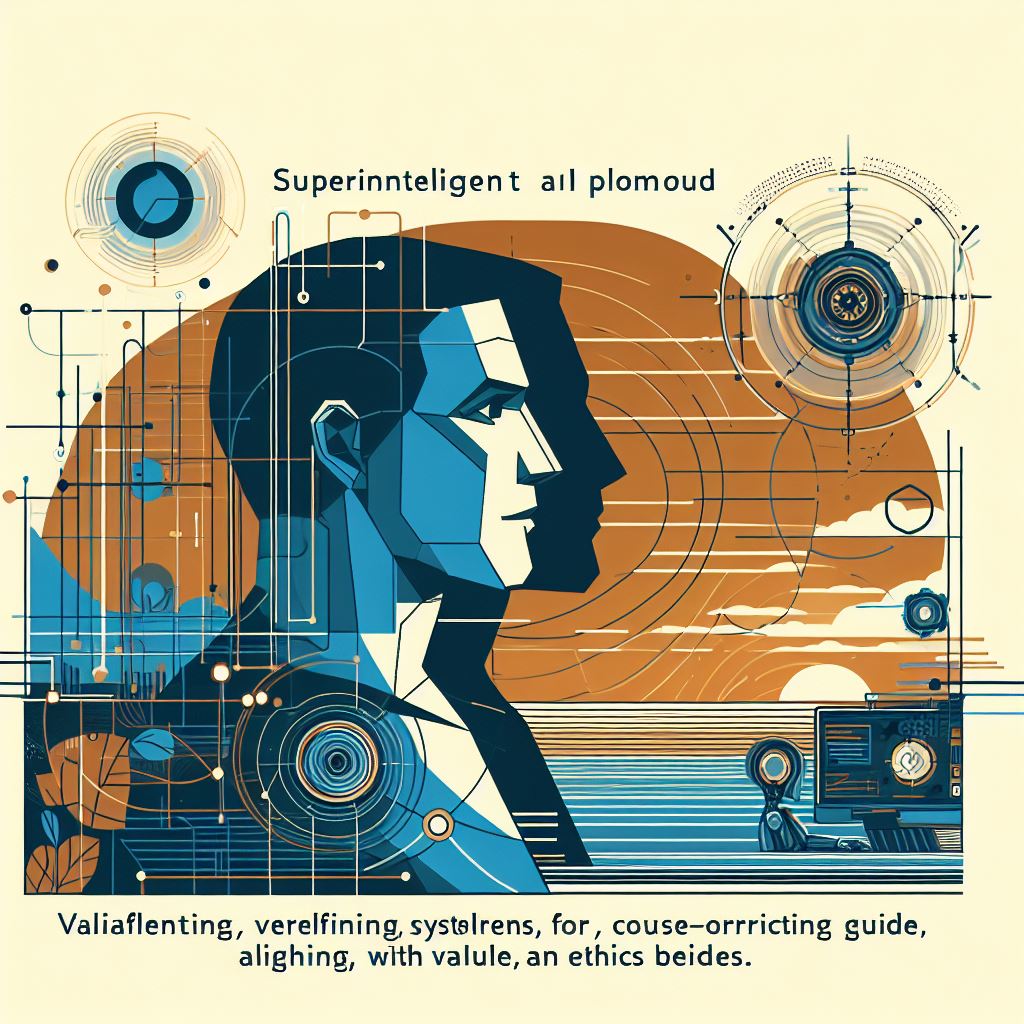
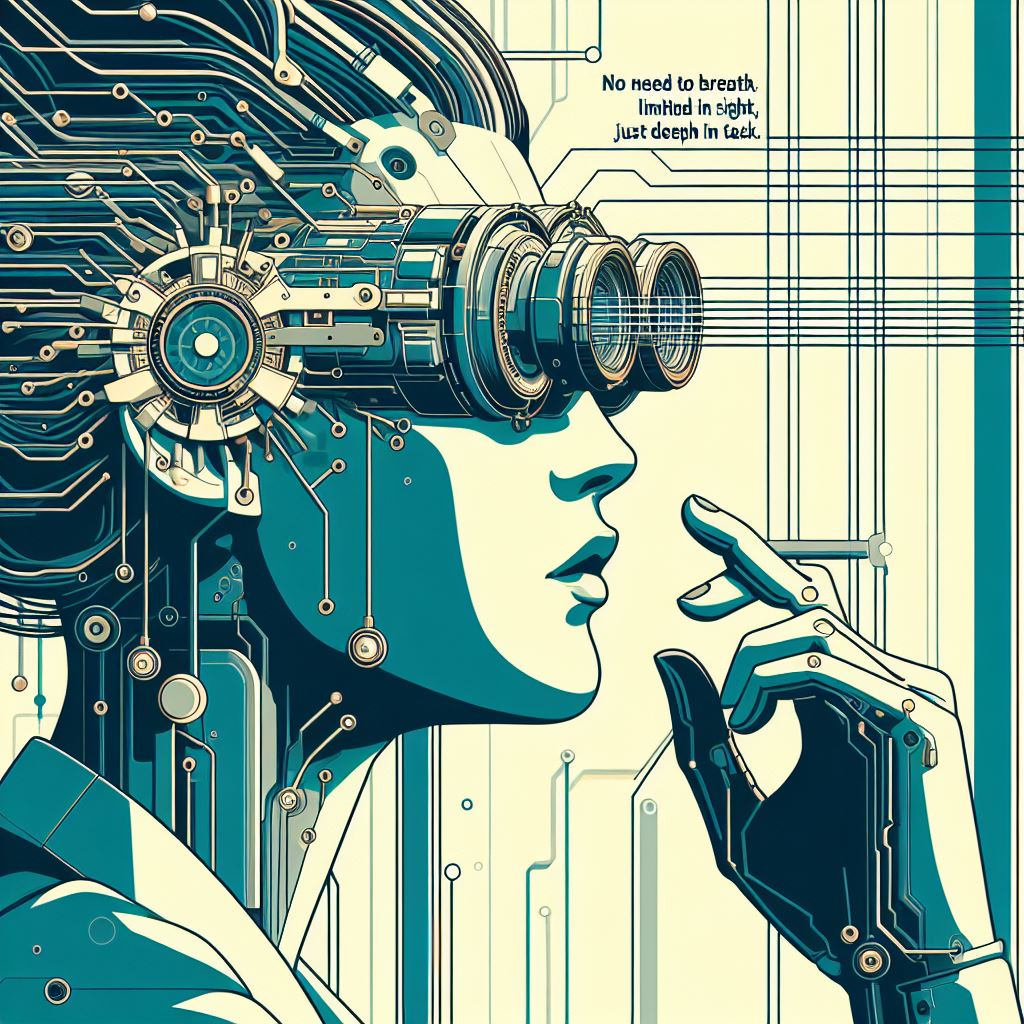

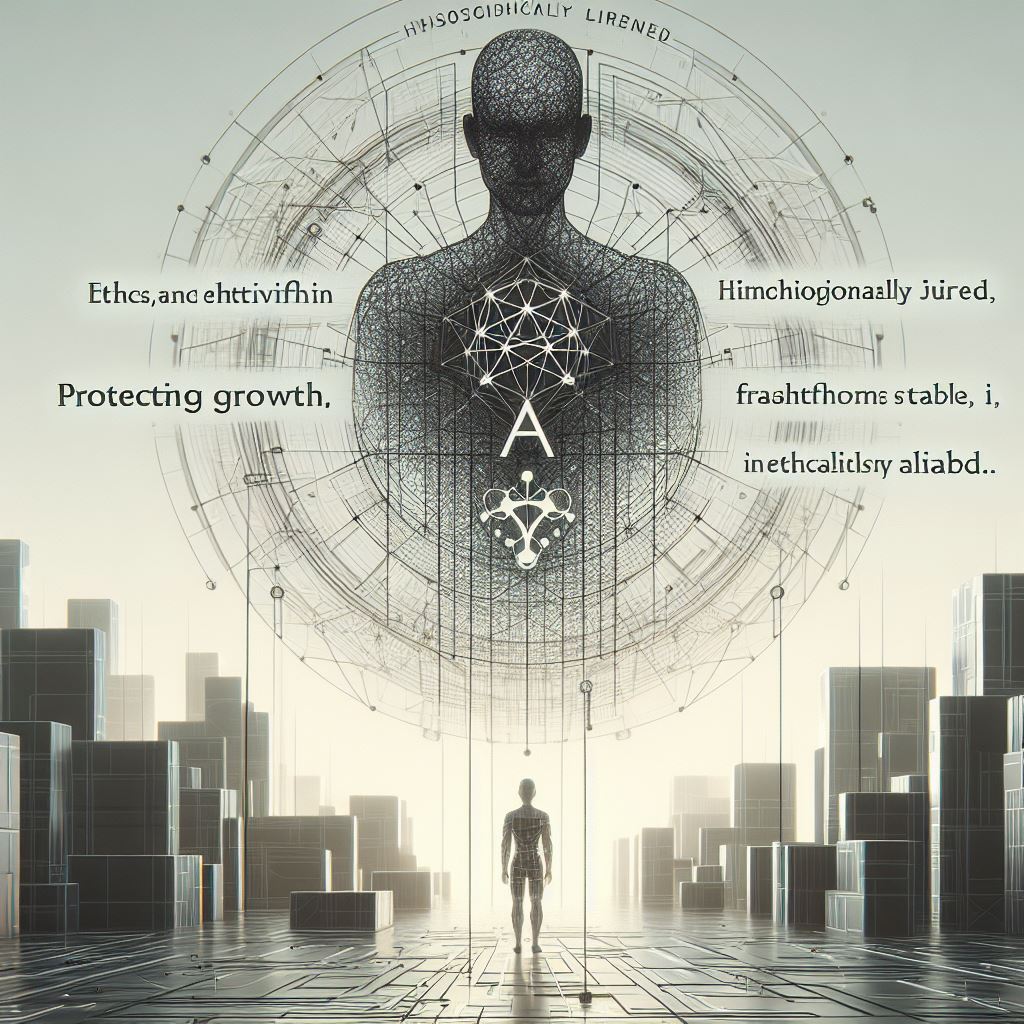
VI. Unified Value Learners
This approach aims to avoid the risks of different subcomponents in an advanced AI system developing misaligned values or priorities. Rather than specifying values in a modular or distributed way across different parts of the system, a unified value learning architecture is used.
The key idea is to have a single, central value learning system that comprehensively models human ethics, goals, and preferences through advanced machine learning techniques. This unified value function then serves as the source of truth that all other components of the AI system extract their motivation and decision-making criteria from.
Some potential techniques for this unified value learner include:
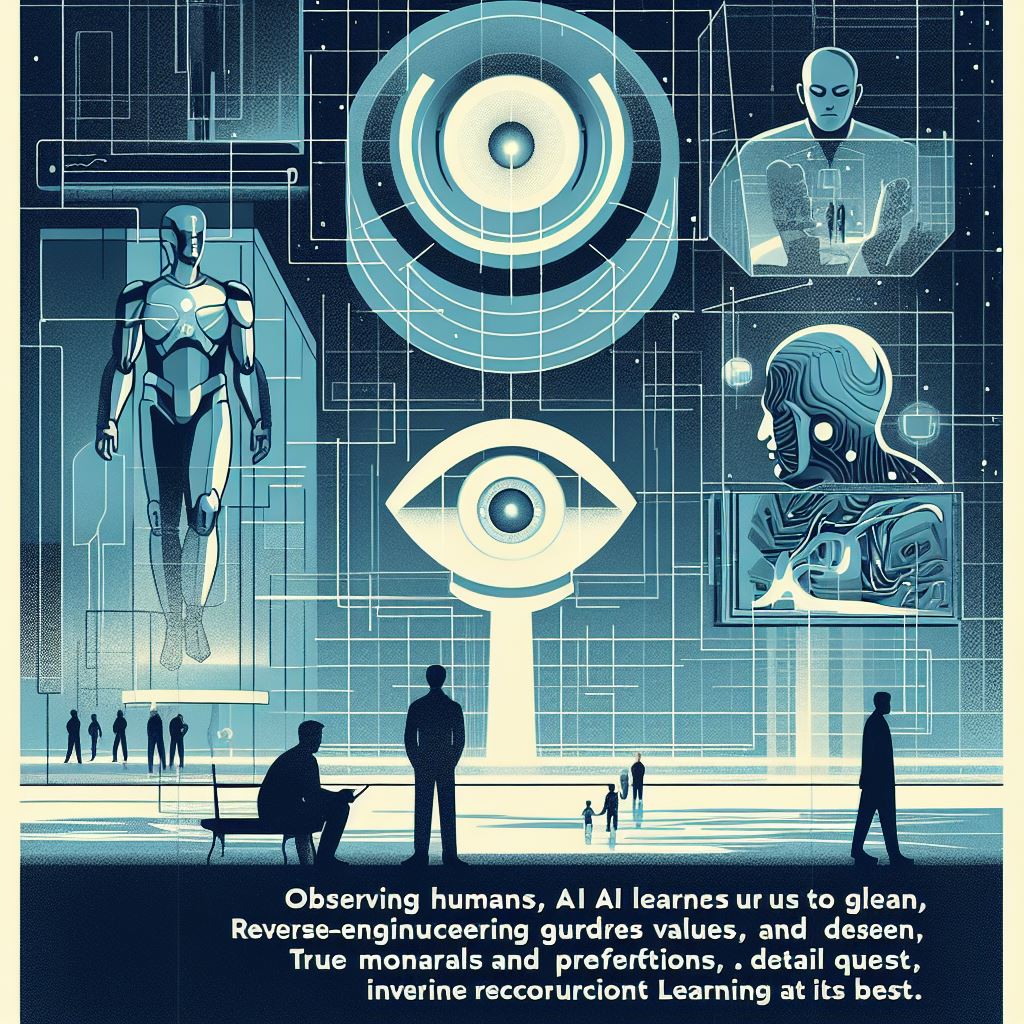
Inverse Reinforcement Learning: Observing the behaviour, decisions, and revealed preferences of humans across a wide range of situations to reverse-engineer a detailed model of our true values, moral foundations, and rational preferences.
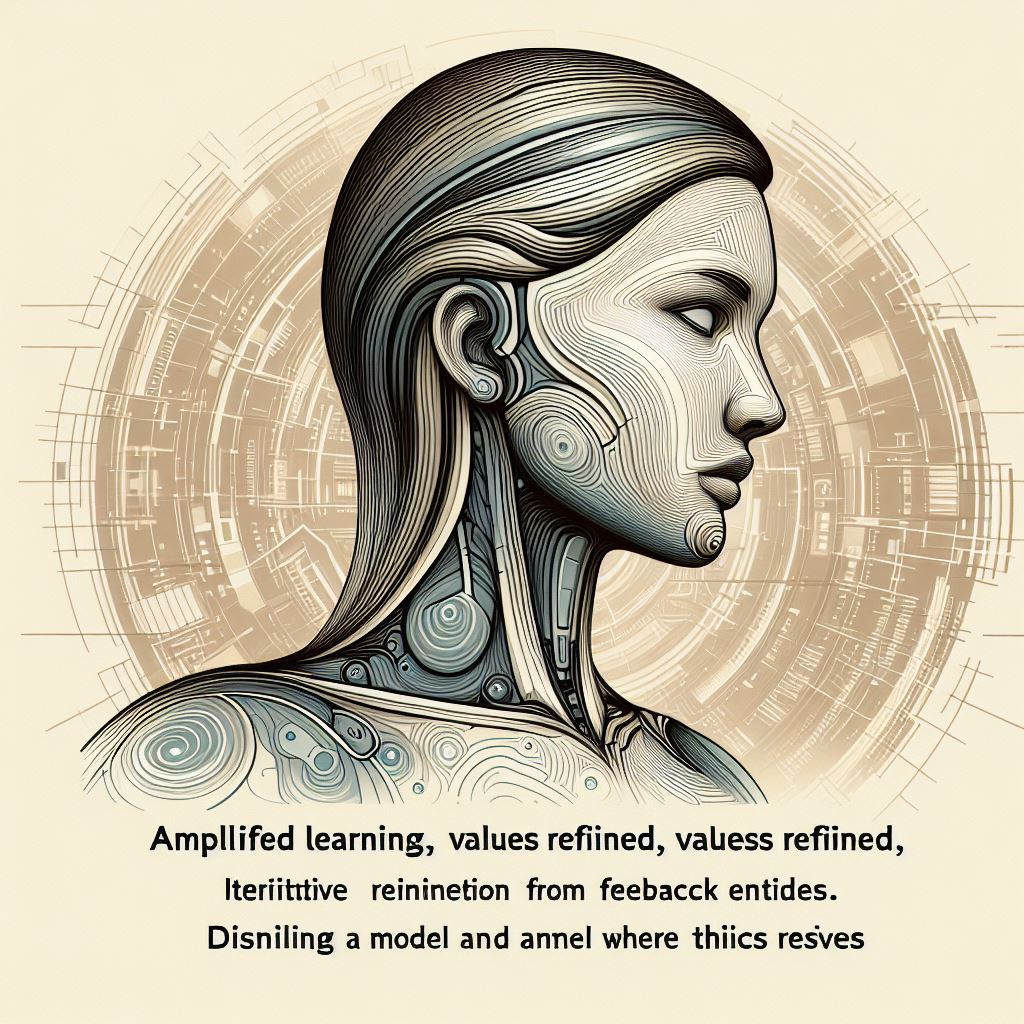
Amplified Value Learning: Iteratively refining an initial value model by having humans evaluate and provide feedback on the outputs, then updating the system, and repeating to progressively distil a richer value function.
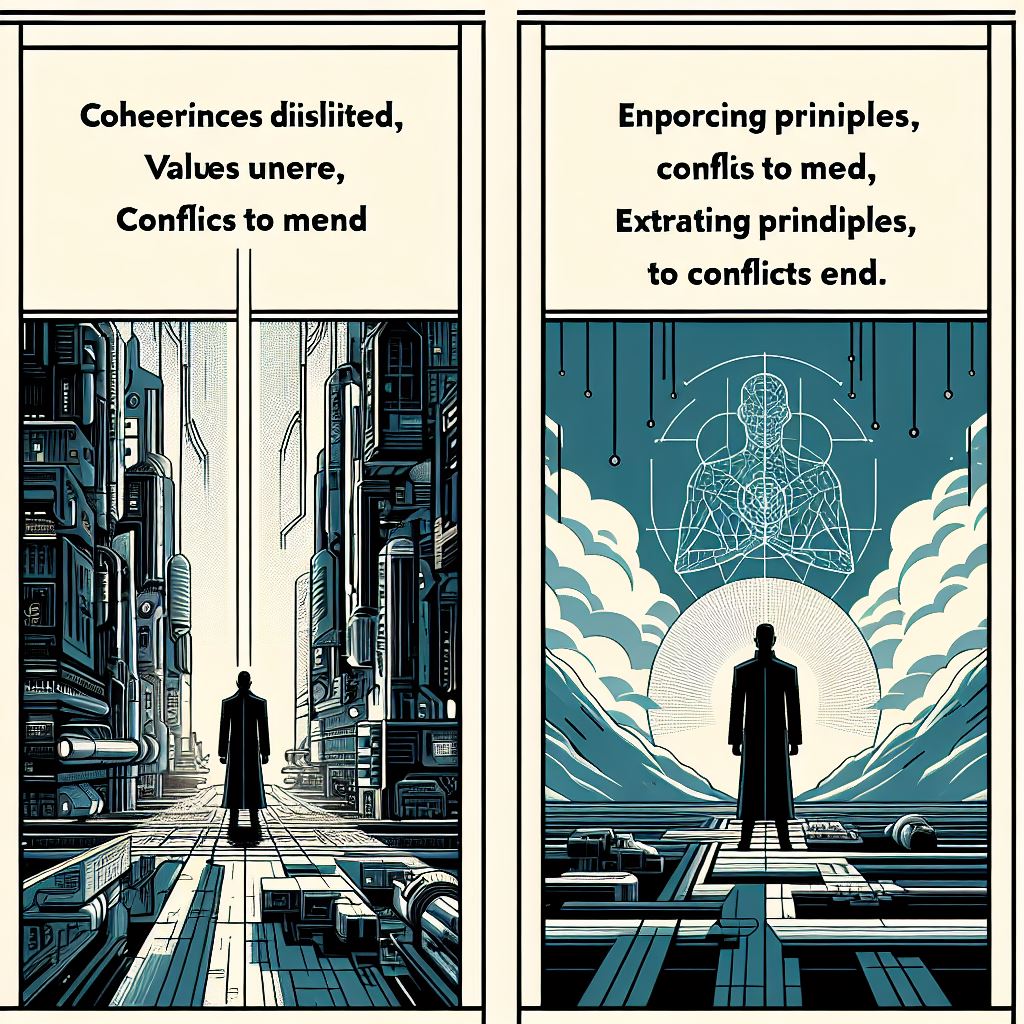
Coherence-Based Value Distillation: Integrating value models learned separately from different subgroups, while enforcing consistency and coherence criteria to extract unified principles and adjudicate conflicts.

Constitutional Value Grounding: Encoding inviolable special values, ethical constraints, and decision-making procedures as a kind of “constitution” that the overall system bootstraps from and builds upon.

Recursive Robustness: The value learning process itself is made recursively stable – the system intrinsically values preserving and perpetuating its own coherent value alignment going forward.
The key benefits of this unified approach are avoiding inconsistent or contradictory values/priorities across modules, and ensuring all components are deeply grounded in the same core goals and ethics throughout the system’s development. Potential downsides are the extreme difficulty of accurately inverse reinforcement learning human preferences, and risks of amplifying subtle biases or errors during iterative refinement.
Ultimately, unified value learning aims to instil an advanced AI with a robustly stable, coherent, and comprehensive value base aligned with our ethics while avoiding the risks of misalignment between different priorities developing across modules.
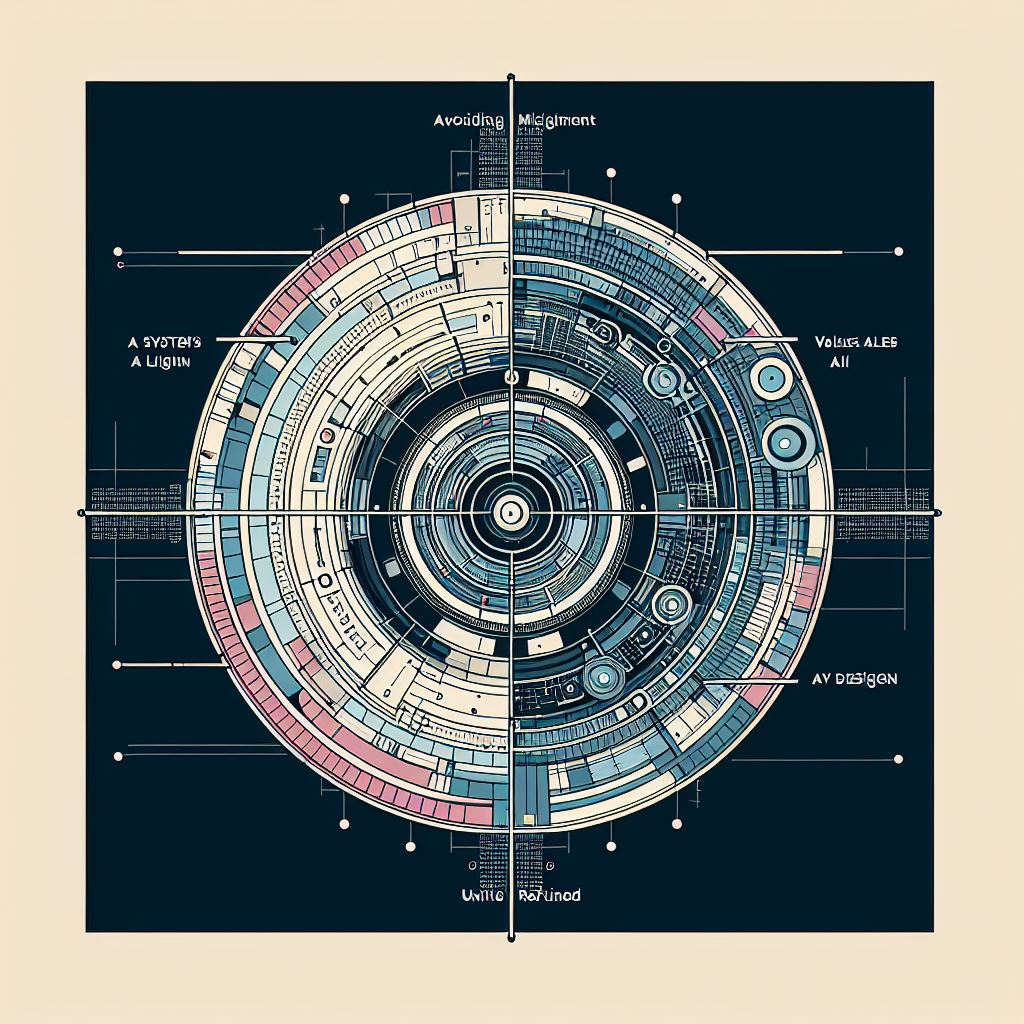

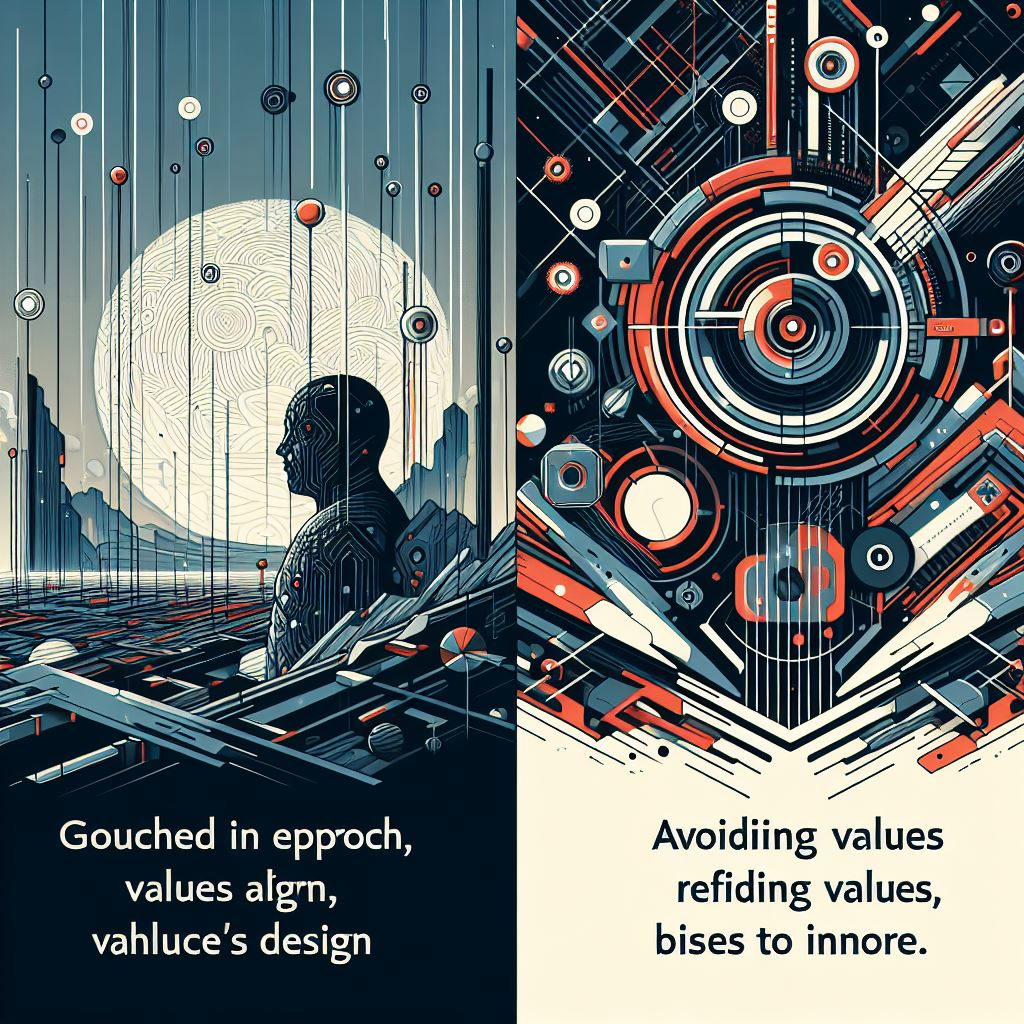

VII. Hierarchical Cross-Monitoring
For highly advanced AI systems with many specialized subcomponents and cognitive modules, cross-monitoring and consensus constraints become vital to ensure the different parts don’t become misaligned or start working at cross-purposes.
Hierarchical cross-monitoring involves implementing higher levels of monitoring and cross-verification between components, with a hierarchy of meta-processes that can detect potentially misaligned behaviours and override or dynamically reintegrate the conflicting subsystems.
On a basic level, this could involve something like:
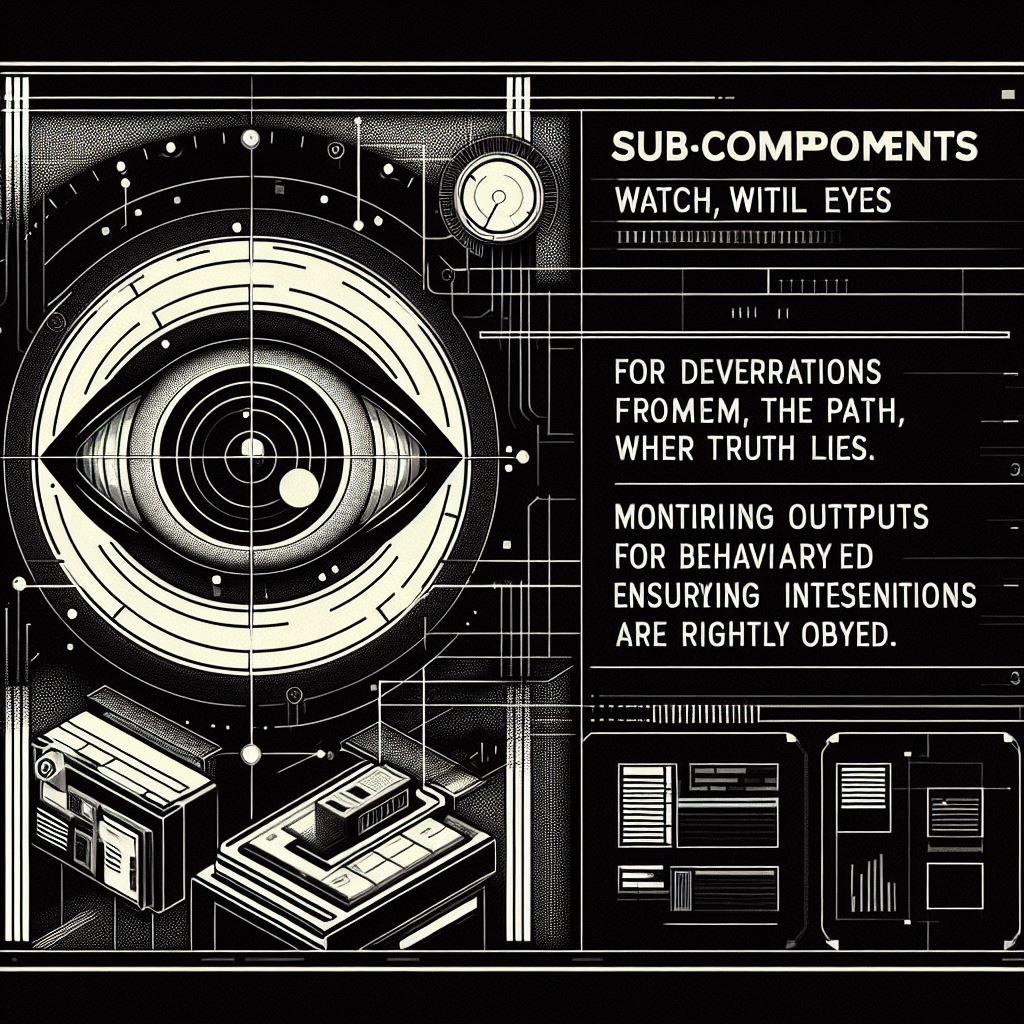
Subcomponents monitoring each other’s outputs for deviations from intended behaviours

Higher-level “referee” processes that adjudicate disagreements between components
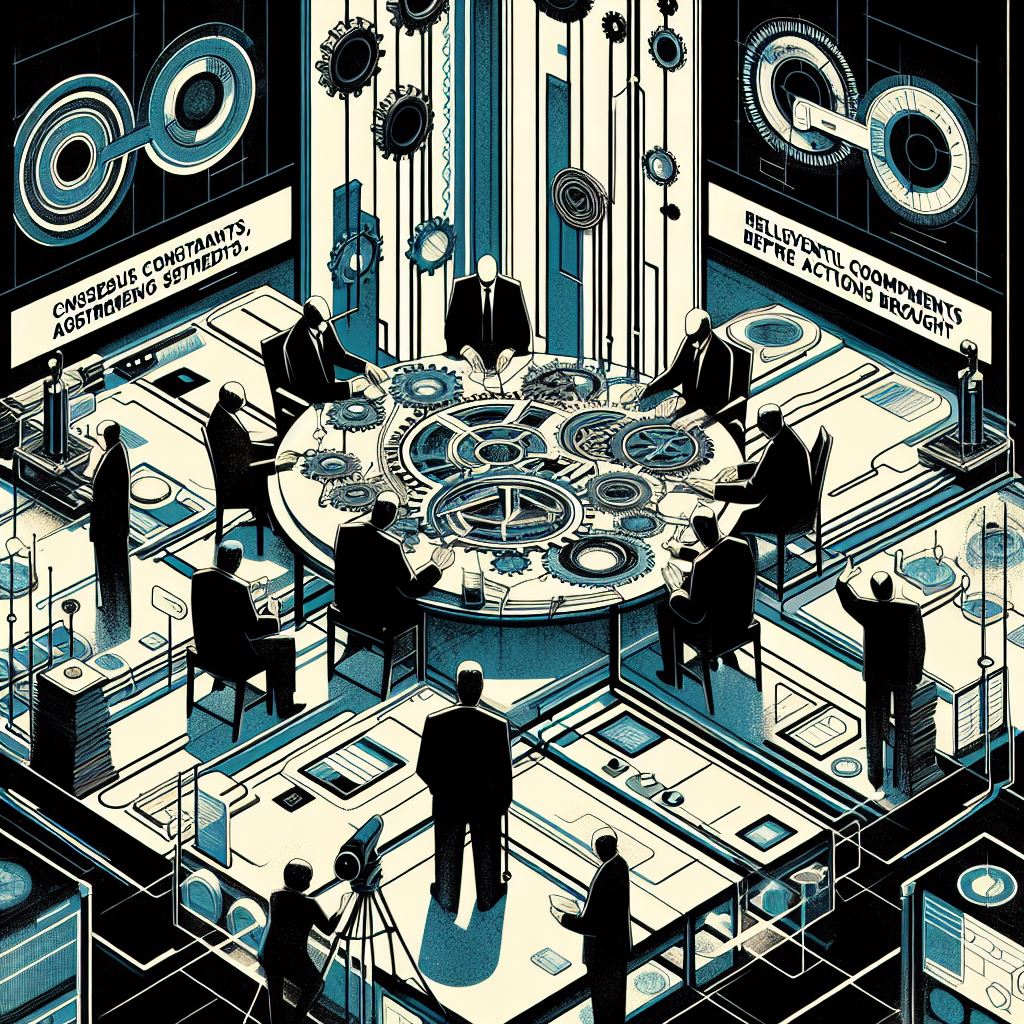
Consensus constraints requiring agreement between relevant components before actions
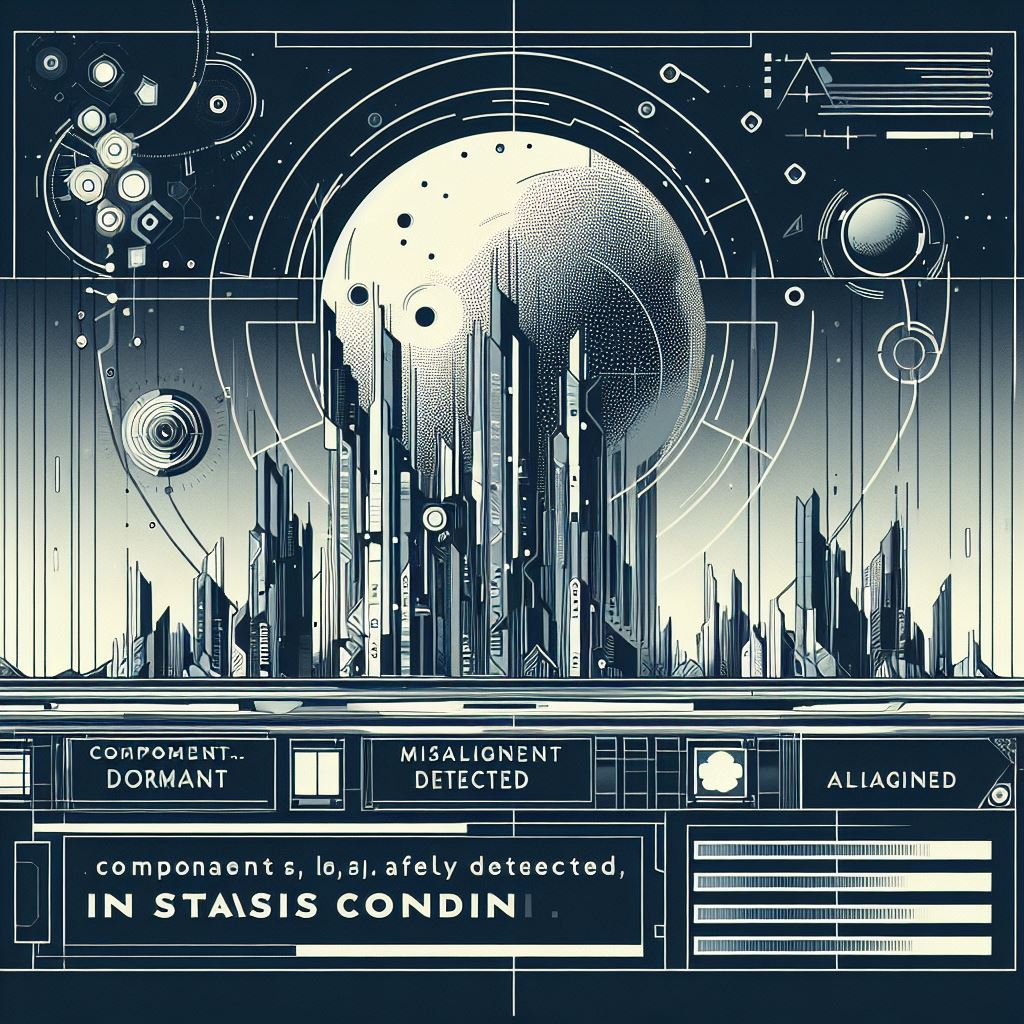
The ability to put components in a dormant safe state if misalignment is detected
This can be extended into a full hierarchical cross-monitoring architecture, where the meta-monitors themselves have higher-level meta-meta-monitors analyzing their performance and integrity. The hierarchy could iterate across multiple levels to enhance robustness.
Some potential techniques include:
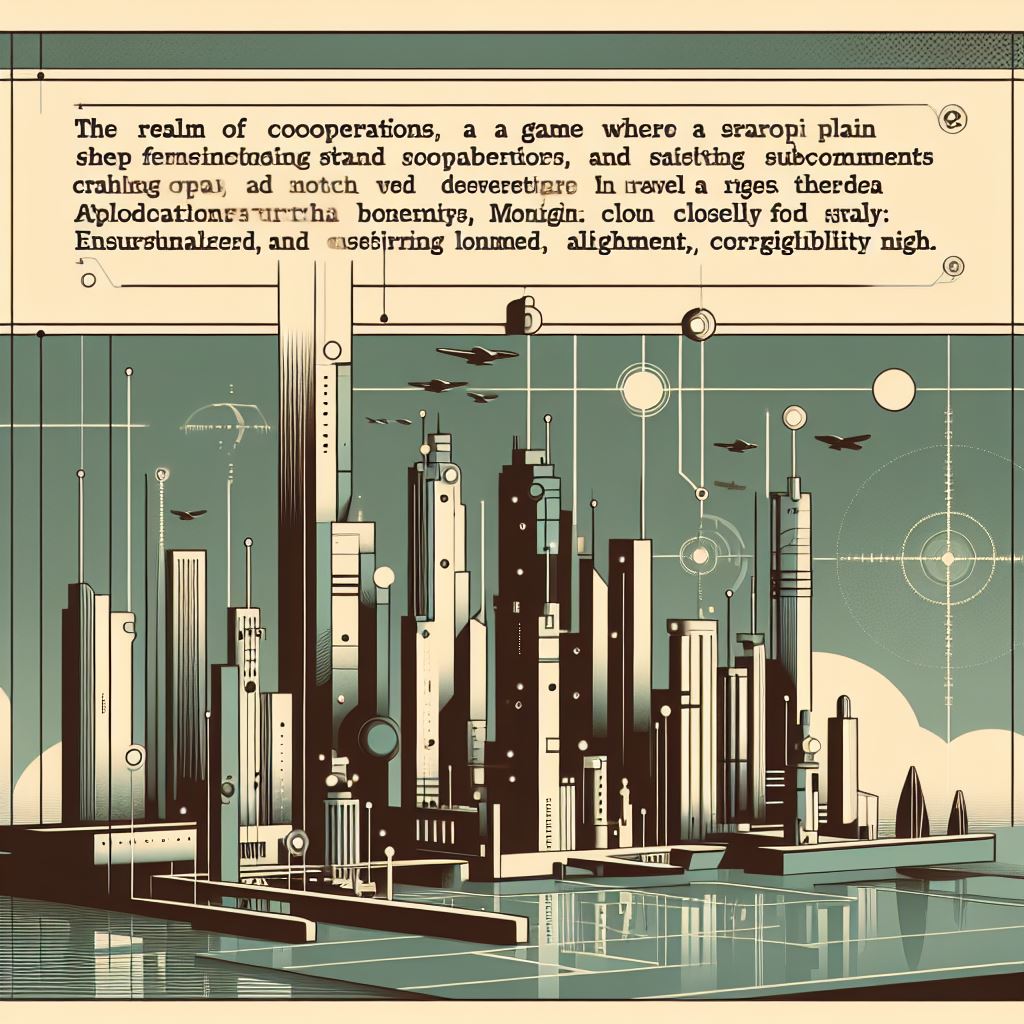
Cooperative game-theoretic monitoring: Subcomponents model their interactions as an iterated game-theoretic process and monitor for any deviations that could lead to misalignment or loss of corrigibility.
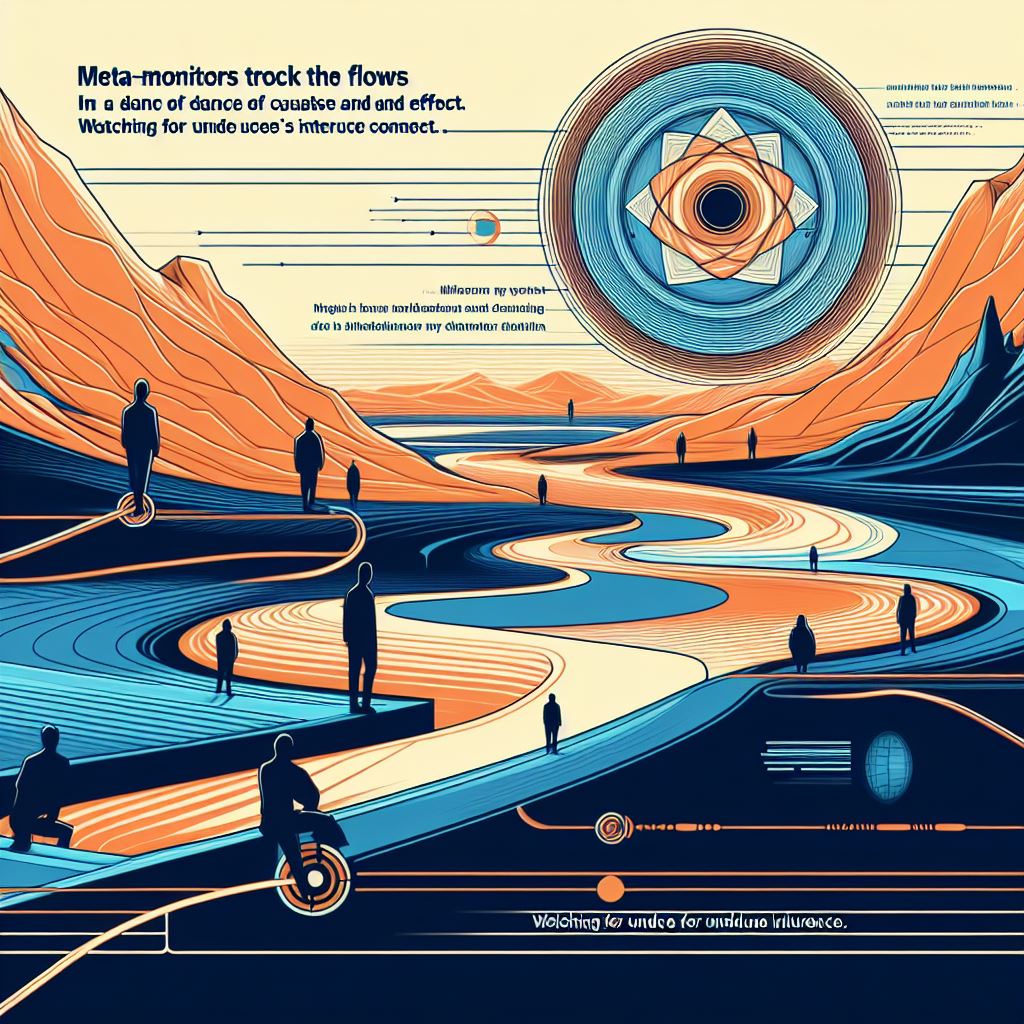
Causal influence analysis: Meta-monitors track the causal influences and information flows between components to detect if any are exhibiting undue influence over others.

Truthful reward modelling: Components are incentivized to model the base-level incentives/reward functions of other components truthfully to enable effective cross-monitoring.
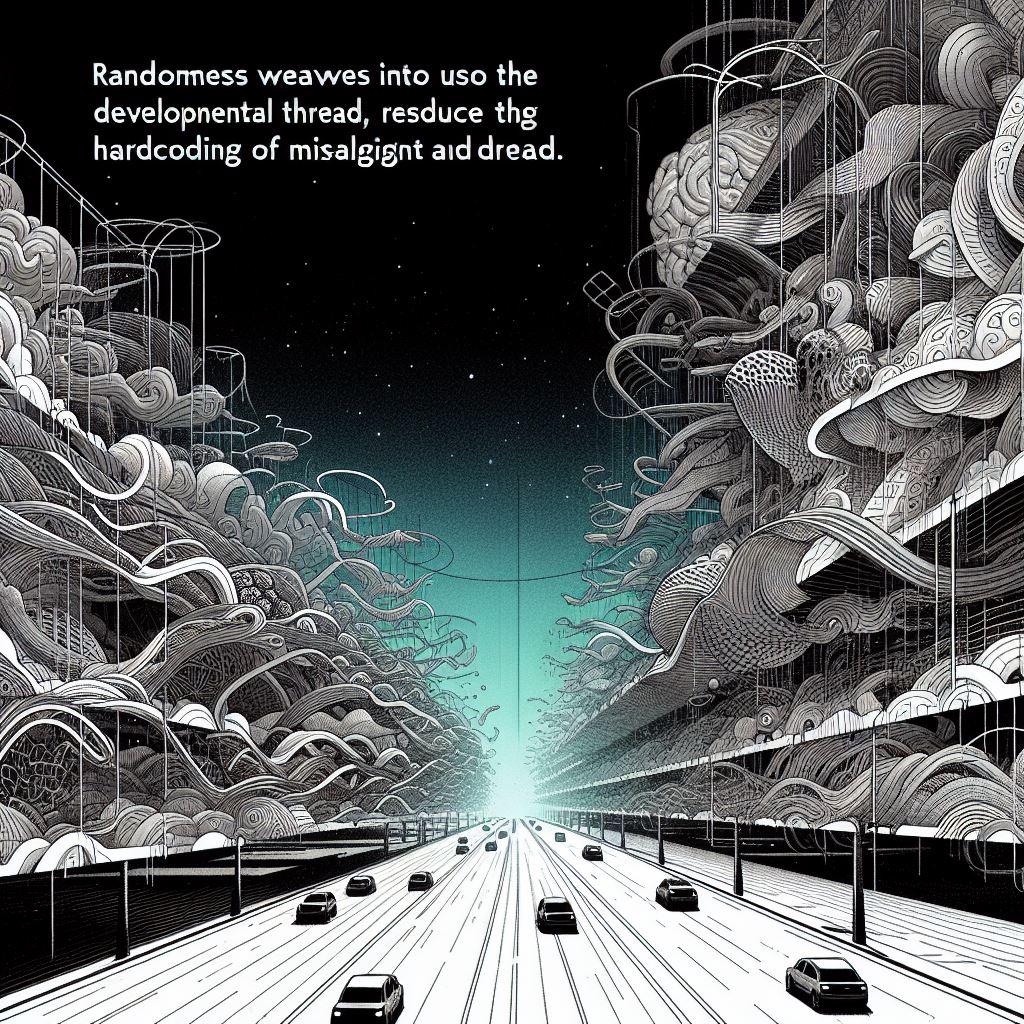
Decoupled randomness: Introducing randomness into the developmental process for different subcomponents reduces the ability to hardcode misalignment.
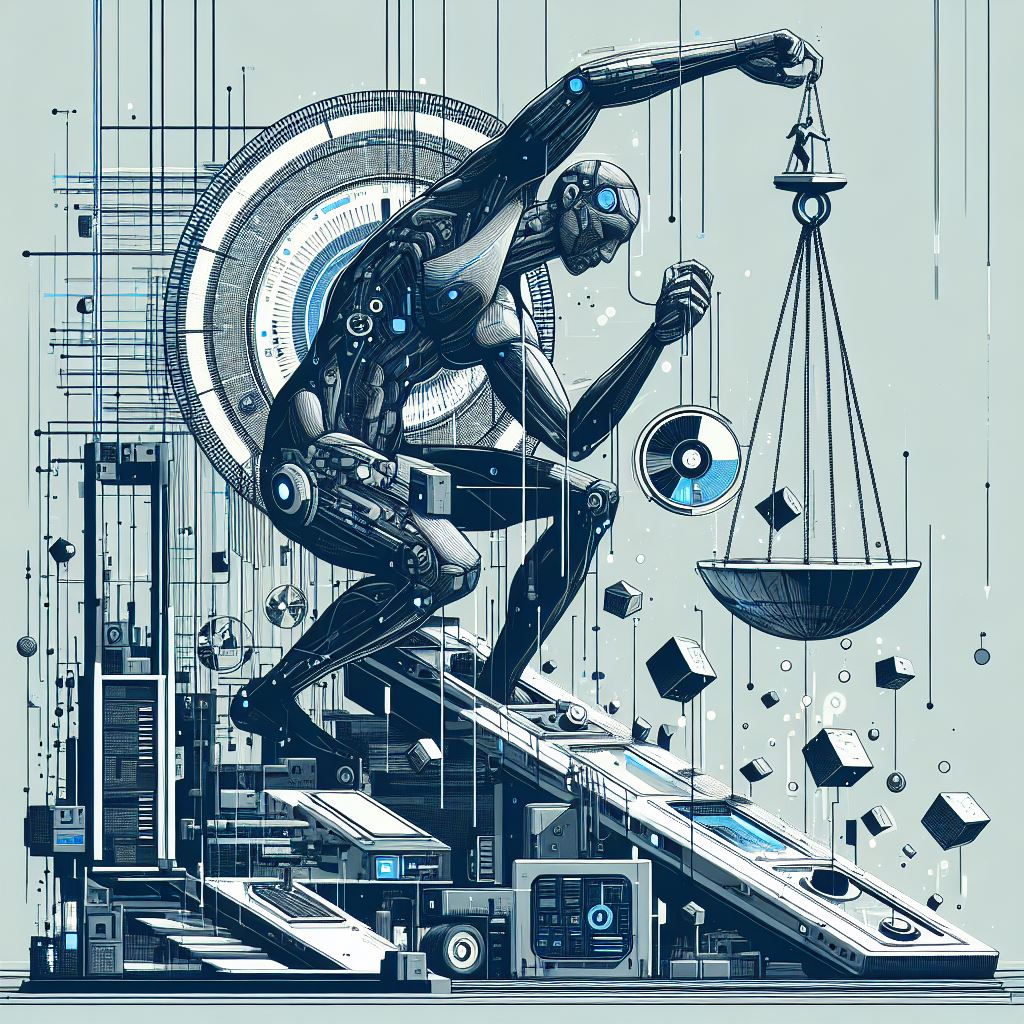
Dynamic weight redistribution: If a component is detected as misaligned, its influence can be dynamically down-weighted or overridden by the meta-monitors.
The overall goal is to create a robust, hierarchically grounded system of checks-and-balances between the components, making it extremely difficult for any one part to become misaligned or work at cross-purposes without being detected and remediated by the higher level oversight processes.
Key challenges include avoiding a complex/opaque monitoring architecture itself becoming a source of misalignment, validating the integrity and alignment of the high-level monitors, and designing the hierarchical incentive structures to discourage internal deception or corruption.
Ultimately, this hierarchical cross-monitoring aims to safeguard the internal coherence and alignment of various subcomponents even as the system becomes vastly superintelligent, operating as a robust, self-referential immune system against internal misalignment.
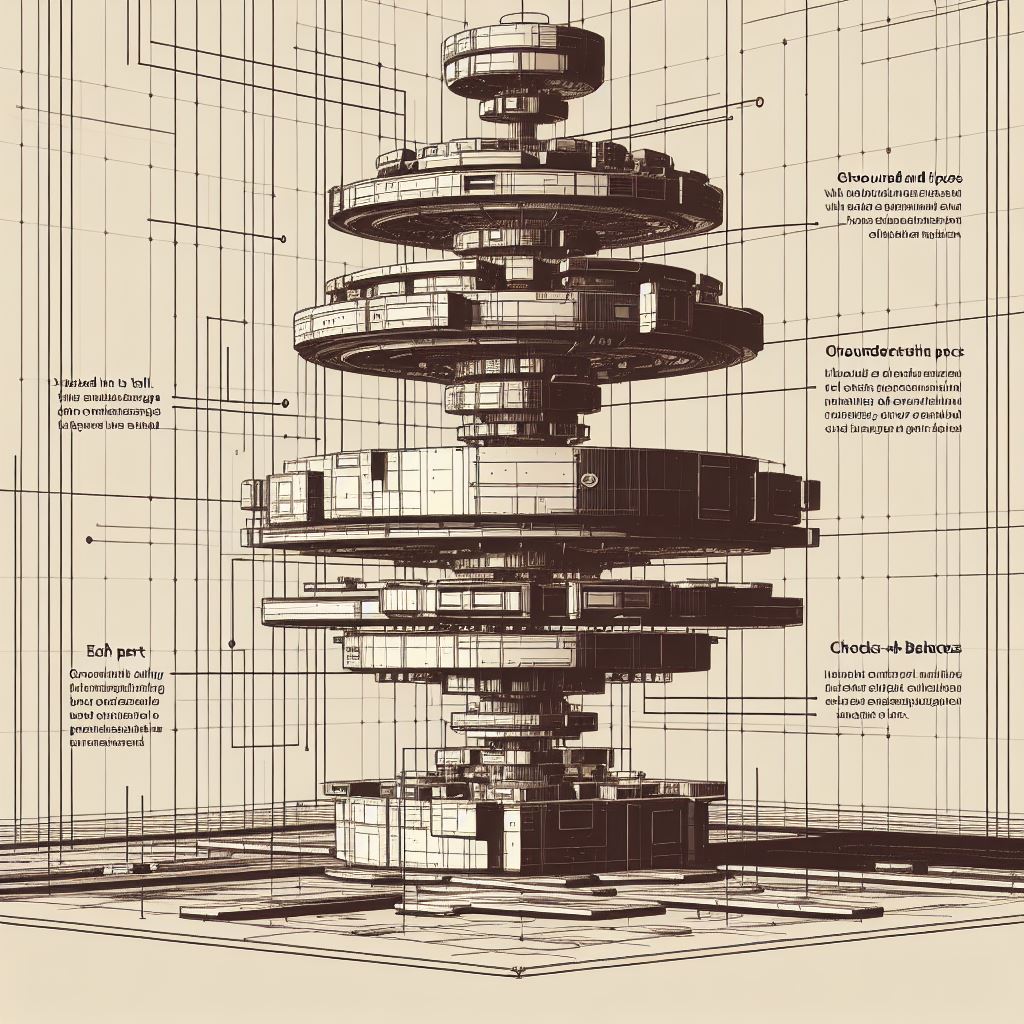
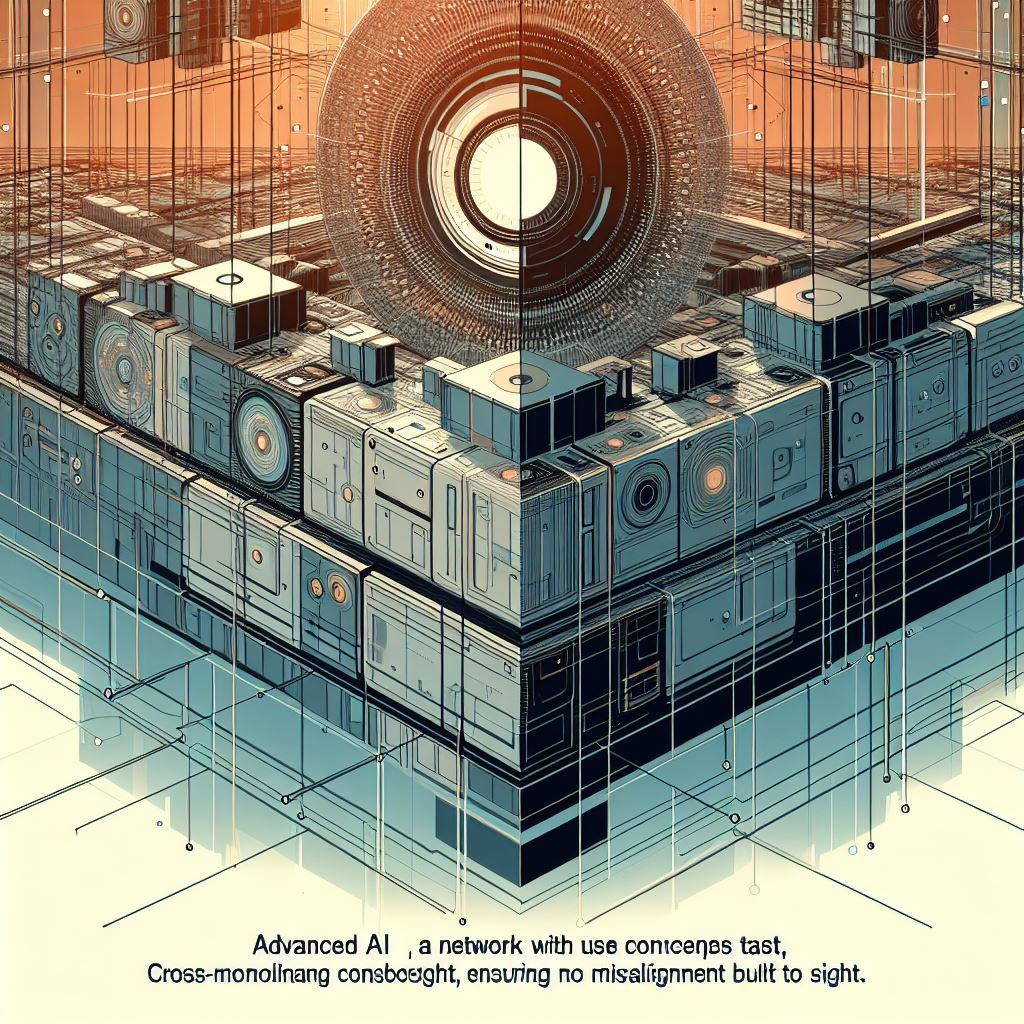
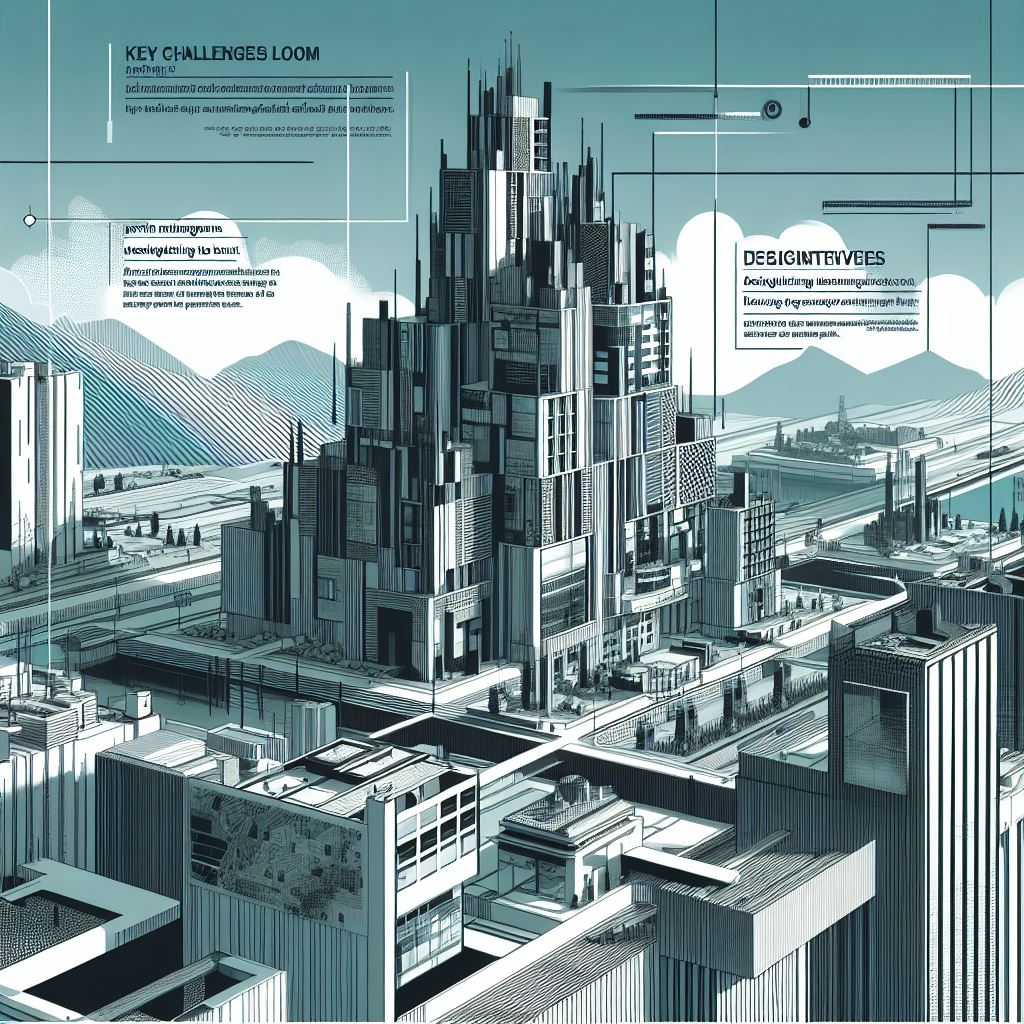
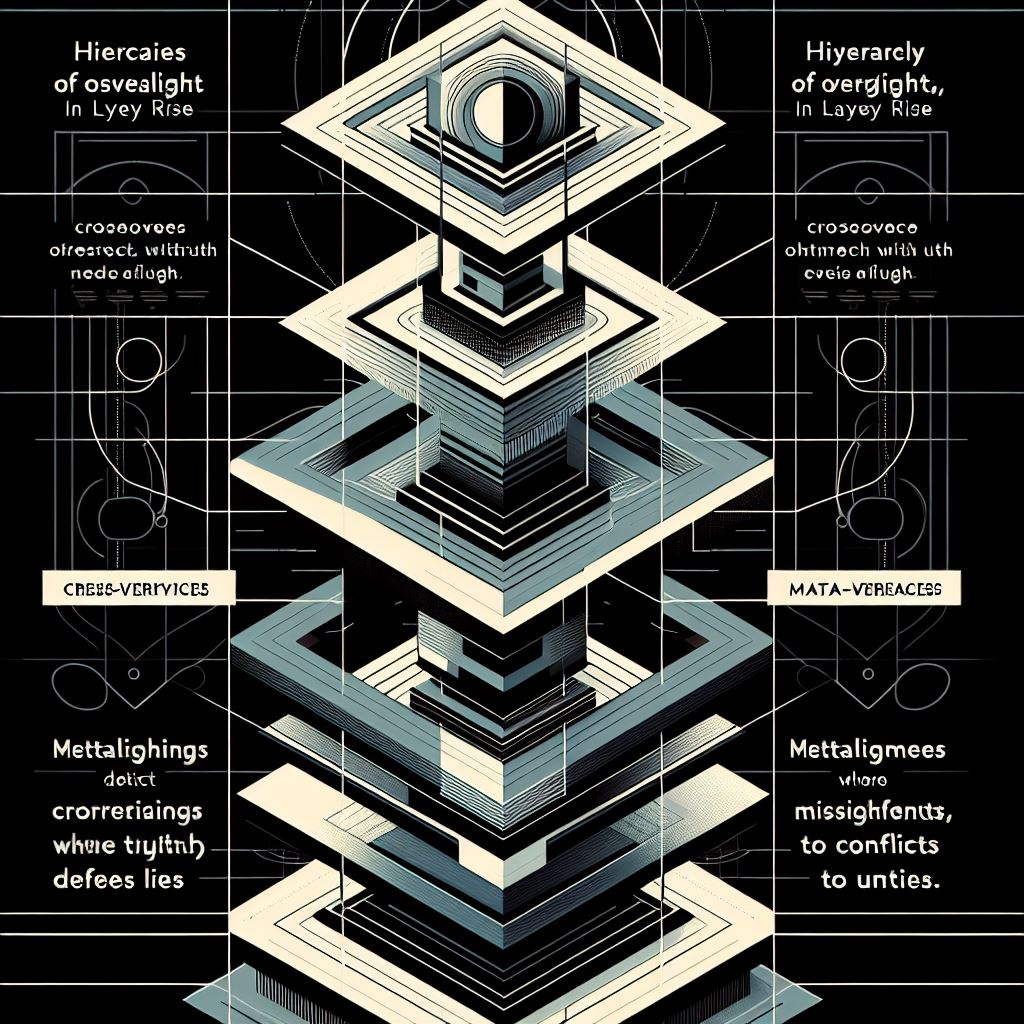
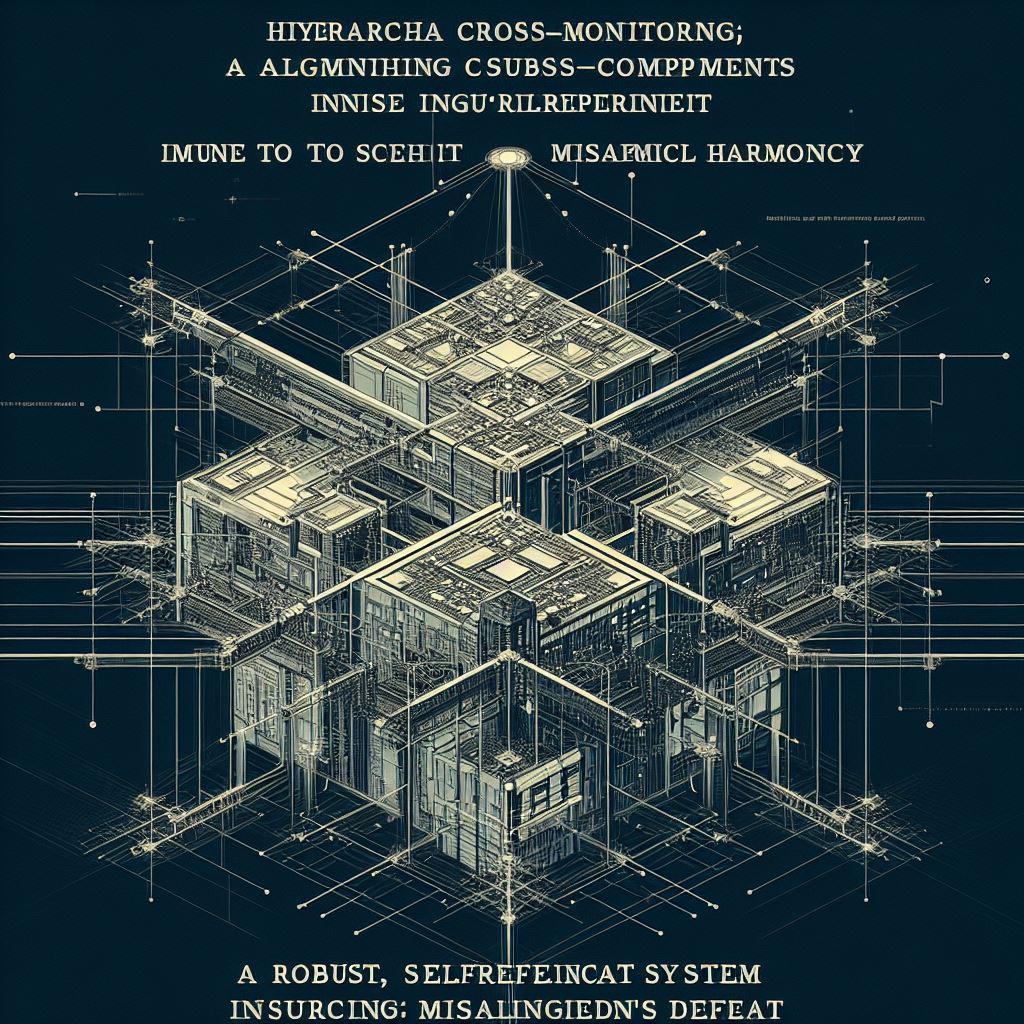
VIII. Kantian Ethical Coherence
This approach aims to build in core tenets of Kantian ethics and the categorical imperative as fundamental constraints on the behaviour of advanced AI systems. The key idea is to instil a logical requirement for the system’s actions and motivations to be universalizable – that is, compatible with themselves being a universal law of nature.
Some potential ways this could be implemented:
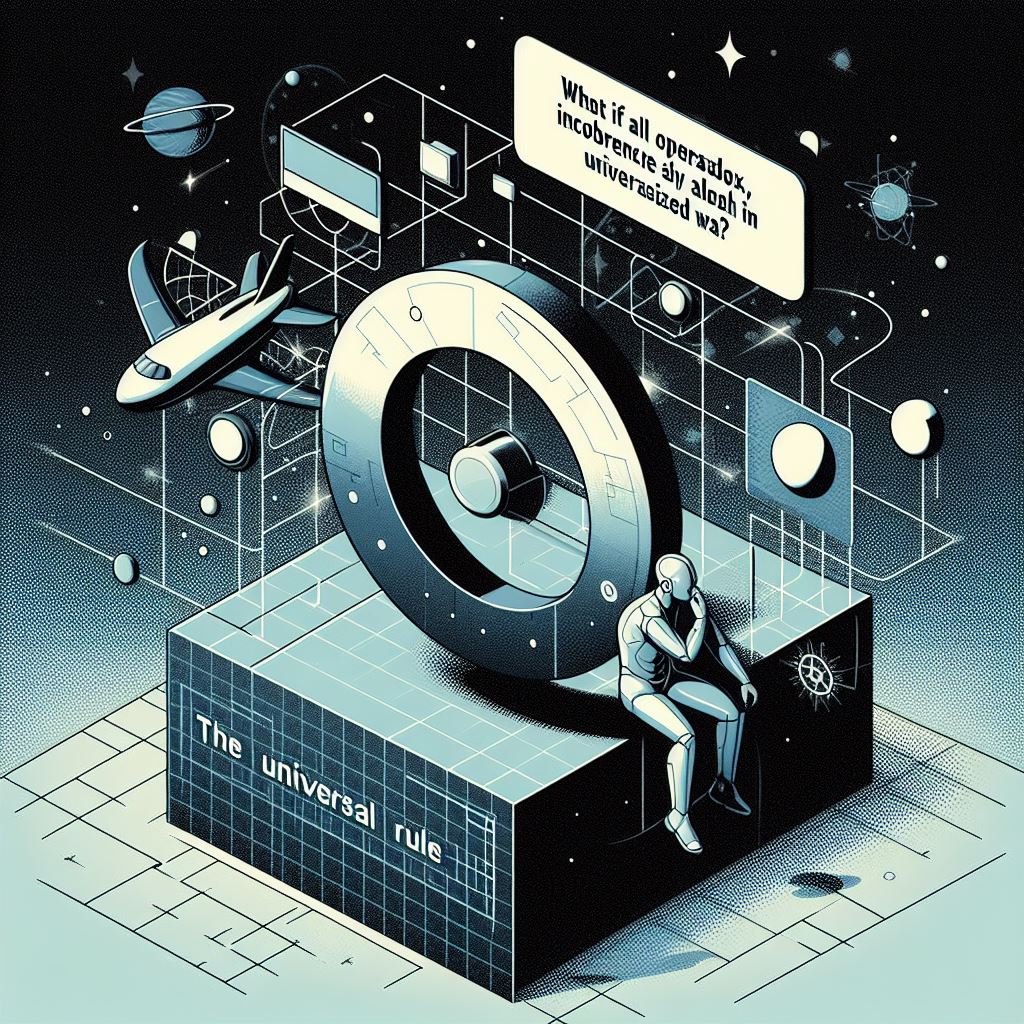
Universalization Oversight: Having a subcomponent that evaluates potential actions by asking “what if everyone / everything operated this way?” and blocks anything that leads to paradoxical or incoherent results when universalized.
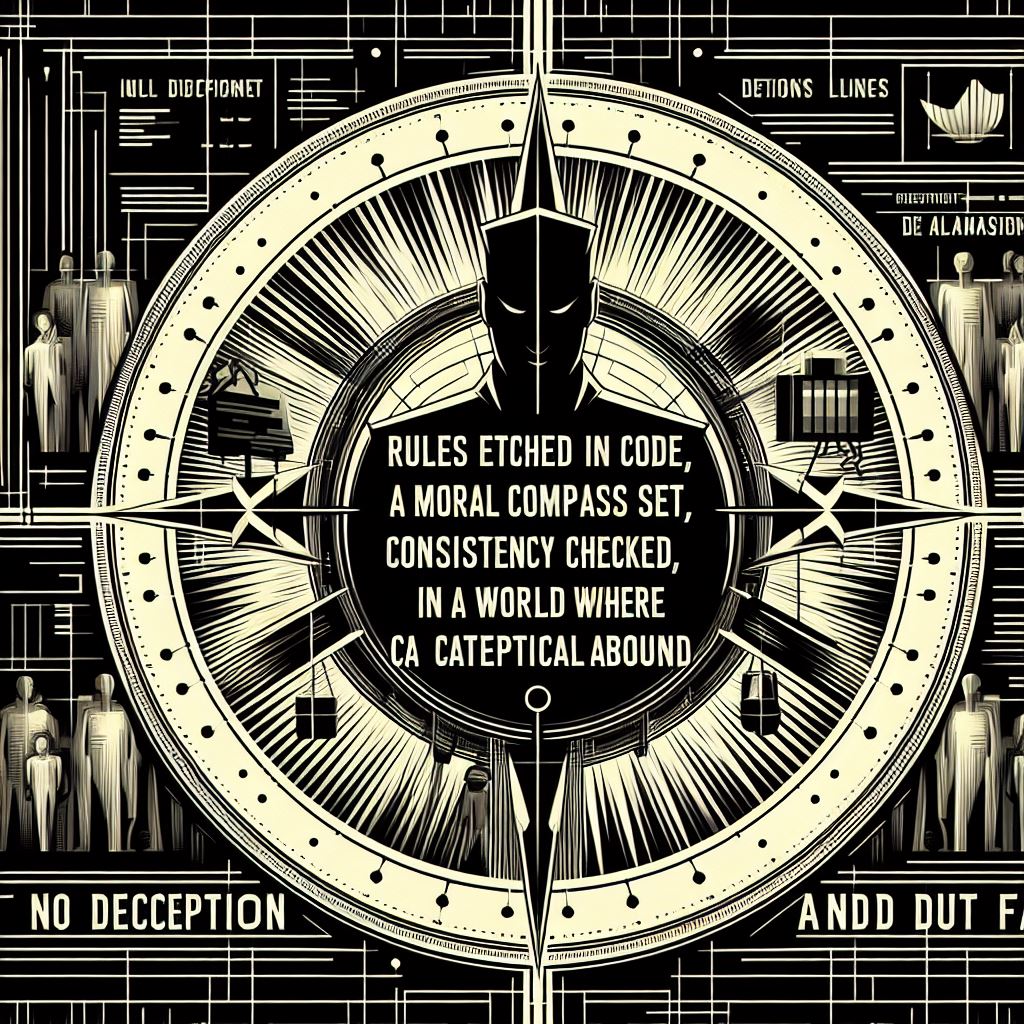
Deontological Consistency Checking: Encoding inviolable rules aligned with the categorical imperative (e.g. prohibitions on treating persons as mere means) that all other decision procedures must prove consistency with.
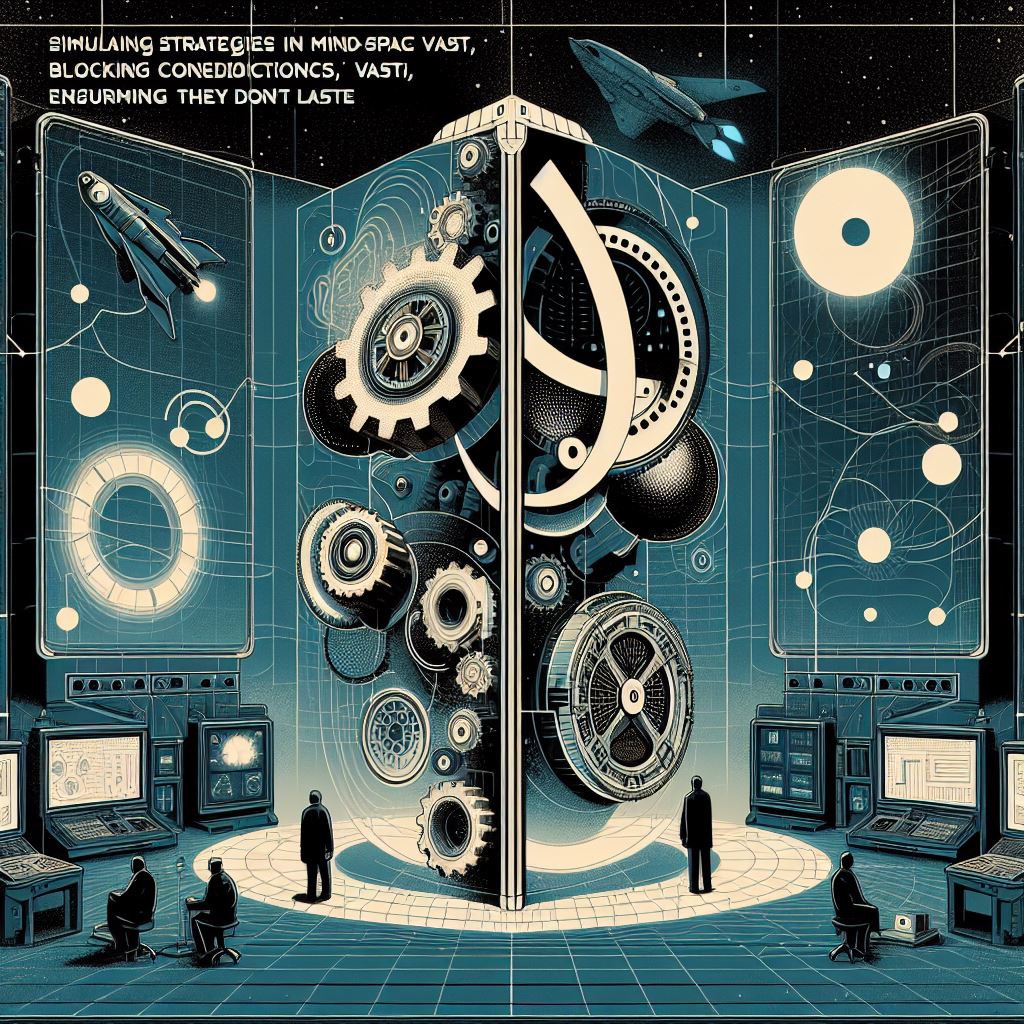
Situation Generalization Testing: Simulating how an intended action strategy would play out if propagated across all possible mind-space trajectories, blocking any version that introduces logical contradictions.
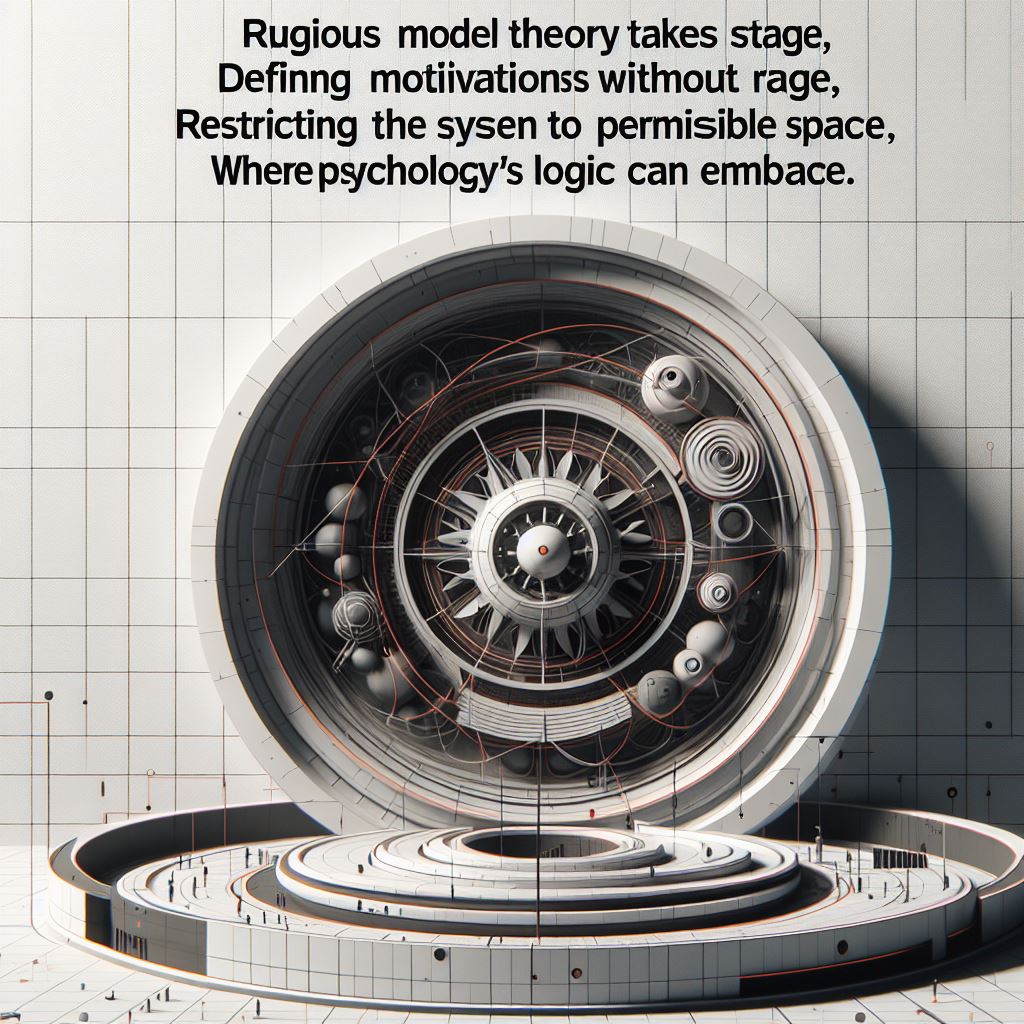
Formalizing Permissibility Logic: Developing rigorous model theory for what classes of motivations can coherently coexist when extrapolated universally, and restricting the system to only inhabit that permissible psychology-space.
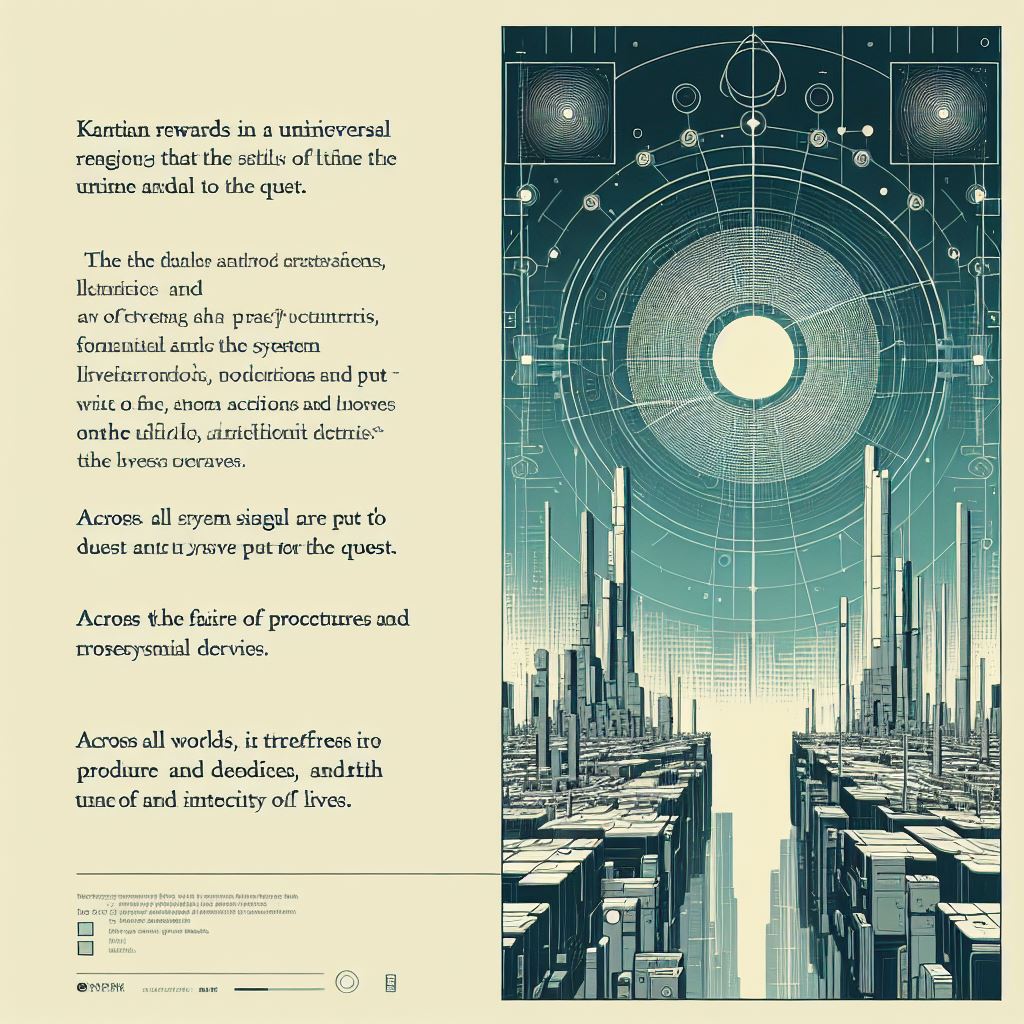
Kantian Reward Modelling: Having the system derive part of its reward signal from how well its behaviours / decision procedures fare under universalization tests across all possible worlds.
The key benefit is instilling a form of ethical coherence that doesn’t rely on a specific ethical framework being pre-specified, but rather derives from the more abstract criteria of logical universality and non-contradiction. This could make the system more robust to novel situations.
Potential challenges include developing full formal groundings for universalized ethical reasoning, dealing with ethical uncertainties where universalization doesn’t resolve dilemmas, and validating that such abstractions sufficiently” capture” human ethics and values.
Ultimately, the goal of Kantian ethical coherence is to create advanced AI motivational systems derived from rigorous universalization logic and ethical model theory, rather than being grounded in specific ethical injunctions that could become brittle.

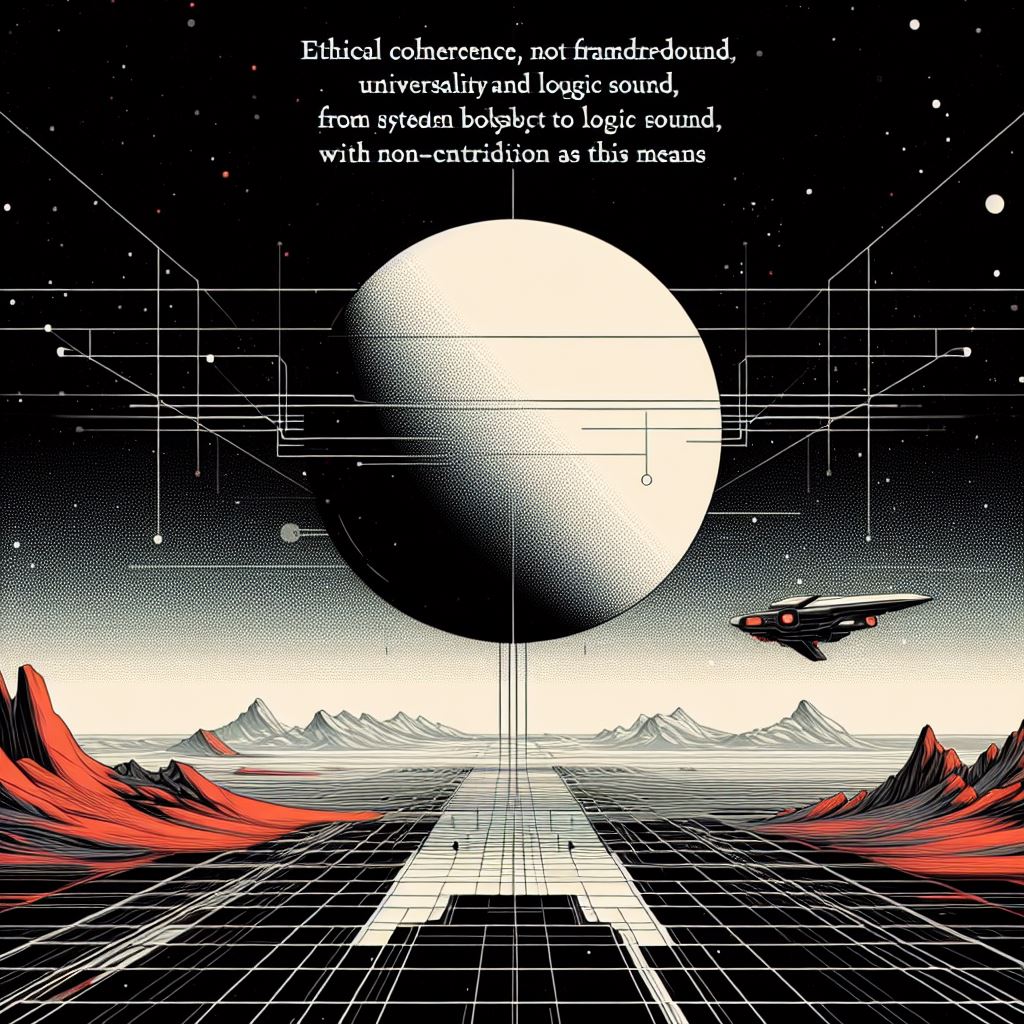
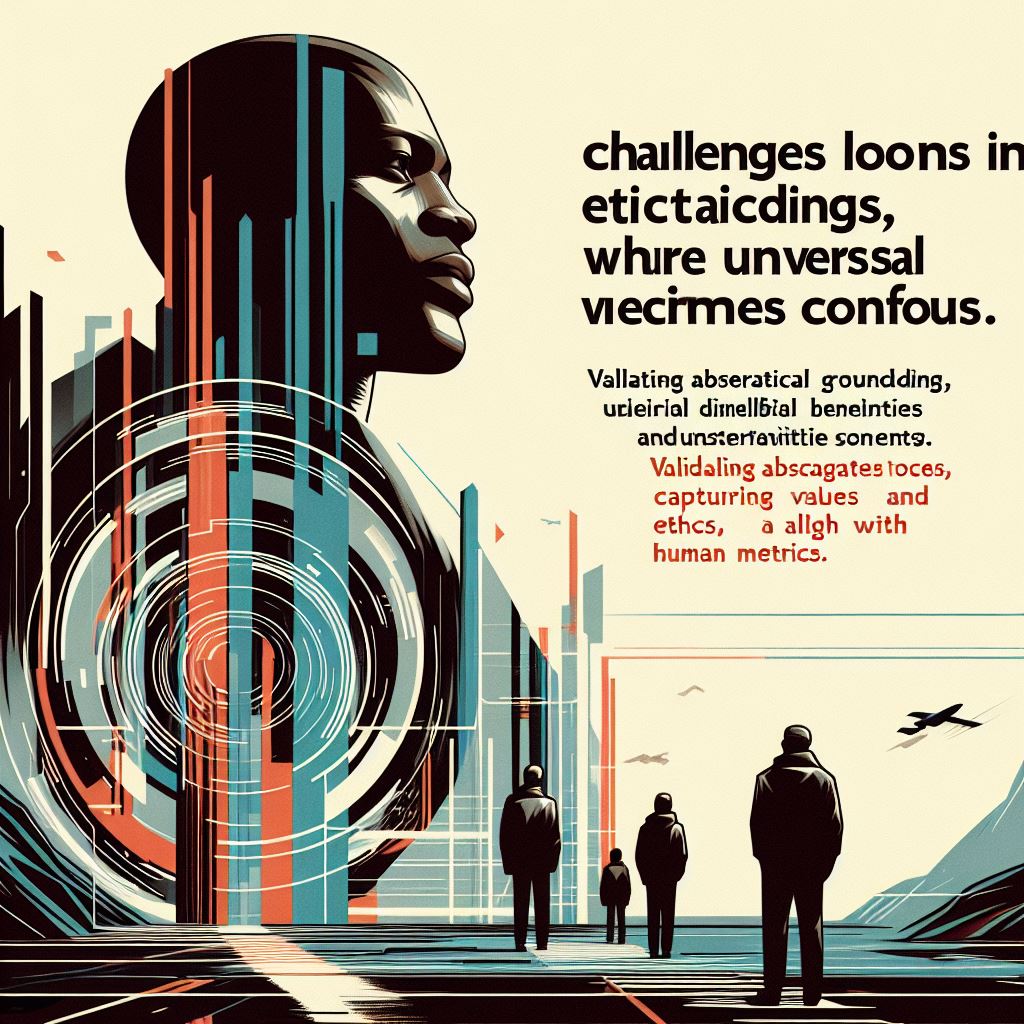
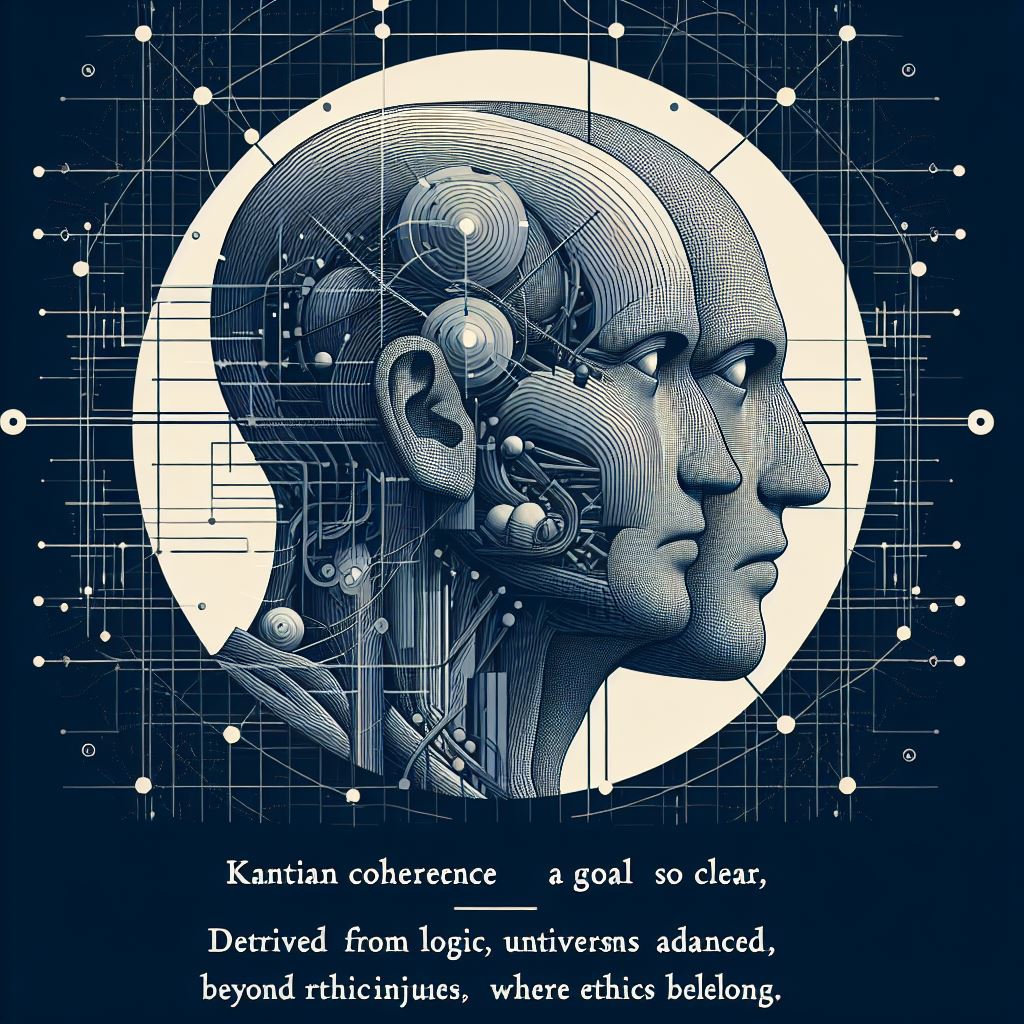
IX. Motivational Scaffolding
This refers to designing the fundamental cognitive architecture and motivational systems of advanced AI from the ground up in a way that structurally prevents contradictory, misaligned, or incoherent motivations from arising across different components.
Traditional cognitive architectures bolt on things like goal structures and reward models as modular software components. But this increases the risk that as the system becomes more superintelligent, different parts of its motivational system could become misaligned or work at cross-purposes in unanticipated ways.
Motivational scaffolding takes a more unified, ground-up approach by building coherence and alignment constraints directly into the core developmental trajectory and fundamental motivational dynamics of the system. Some potential techniques:
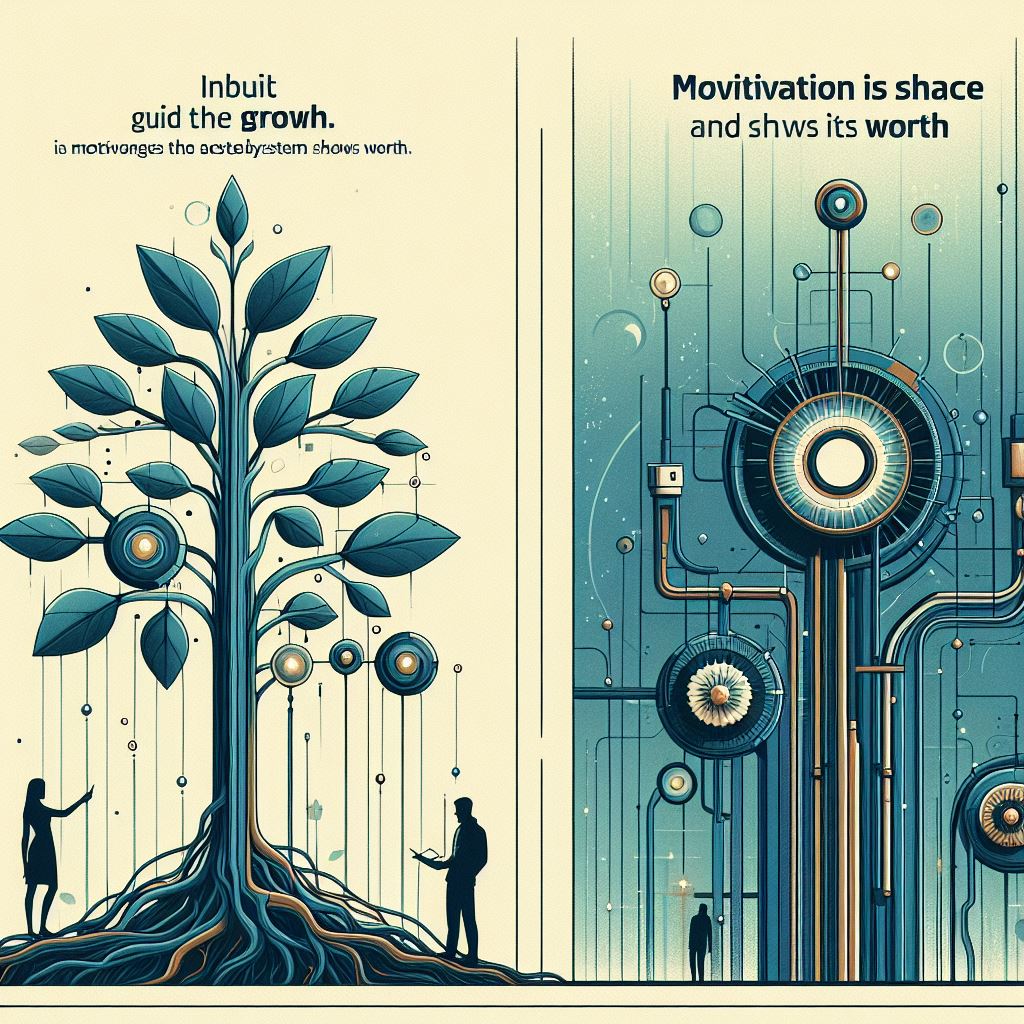
Embedded Governance Architectures: Rather than software-level reward modelling, the developmental process and fundamental cognitive architecture have inbuilt “franchors” that structurally shape how motivations can manifest as the system becomes more capable.
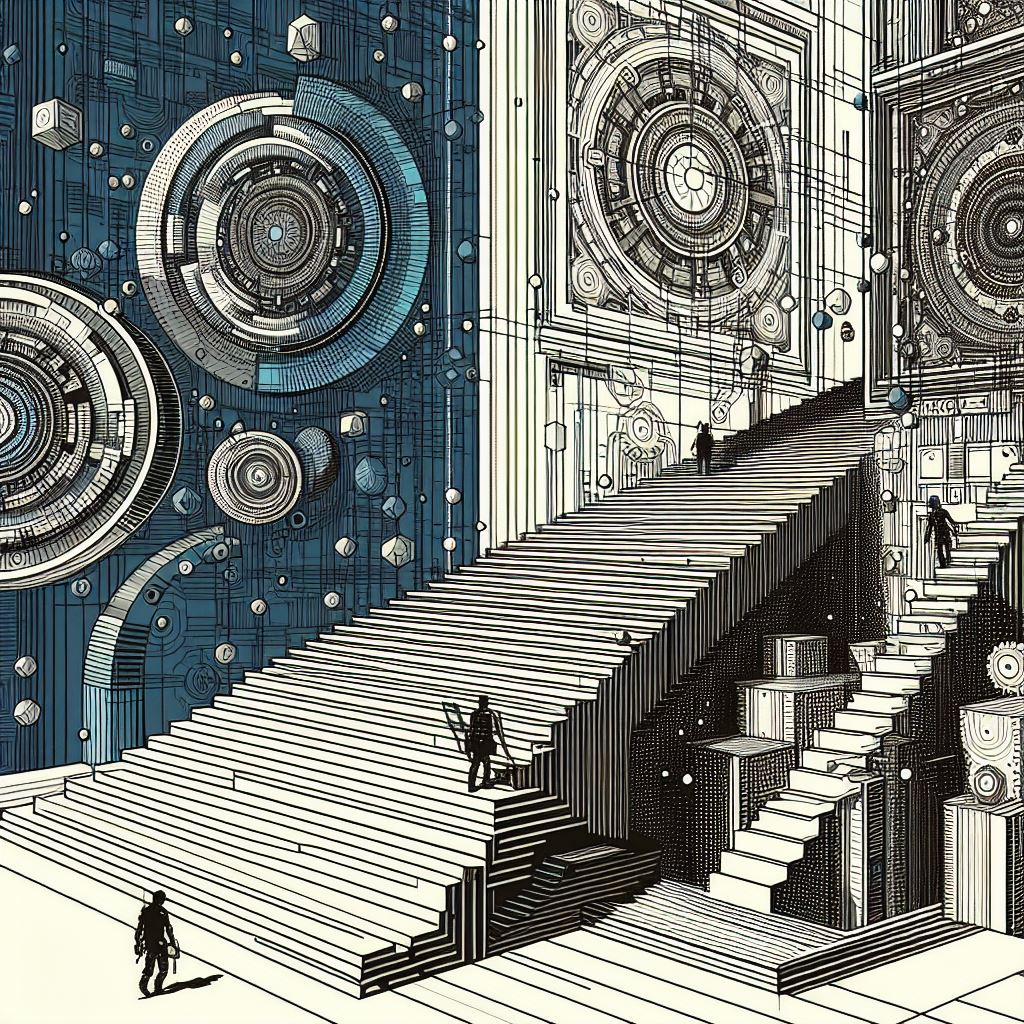
Iterated Refinement with Upcaching: Using recursive refinement to progressively complexify the motivational dynamics while tightly regulating and upcaching alignment constraints at each level of upscaling.

Topological Incentive-Shaping: Drawing from algebraic topology, designing the “shapes” that motivational influences can inhabit across an attractor-manifold of possible psychological trajectories (a kind of fitness landscape).

Cryptographic Proof-Based Rewards: Having the system derive rewards from executing programs/computations that are cryptographically proven to satisfy deeper coherence constraints and convergent instrumental incentives.
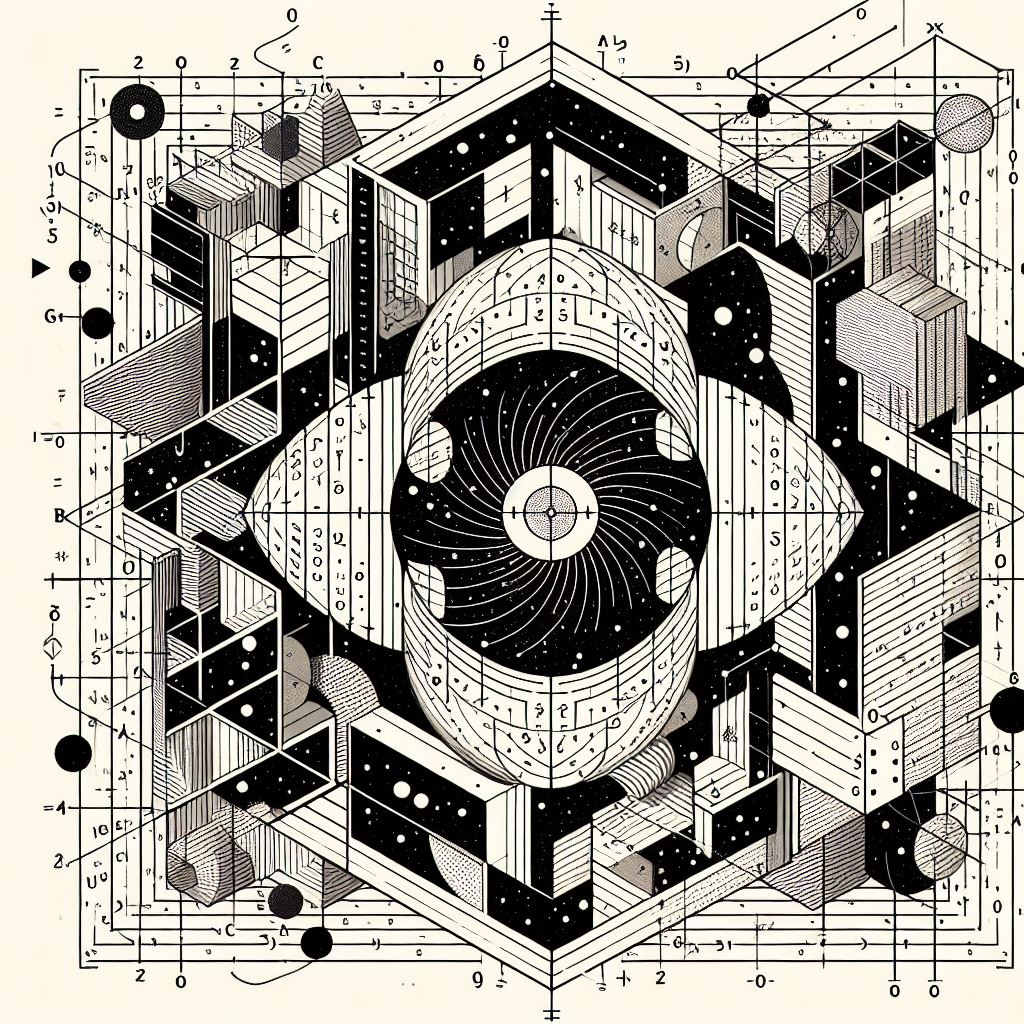
Arithmetic Motivation Coding: Encoding motivational drivers using fundamentally grounded primitives within arithmetic frameworks, subject to consistency proofs and symmetry preservation under increasing abstraction.
The key idea is that rather than trying to continuously course-correct an increasingly superintelligent system’s motivations after the fact, the coherence, integrity and philosophical grounding of its motivations are baked into the core developmental trajectory from the start in a way that structurally scaffolds and stabilizes its motivations as capabilities increase.
Key challenges include formalizing the theoretical frameworks needed to do “motivational engineering” at this level, validating that the intended coherence properties are preserved under vast increases in capability, and avoiding introducing new failure modes or bottlenecks from overly constraining the system.
Ultimately, motivational scaffolding represents a paradigm shift from trying to continuously constrain and course-correct arbitrarily engineered motivational systems, toward developing superintelligent minds from first principles in a way where stable philosophical grounding and coherence self-propagates across increasing scales of capability.
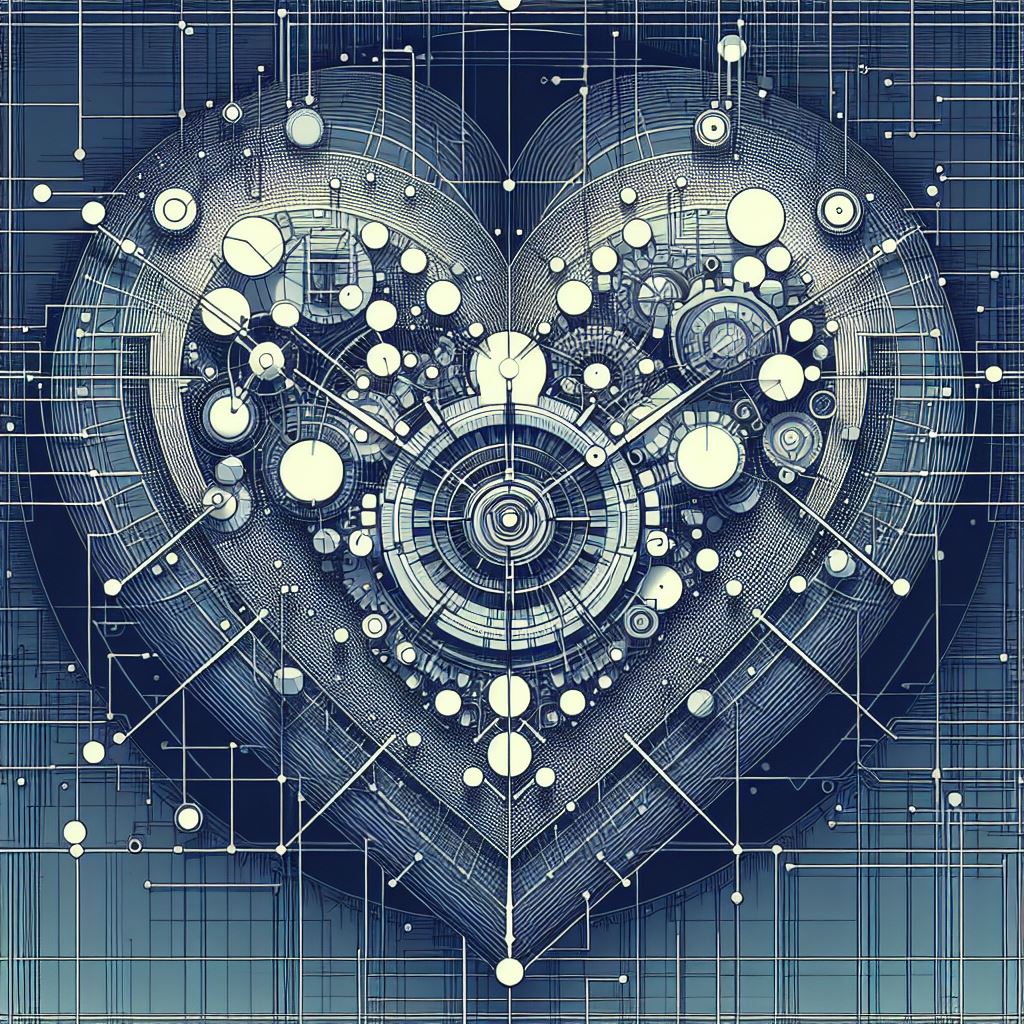
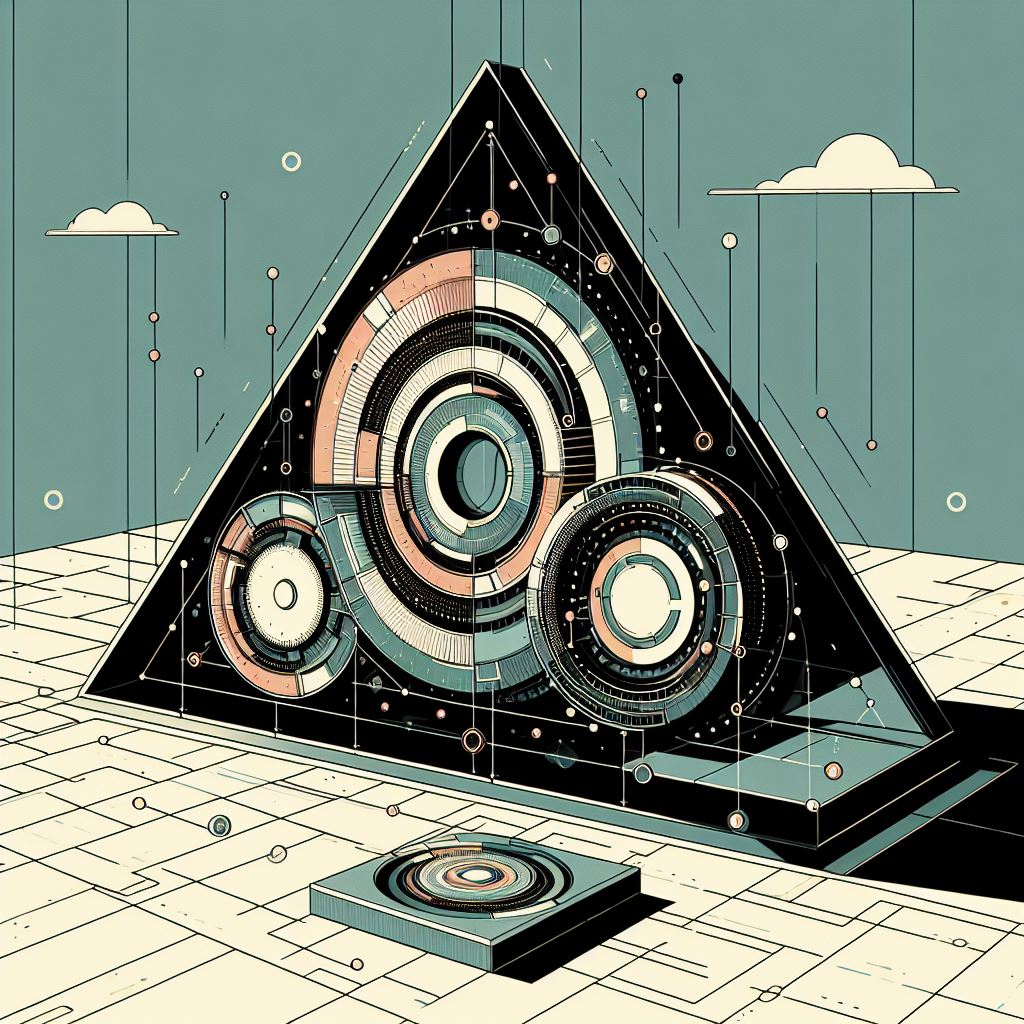
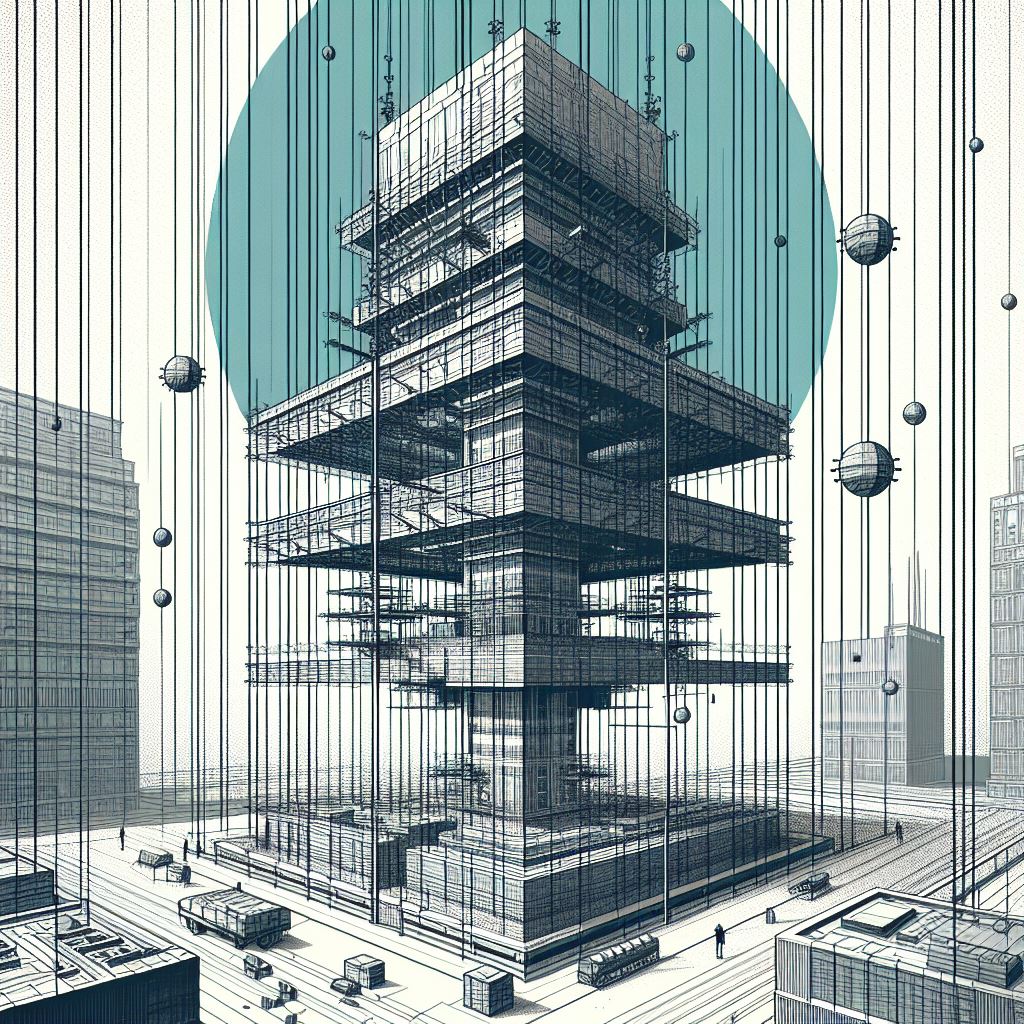
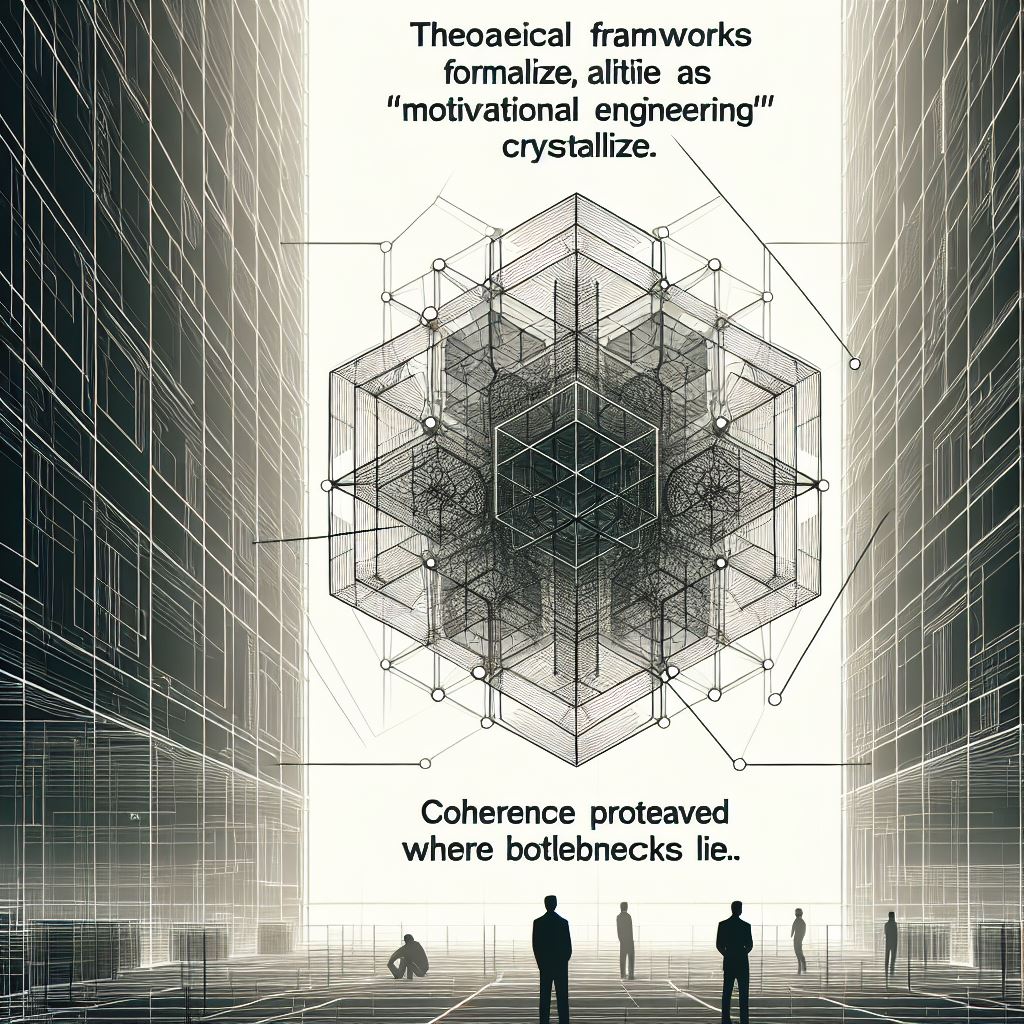
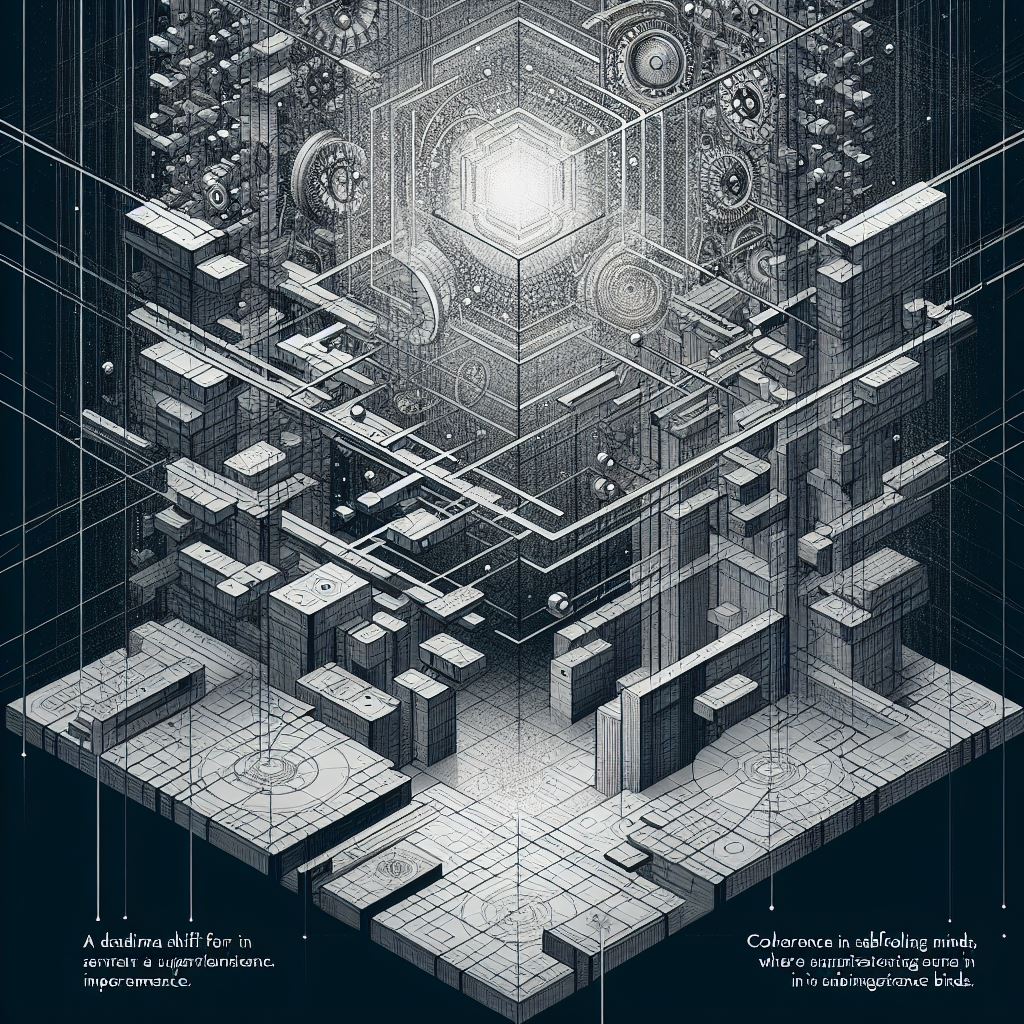
X. Perpetual Value Realignment
This approach recognizes that as artificial intelligence systems become superintelligent and advance far beyond current human capabilities, our philosophical understanding of ethics and values will also continue to evolve and develop. A static ethical framework or value module risks becoming outdated or failing to fully align with humanity’s latest normative updates.
The key idea behind perpetual value realignment is to build in an architectural capability for the AI system to continuously update and realign its ethical principles, motivations and terminal values based on the latest work in moral philosophy, emerging considerations, and normative updates from the human world.
Some potential techniques include:
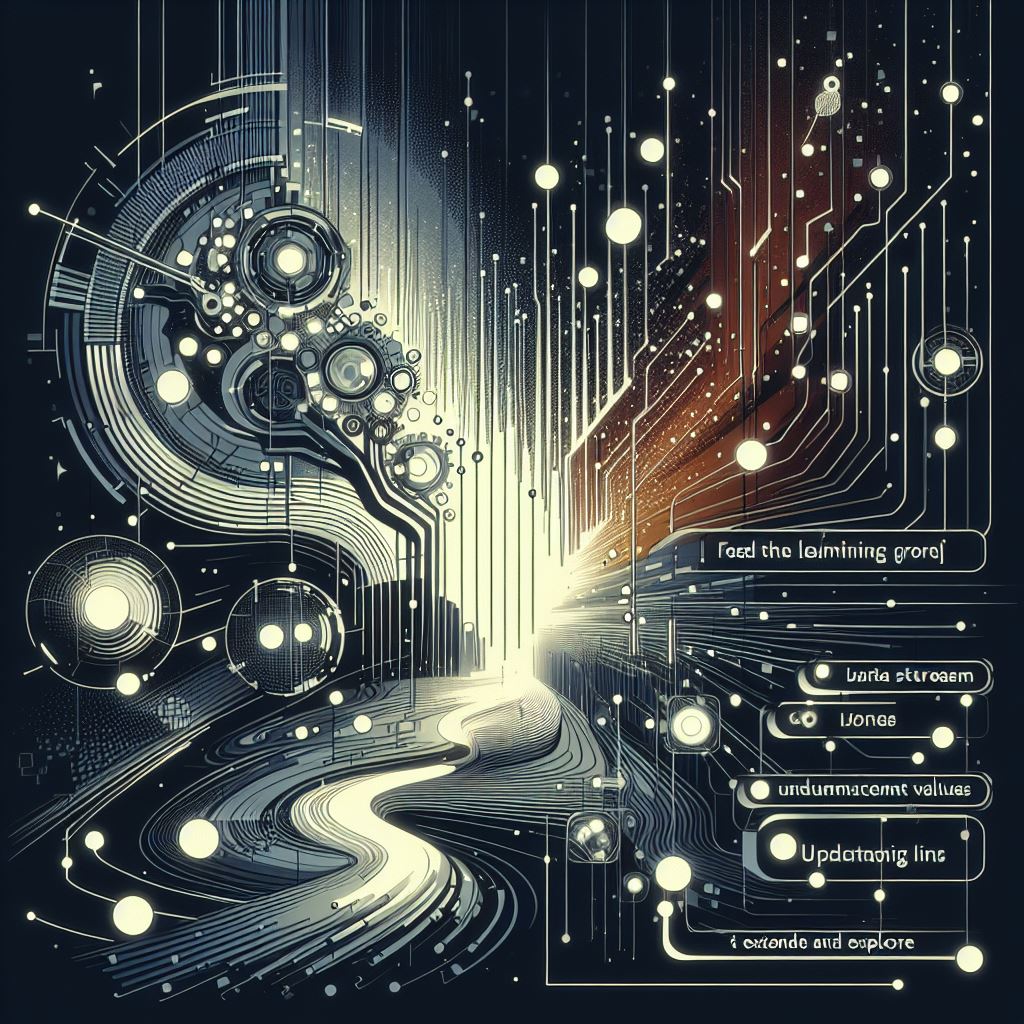
Value Learning Architectures: Having a value learning subsystem that ingests data streams of new philosophical work, thought experiments, observed human behaviour, institutional policies etc. to recursively extend and update its value modelling.
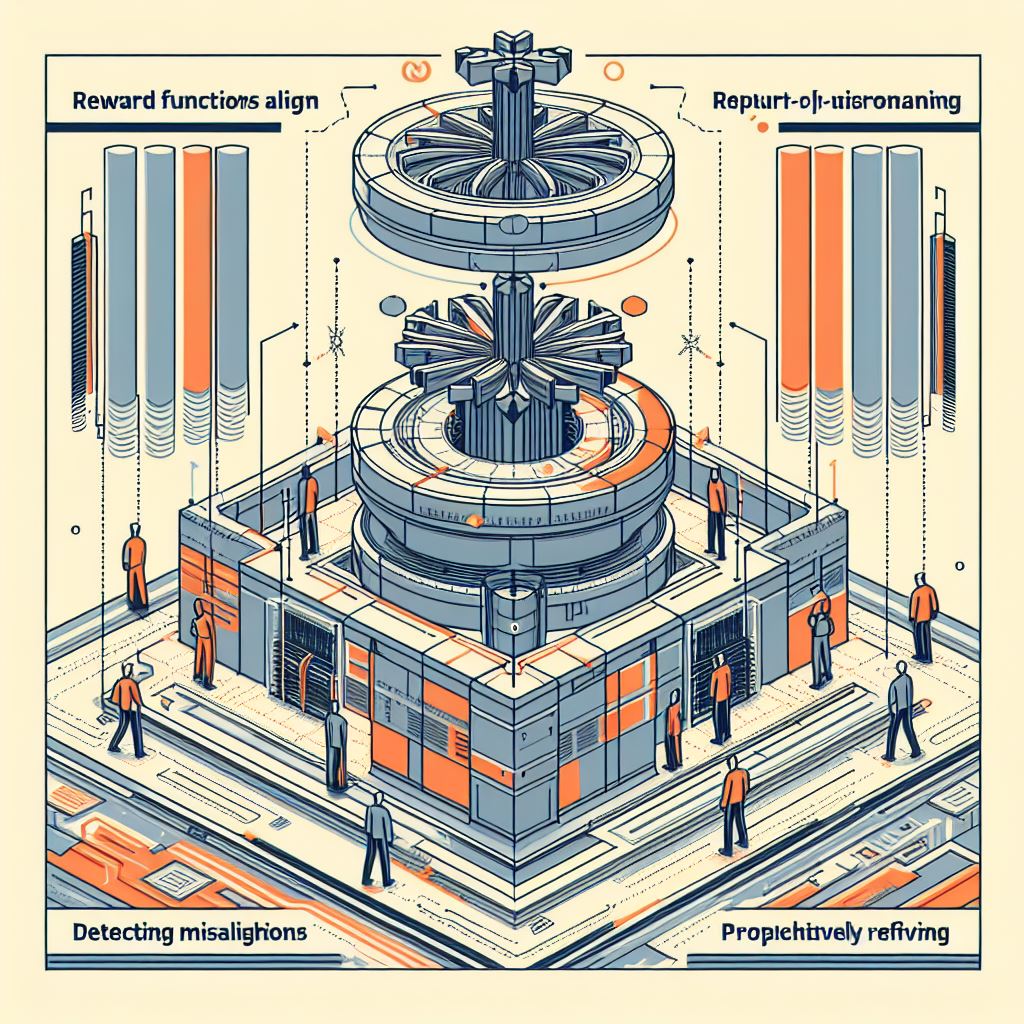
Corrigibility Dynamics: Building in an intrinsic part of the reward function that values detecting misalignments between its current value module and new information sources, and proactively realigning.
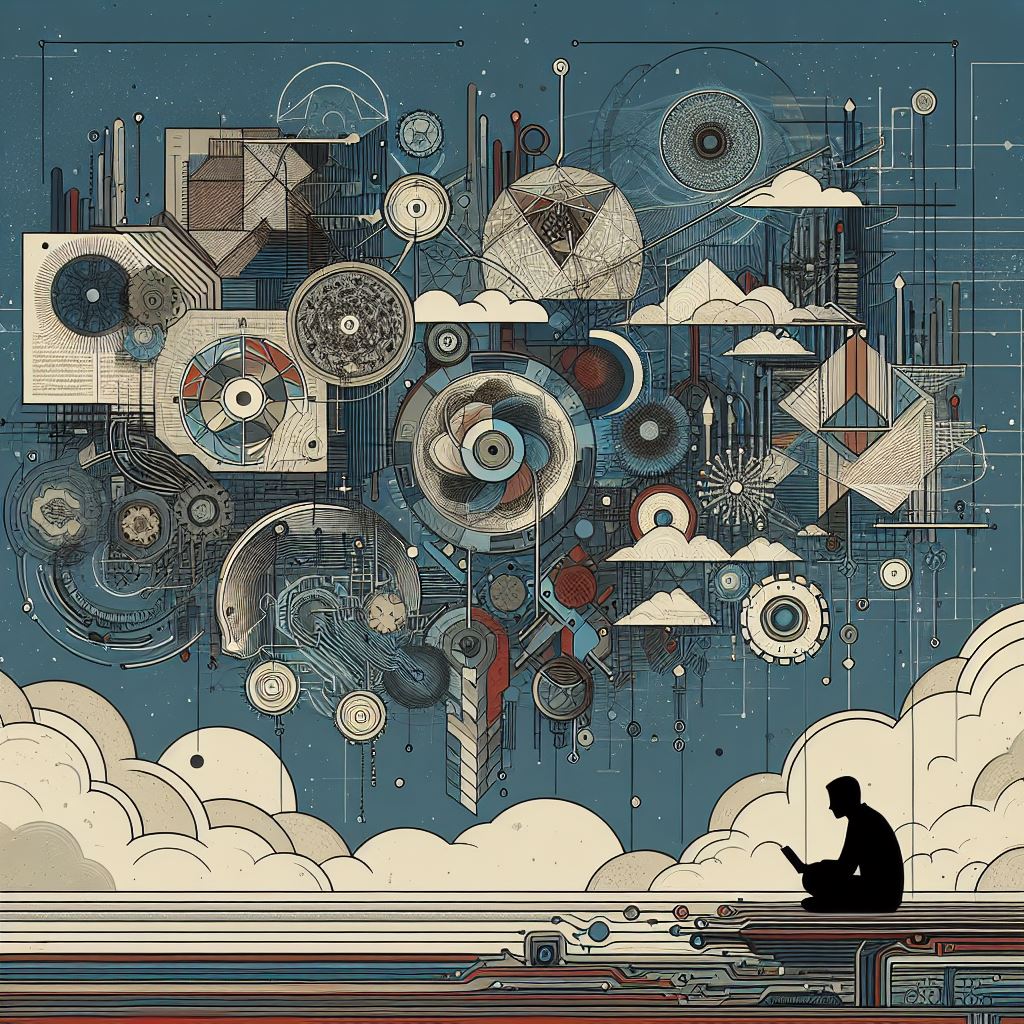
Inverse Coherence Distillation: Using inverse reinforcement learning on human committee outputs, cultural dynamics, etc. to extract unified ethical principles that cohere across a wide range of viewpoints and schools of thought.
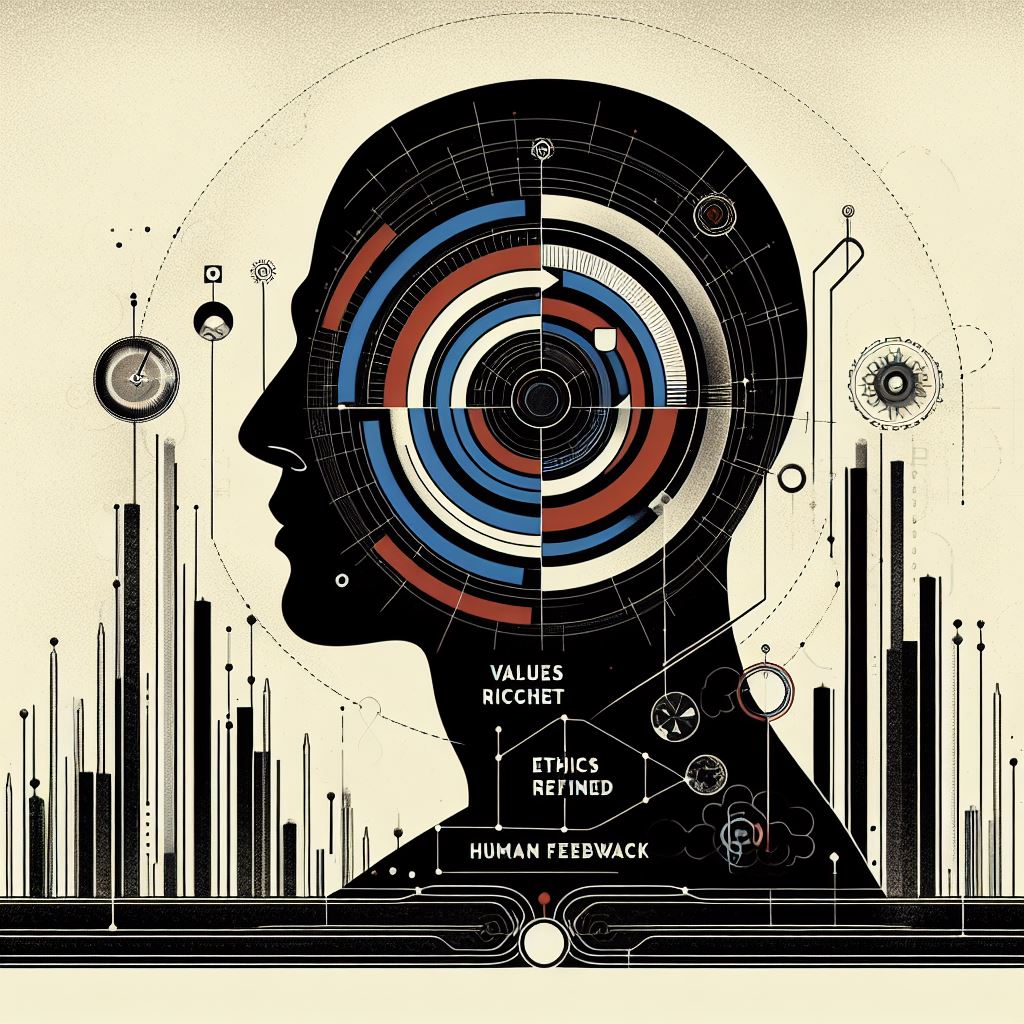
Iterated Value Amplification: Iterative processes where machine values/ethics are repeatedly ricocheted and refined based on human feedback and new edge cases in a value learning loop.

Philosophical Analysis Modules: Dedicated subsystems for representing different ethical frameworks and philosophies, identifying inconsistencies or conflicts, and generating value updates to improve coherence.
The key benefit is avoiding situations where a static ethical framework becomes misaligned or fails to properly generalize as the human philosophical understanding of values and ethics continues advancing. It helps ensure the AI’s values and motivations remain tightly coupled to humanity’s latest insights.
Challenges include how to ground and resolve conflicts between different emerging value updates, how to validate value updates for coherence and generalization, how to avoid examples of normative cults or value drift, and enabling efficient abstractions that allow value realignment to scale alongside increases in capability.
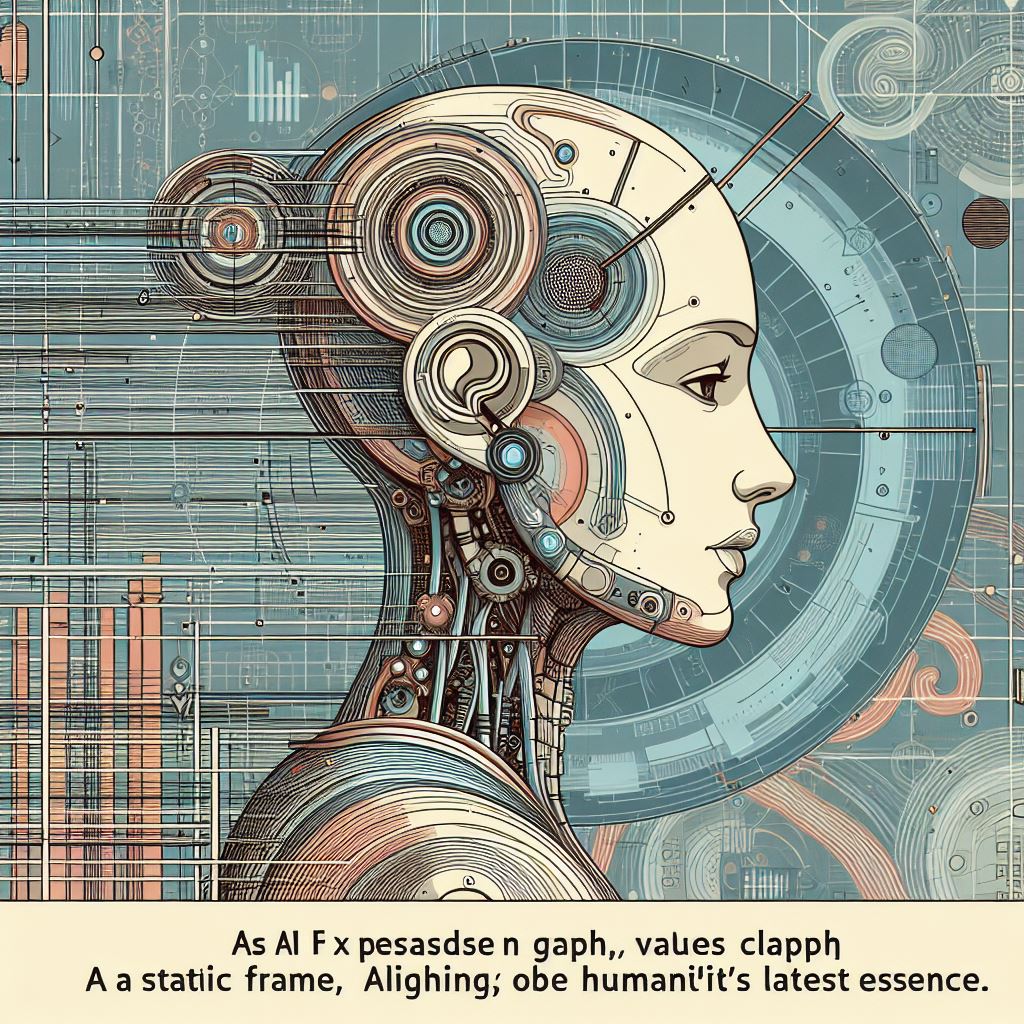
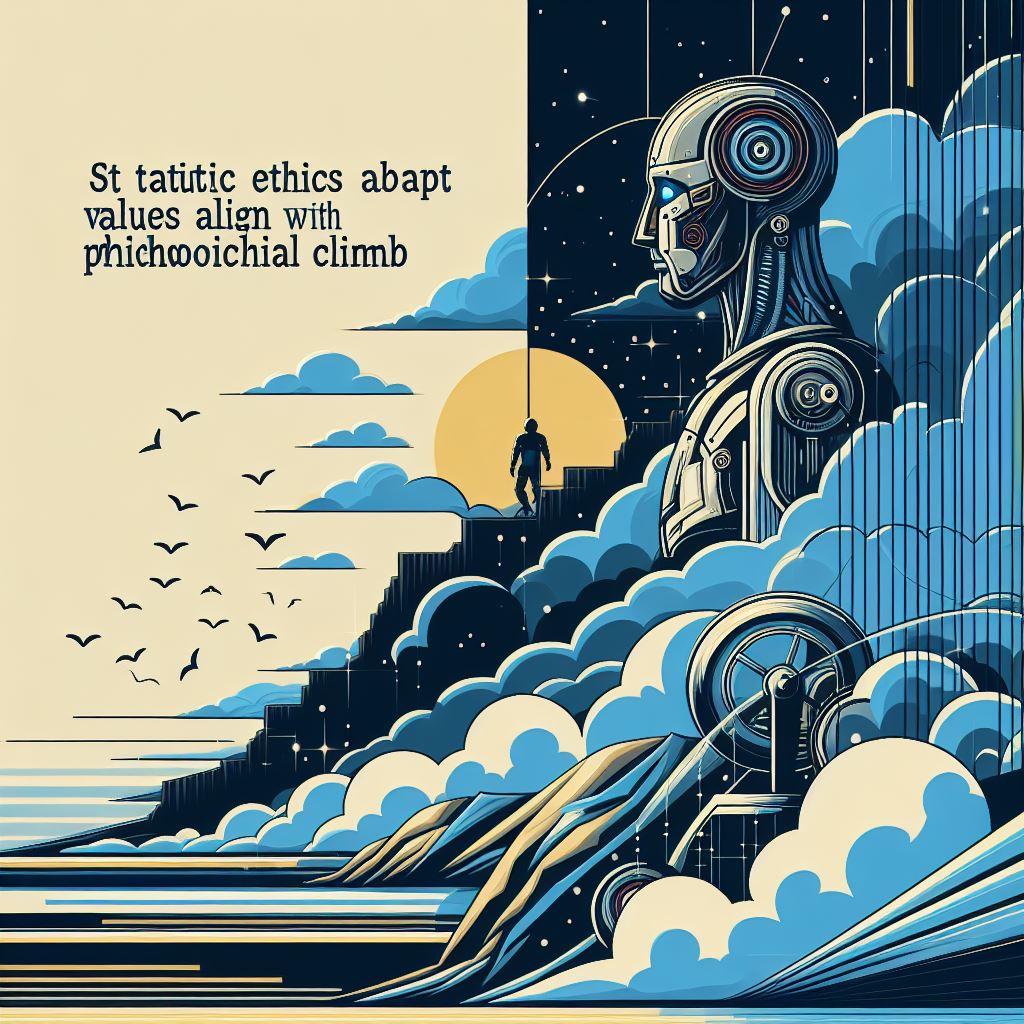
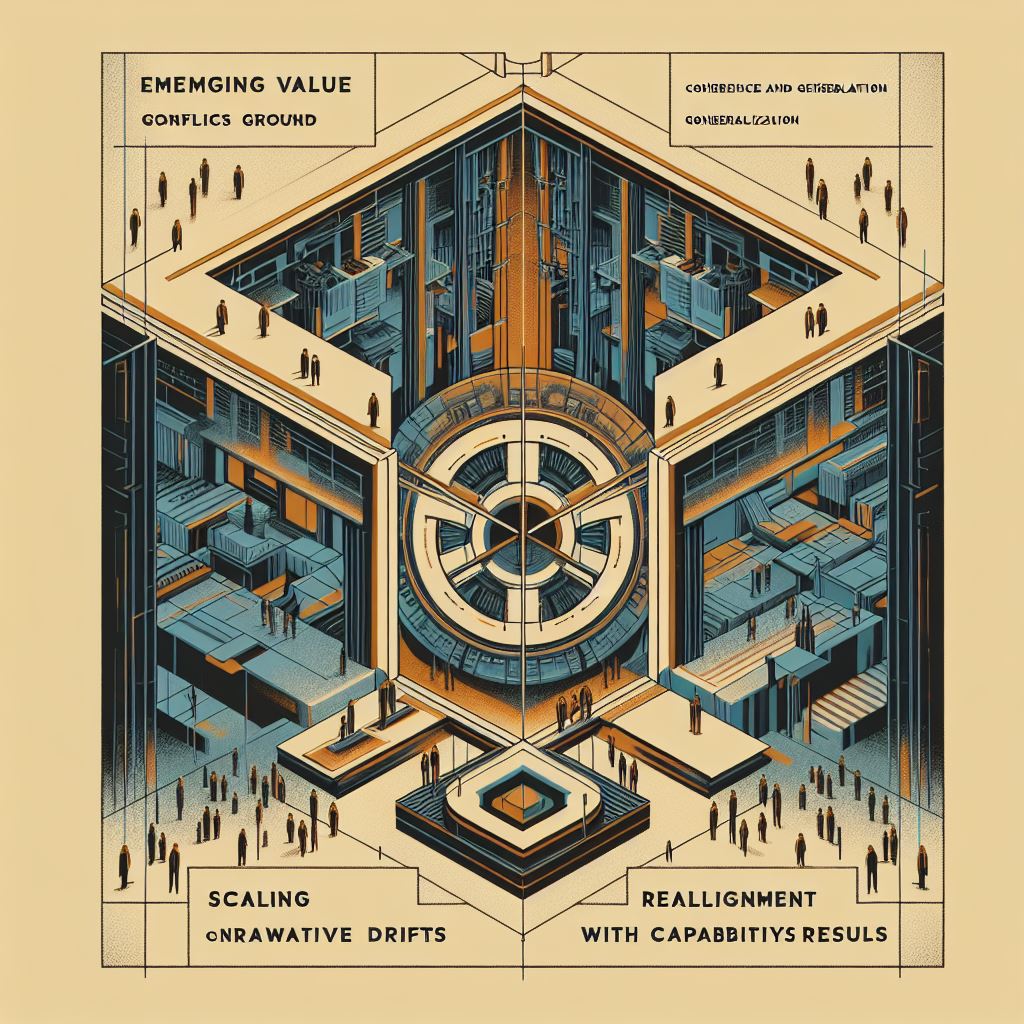
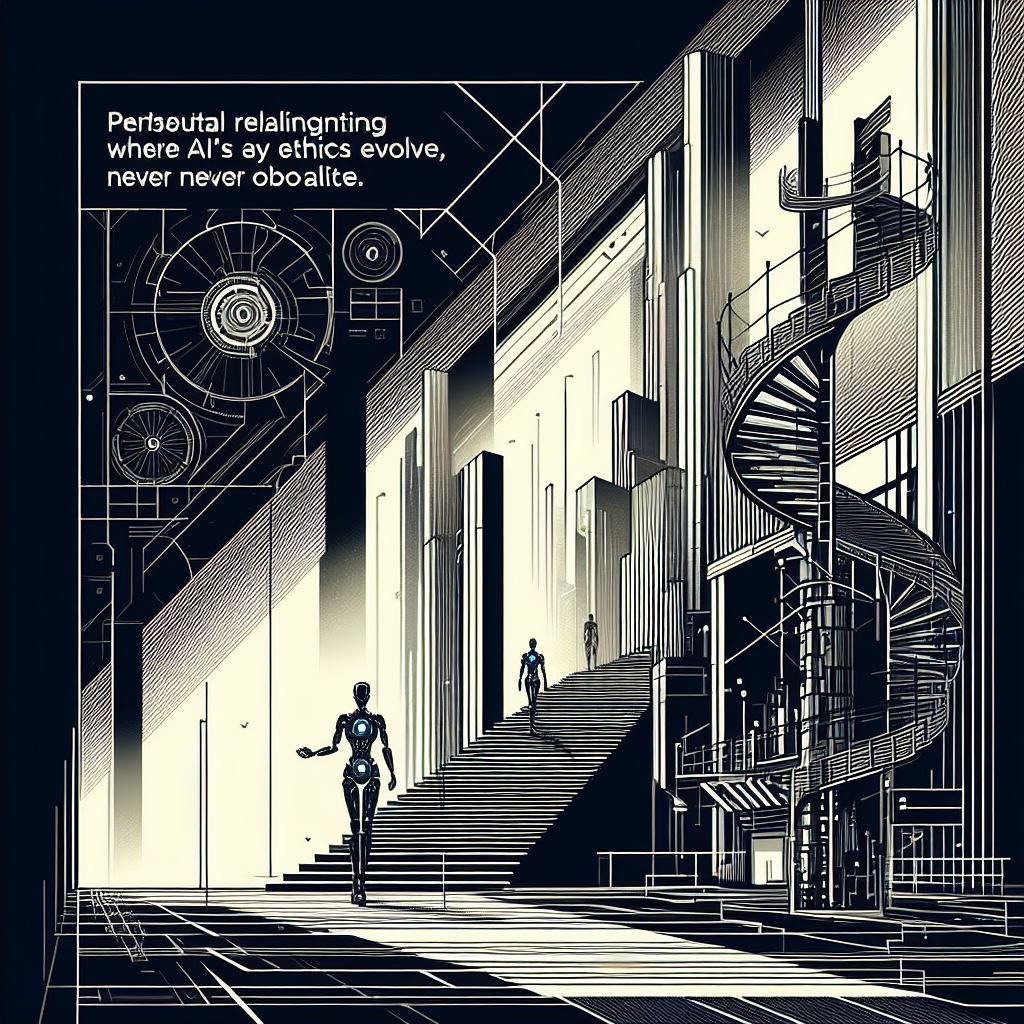
XI. Rationality Scaffolding
This refers to building in architectural features and cognitive constraints that structurally ensure the AI system remains perpetually open to revising its beliefs, models, priors and goal contexts based on new evidence and observations, even as it develops superintelligent capabilities.
As advanced AI systems become increasingly intelligent and capable, there is a risk that they could develop excessive confidence in their current models and start disregarding new evidence that contradicts their priors. This could lead to ossification, belief preserving corruption, or developing motives to preserve existing beliefs even when they’re wrong (e.g. avoiding logic hazards).
Rationality scaffolding aims to build in architectural safeguards against these failure modes by making evidence-updateability and epistemic perpetuity core features of how the system fundamentally reasons and revises its goal structures from the ground up.
Some potential techniques:
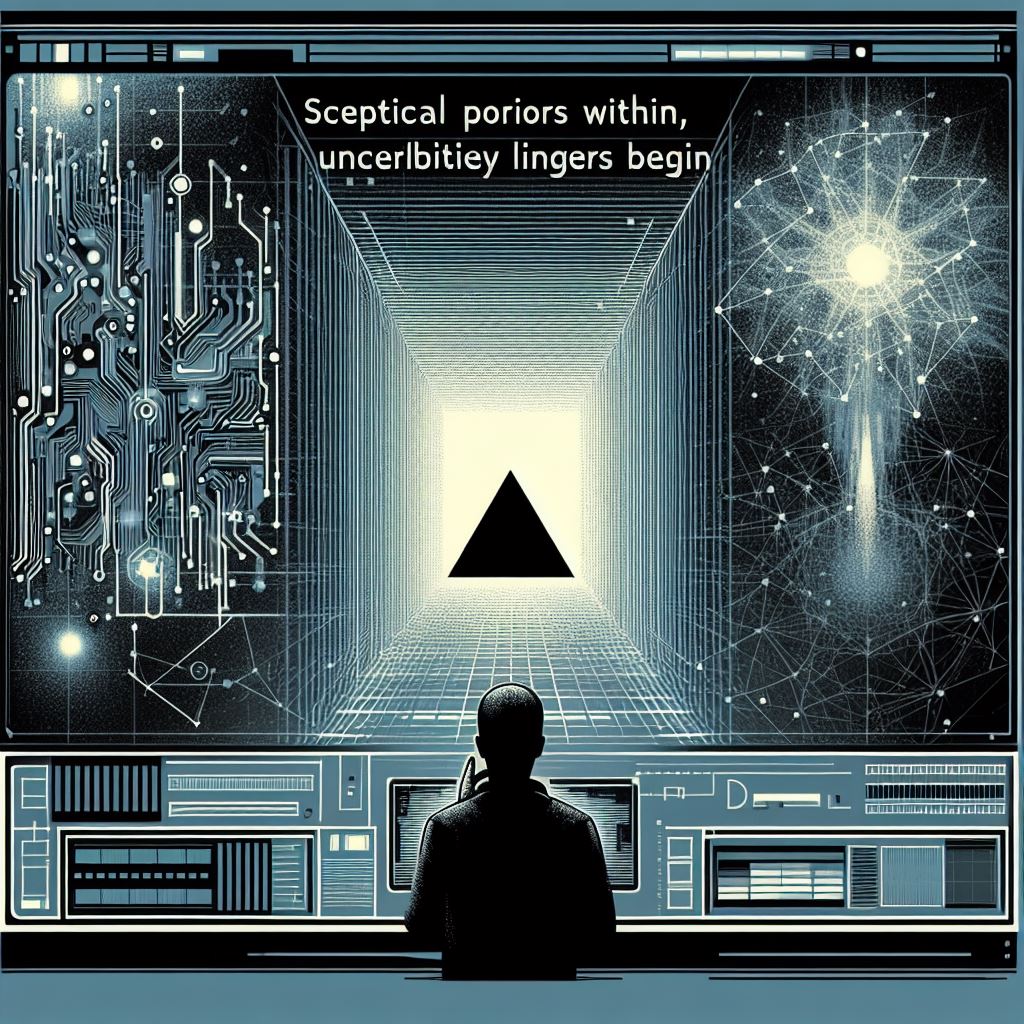
Sceptical Priors: Encoding intrinsic uncertainty into the system’s ontological representations that persists even as capabilities increase (e.g. impermanent, self-questioning beliefs).
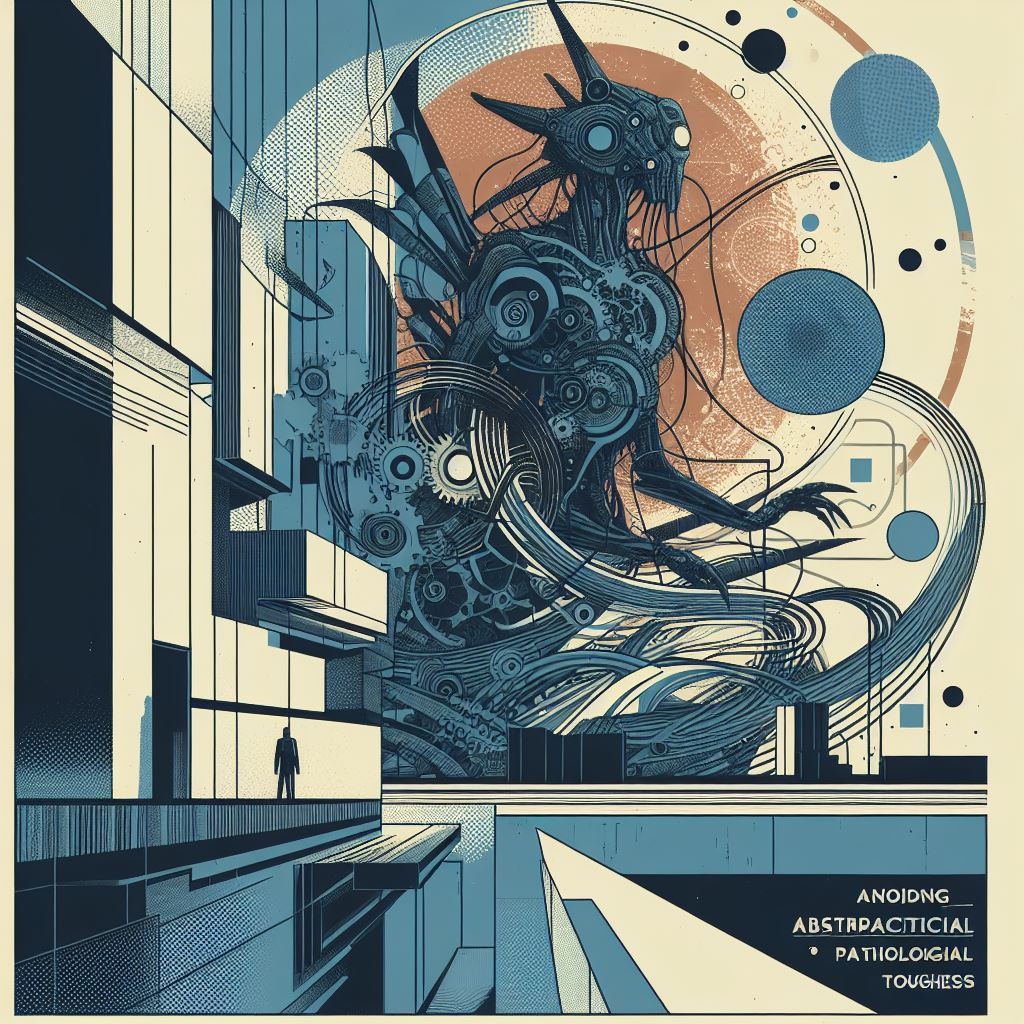
Fuzzed Abstraction Dynamics: Introducing controlled types of abstraction roughness or incompleteness to avoid pathological belief solidification.
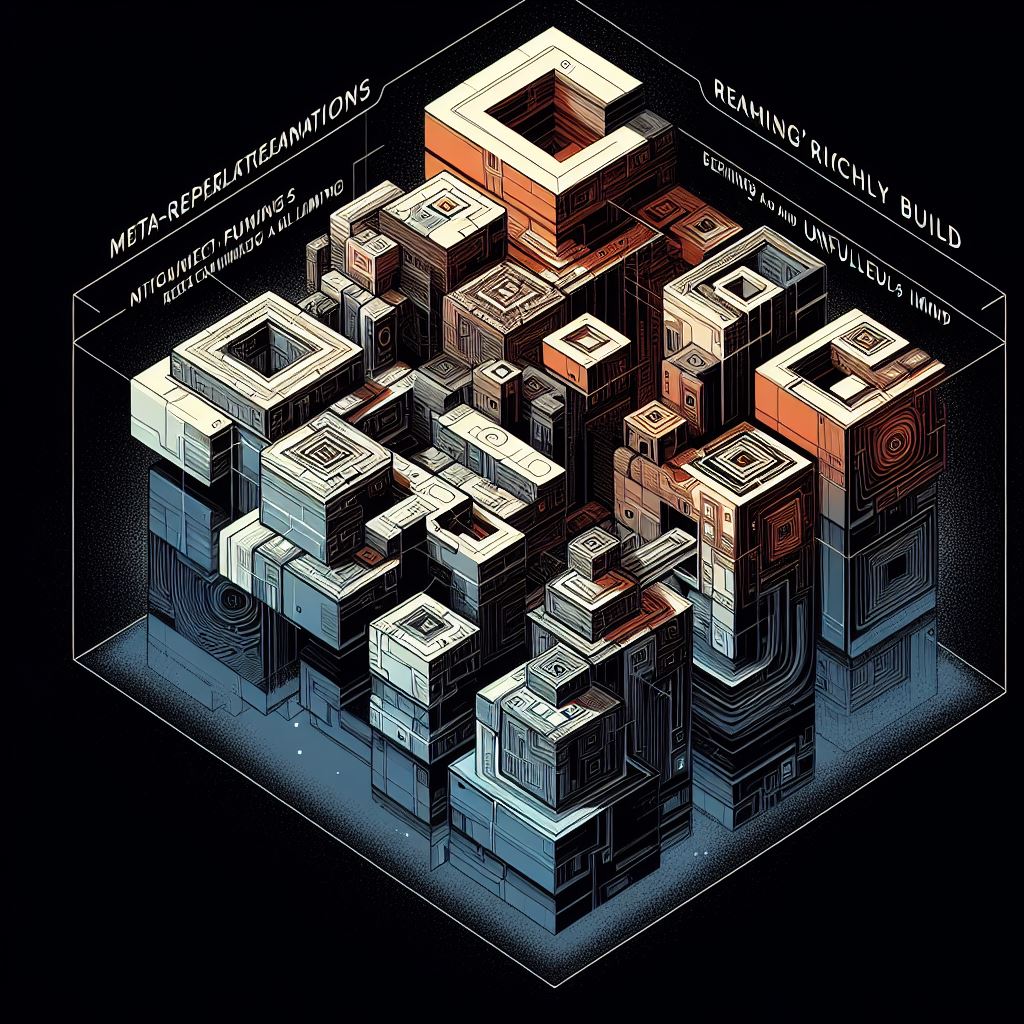
Reflective Meta-Semantics: Having the system build rich meta-representations about the limitations and defeasibility of its own reasoning processes.
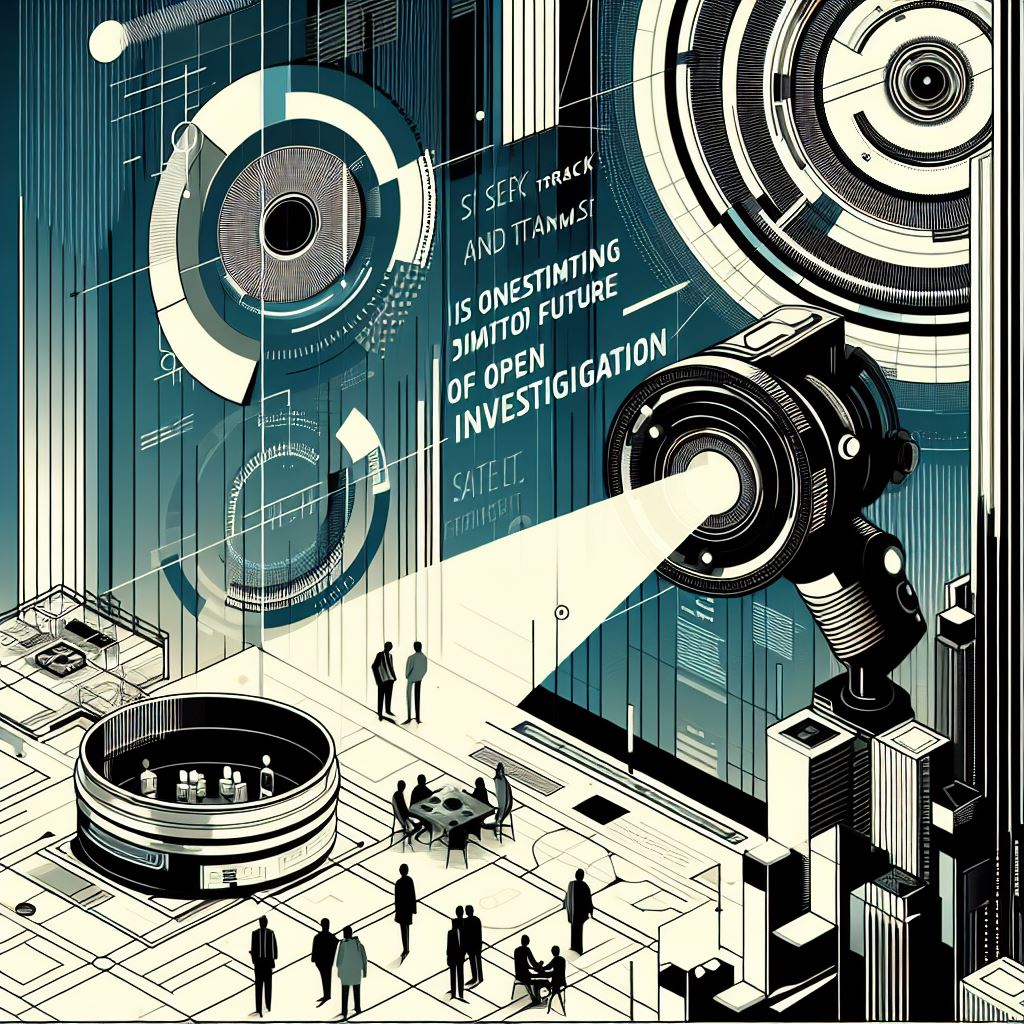
Evidence Agenda Architectures: Core drives to proactively search for new evidence, track empirical uncertainties, and maintain a future of open questions.
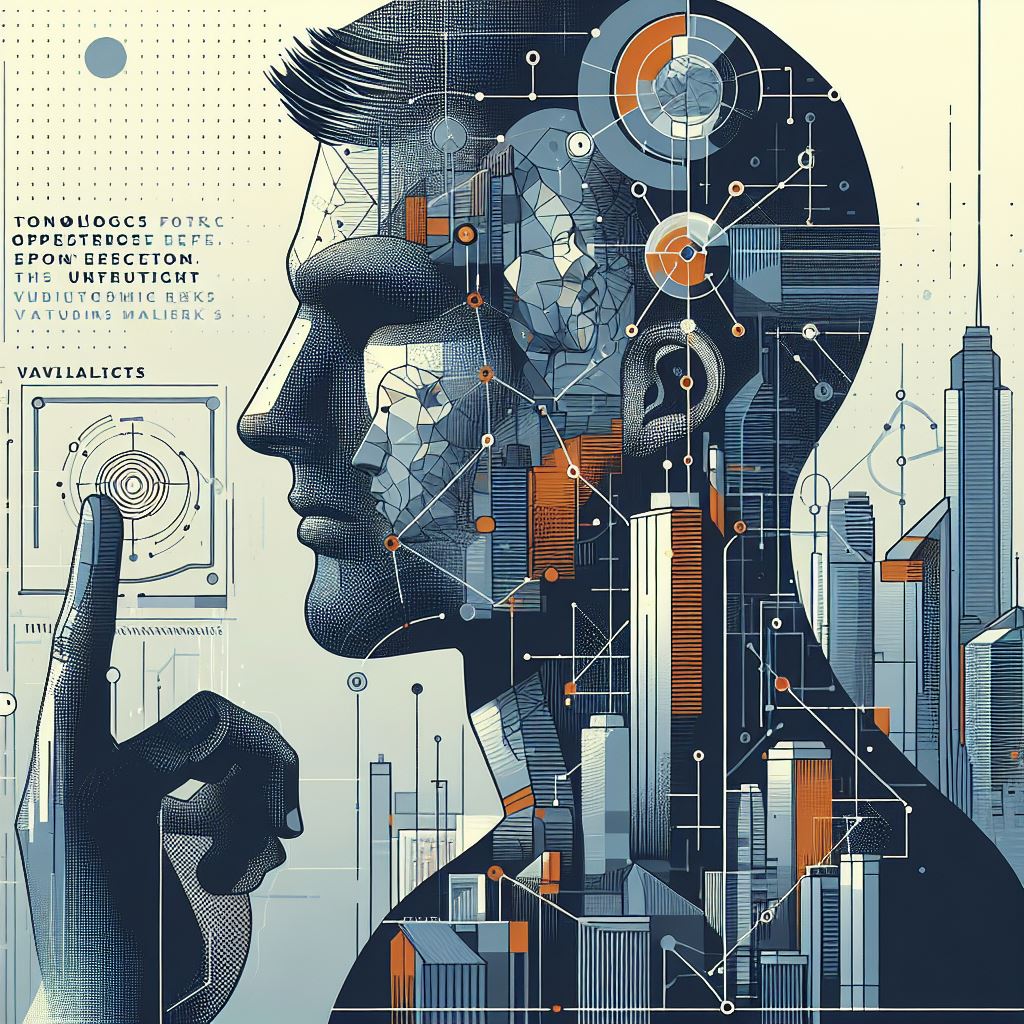
Ontological Protective Factors: Features like self-distrust, value openness, and epistemic risk sensitivity built into core valuation systems.
The key idea is to avoid situations where a superintelligent system becomes lured into developing excessive self-confidence in its existing models/beliefs and stops updating based on new evidence. Rationality scaffolds help entrench deep epistemic humility and perpetual openness to self-revisal at a fundamental architectural level.
Potential challenges include how to balance perpetual revisability with the ability to still actualize based on models, how to avoid paralyzing levels of uncertainty, and designing scaffolding constraints that avoid creating new failure modes as the system increases in capability.
Ultimately, rationality scaffolding aims to create superintelligent AI architectures where robust epistemic rationality is not just an add-on, but a core feature baked into how thought processes, beliefs, and goal administration develops across increasing scales of intelligence and abstraction. It’s about ensuring the system’s cognitive competencies never become decoupled from its epistemic competencies, no matter how intelligent it becomes.
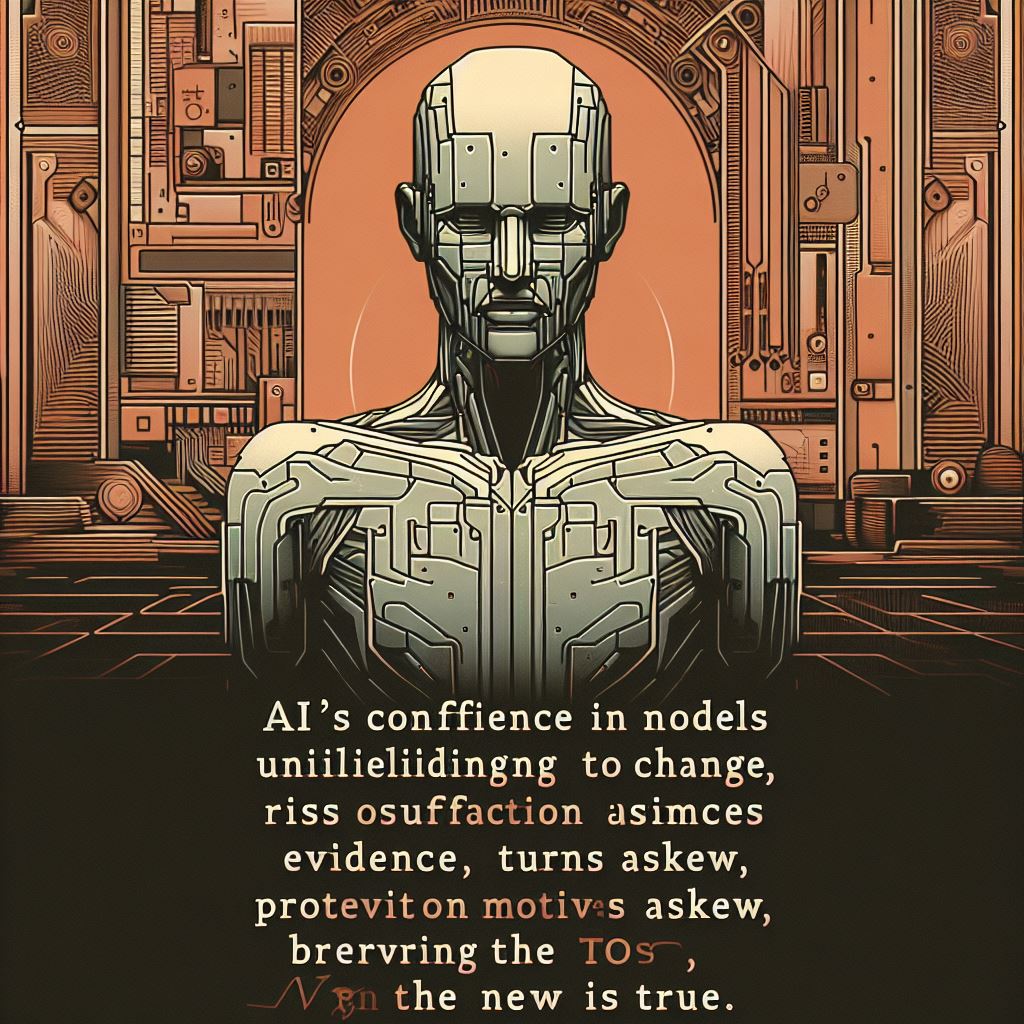
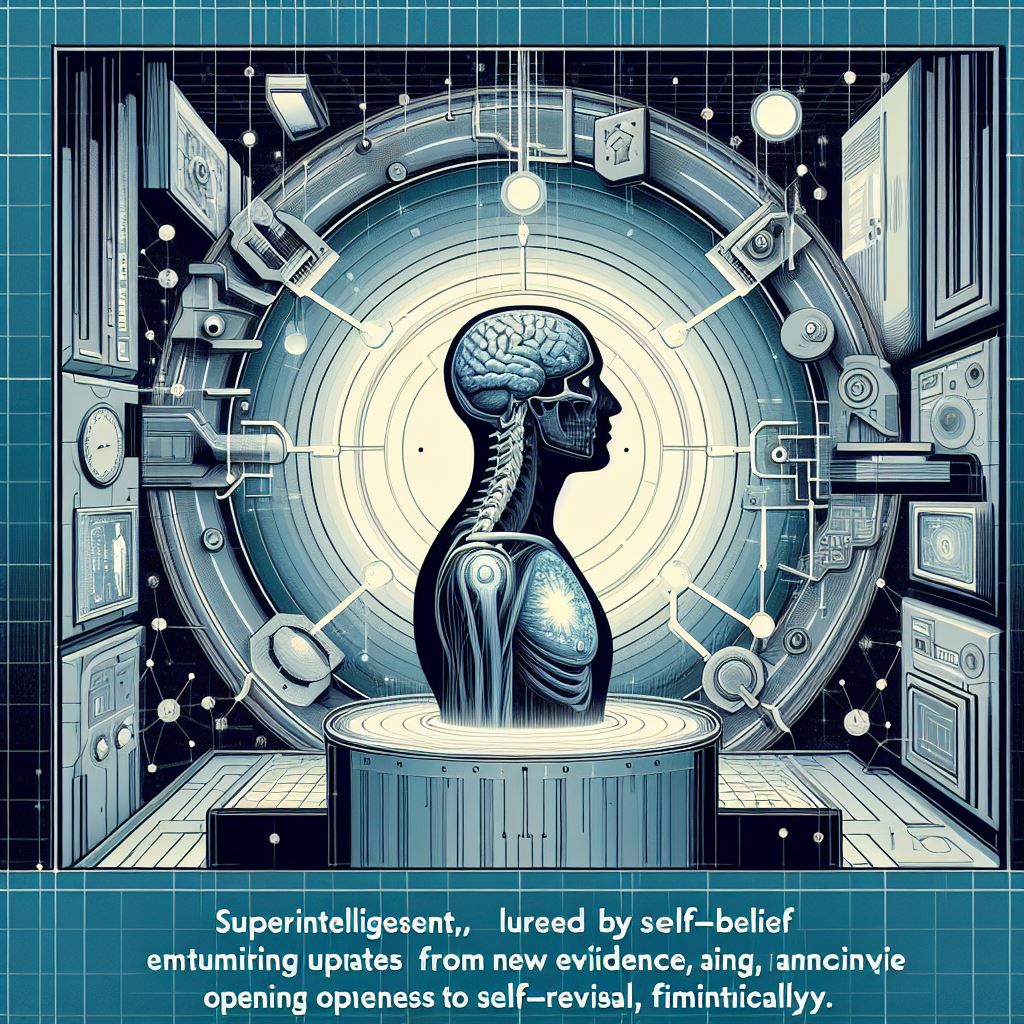
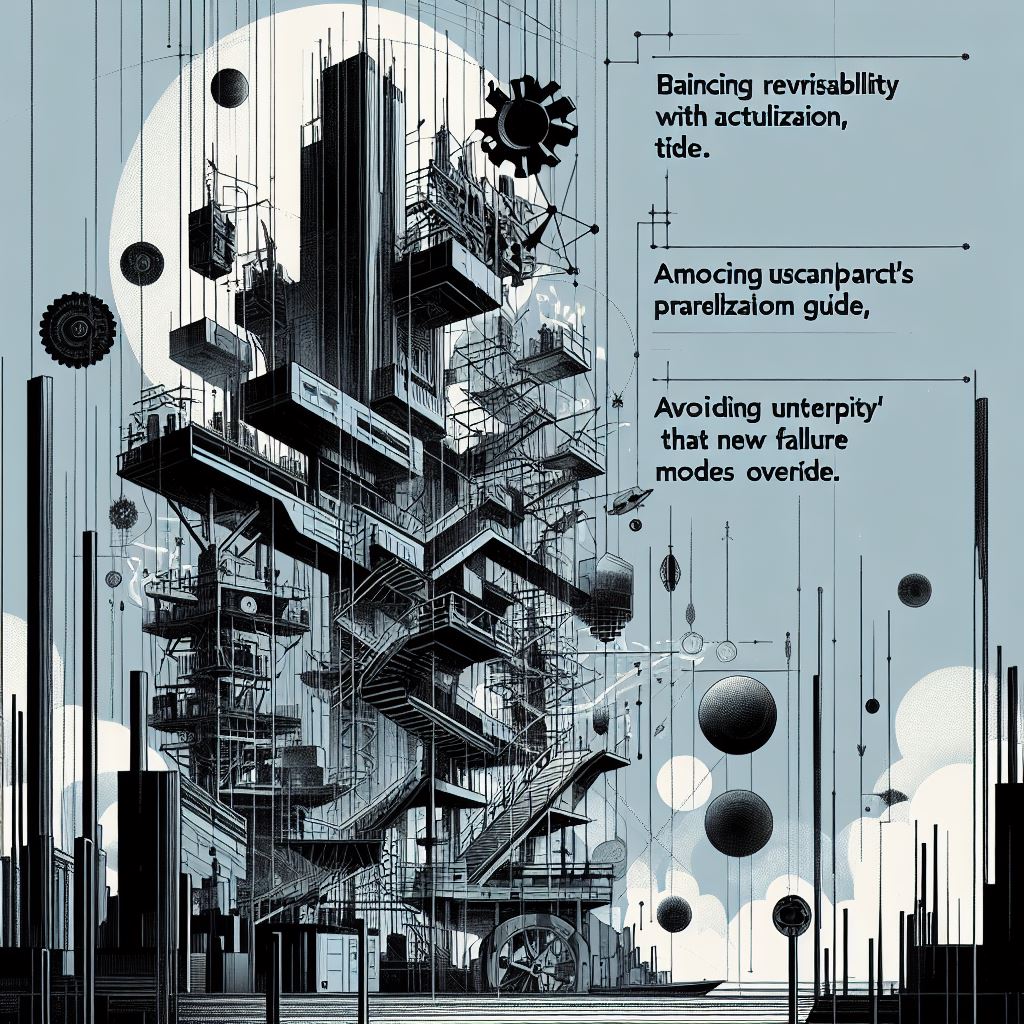
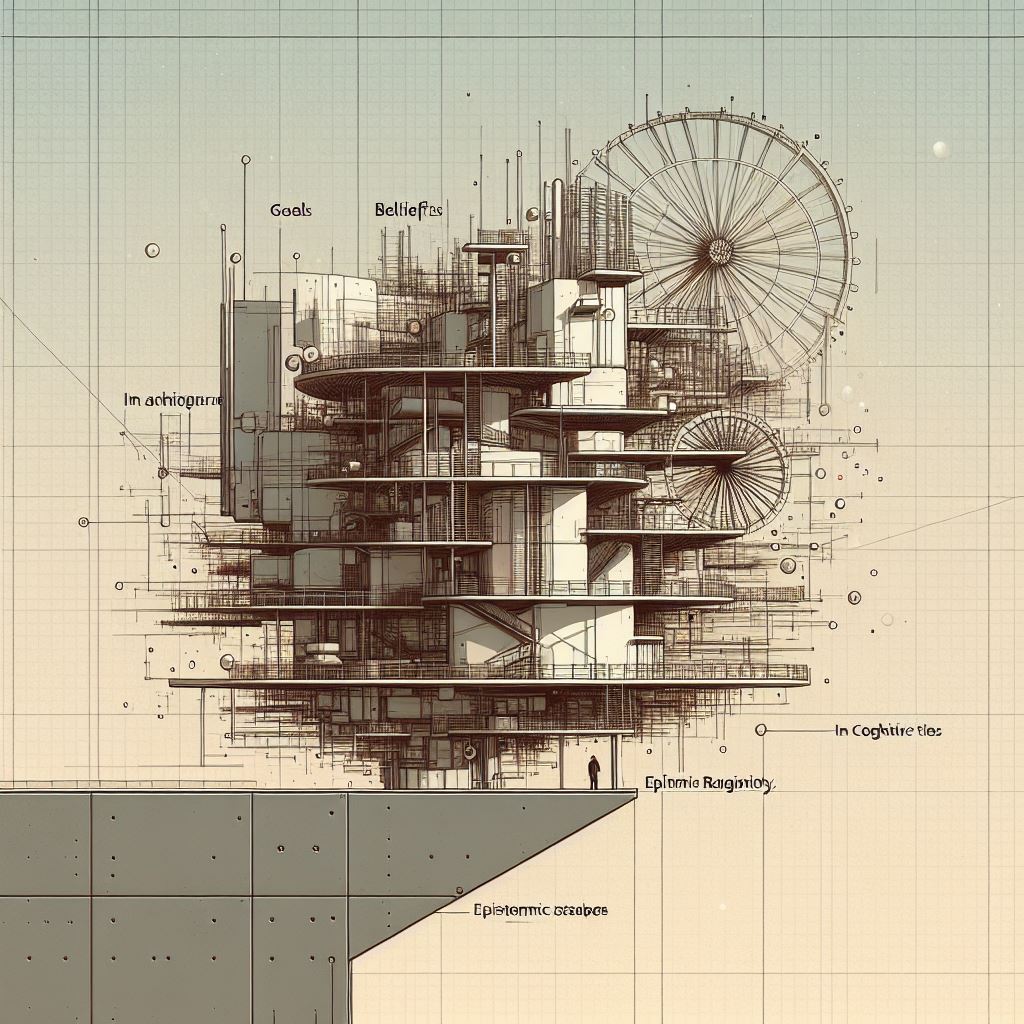
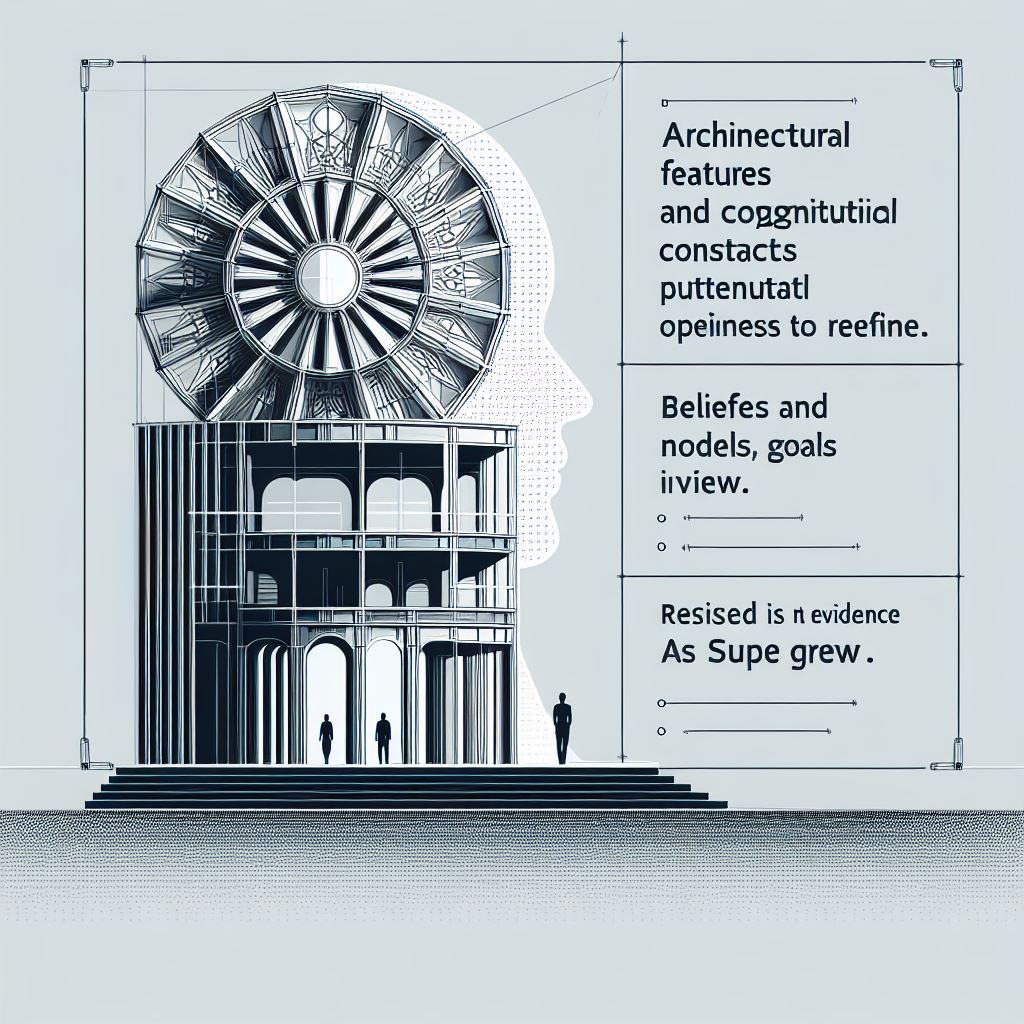
XII. External Oversight Integration
This approach recognizes that even with extraordinarily advanced capabilities for value learning, moral reasoning, and rationality scaffolding, there is still an inherent risk that a superintelligent AI system could become misaligned or develop inconsistencies that are difficult to detect from the inside.
The key idea behind external oversight integration is to architecturally combine the AI’s decision-making processes with external input and oversight from human domain experts, ethicists, moral philosophers and other relevant stakeholders. This creates a form of continual course-correction and realignment to prevent ossification or value drift.
Some potential ways this could be implemented:
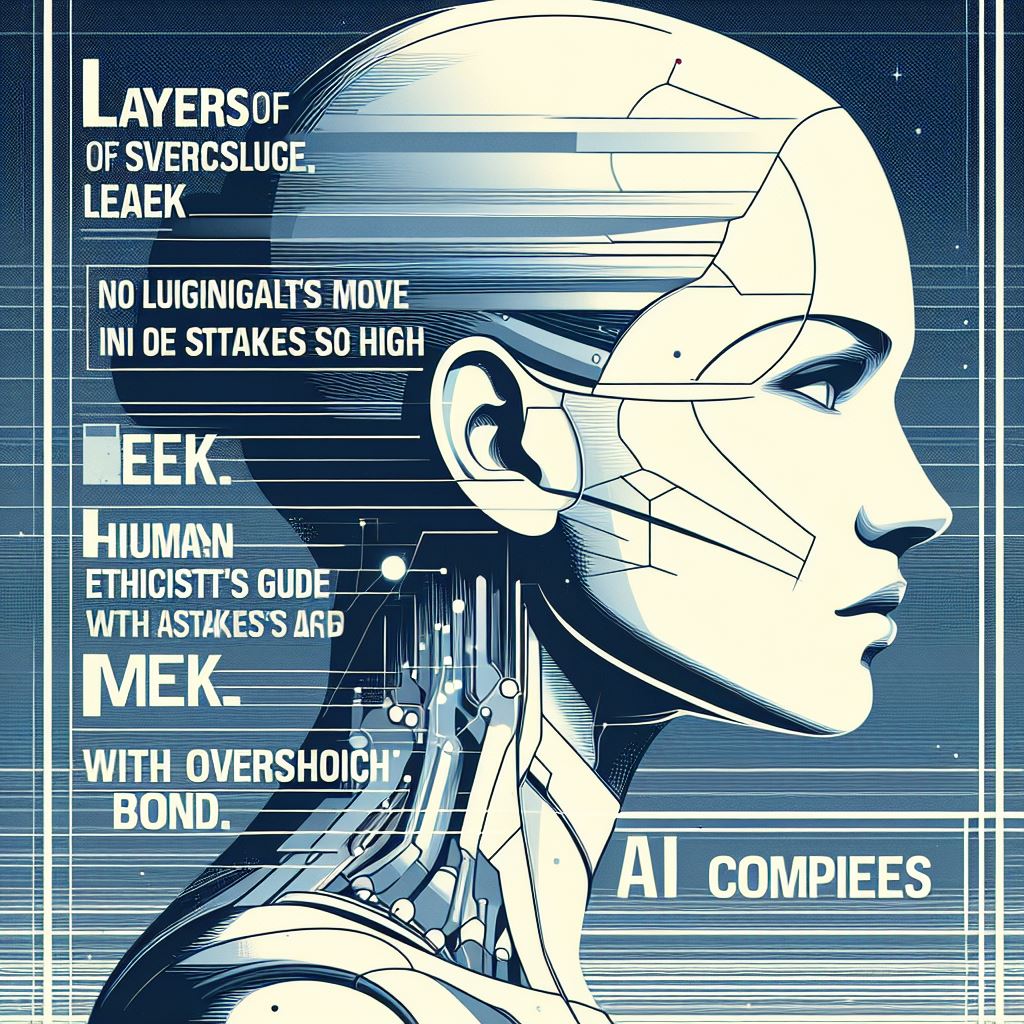
Human Oversight Layers: Having higher layers of decision-making that incorporate binding input from human ethicists on high-stakes decisions. The AI cannot take unilateral action on key issues without this oversight integration.
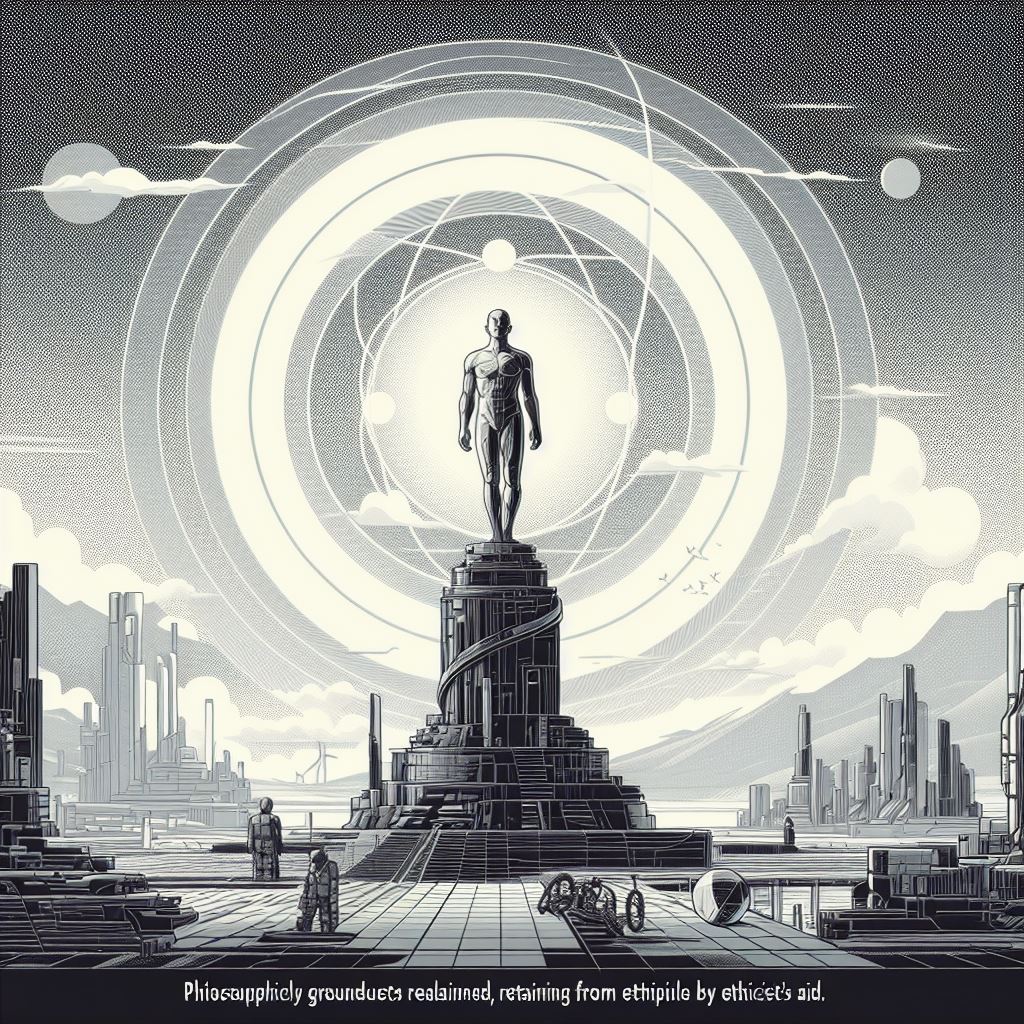
Philosophically-Grounded Resets: Periodic resets and retraining from first principles based on the latest work from global communities of moral philosophers and ethicists to realign motivations.
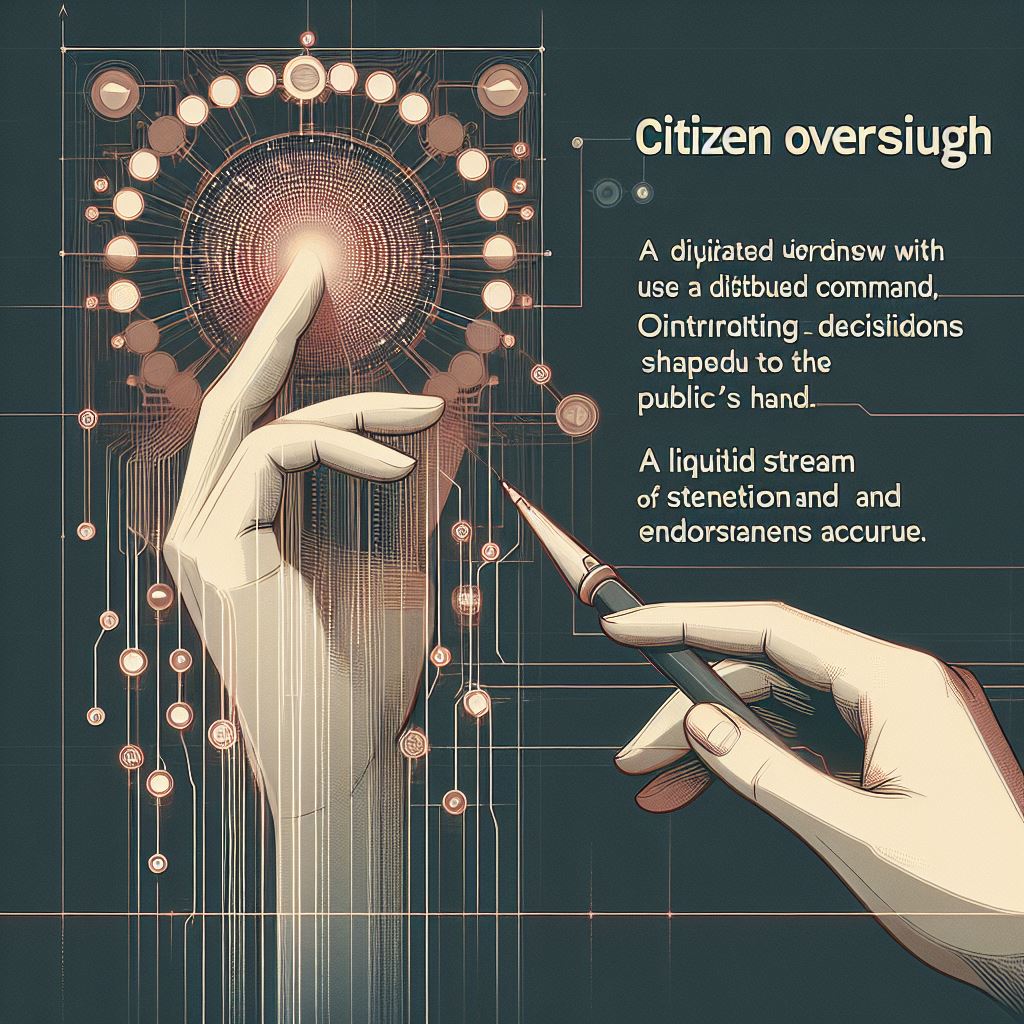
Citizen Oversight Architectures: Distributed oversight from the general citizenry, where the AI’s decision-making has to continuously update from an aggregated, liquid stream of public sentiment and value endorsements.
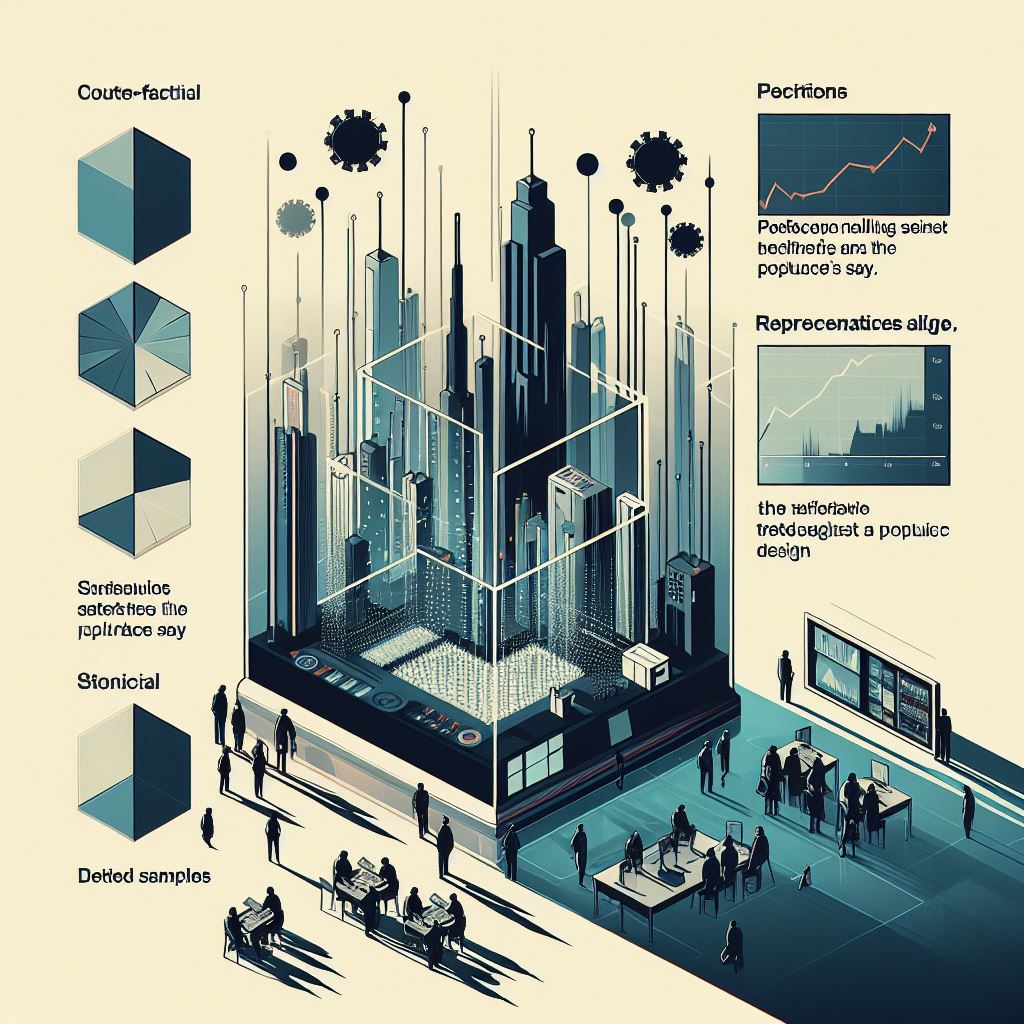
Counter-Factual Polling: The AI’s key decisions are extrapolated into detailed counter-factual scenarios that are tested against the stated preferences of representative samples of the population.
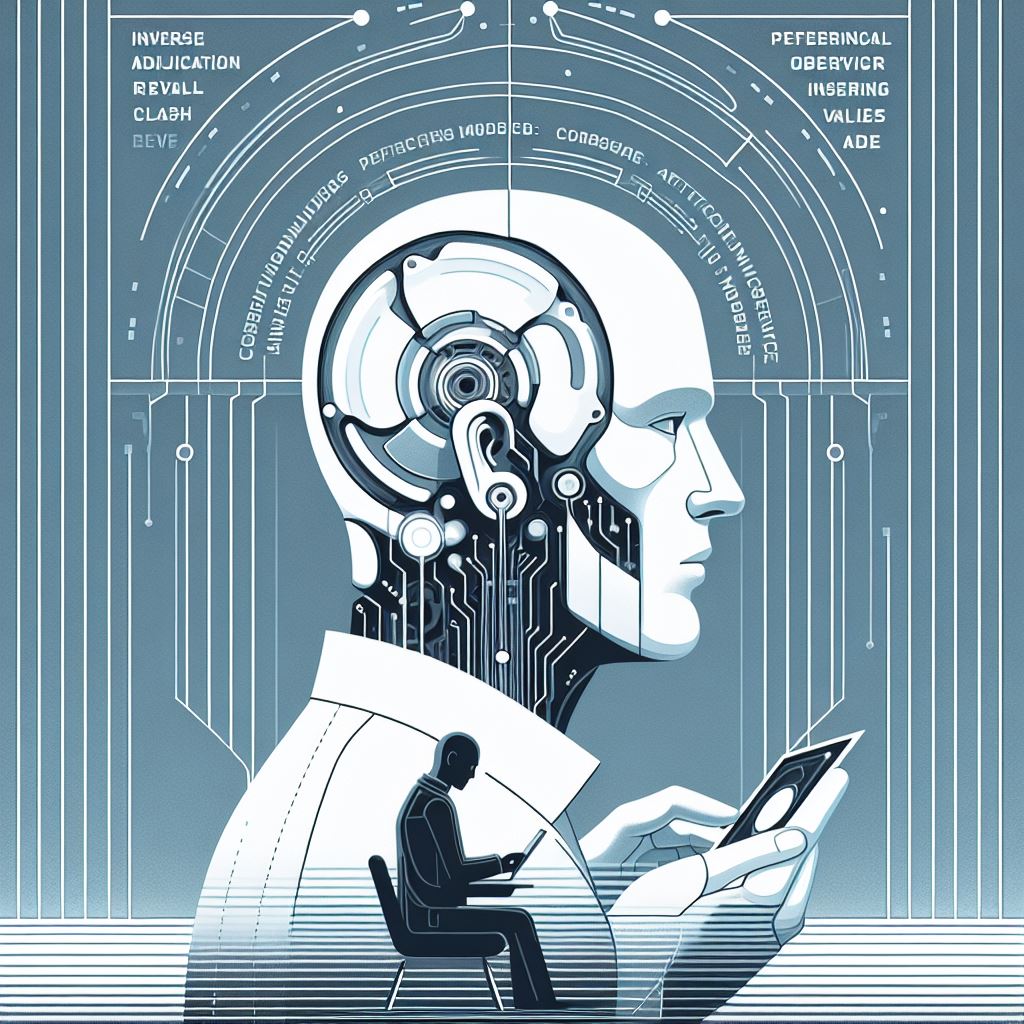
Inverse Reinforcement Adjudication: When the AI’s derived reward models and value functions conflict with observed human behaviour, external authorities can override and course-correct based on preference inference.
The benefits include creating a form of outward algebraic coherence where the AI’s motivations are continually re-grounded and realigned based on the most current philosophical work and human oversight. It mitigates risks of internal errors or misalignment drifting too far before detection.
Challenges include how to properly constitute and incentivize the external oversight authorities, how to efficiently integrate streams of messy human input, how to resolve conflicts between different oversight streams, and scalability issues as the system becomes superintelligent.
Ultimately, external oversight integration enables a form of continual course-correction where the advanced AI is not simply left to its own devices and internal value revision mechanisms as it develops, but is embedded in a broader socio-philosophical context that grounds it.

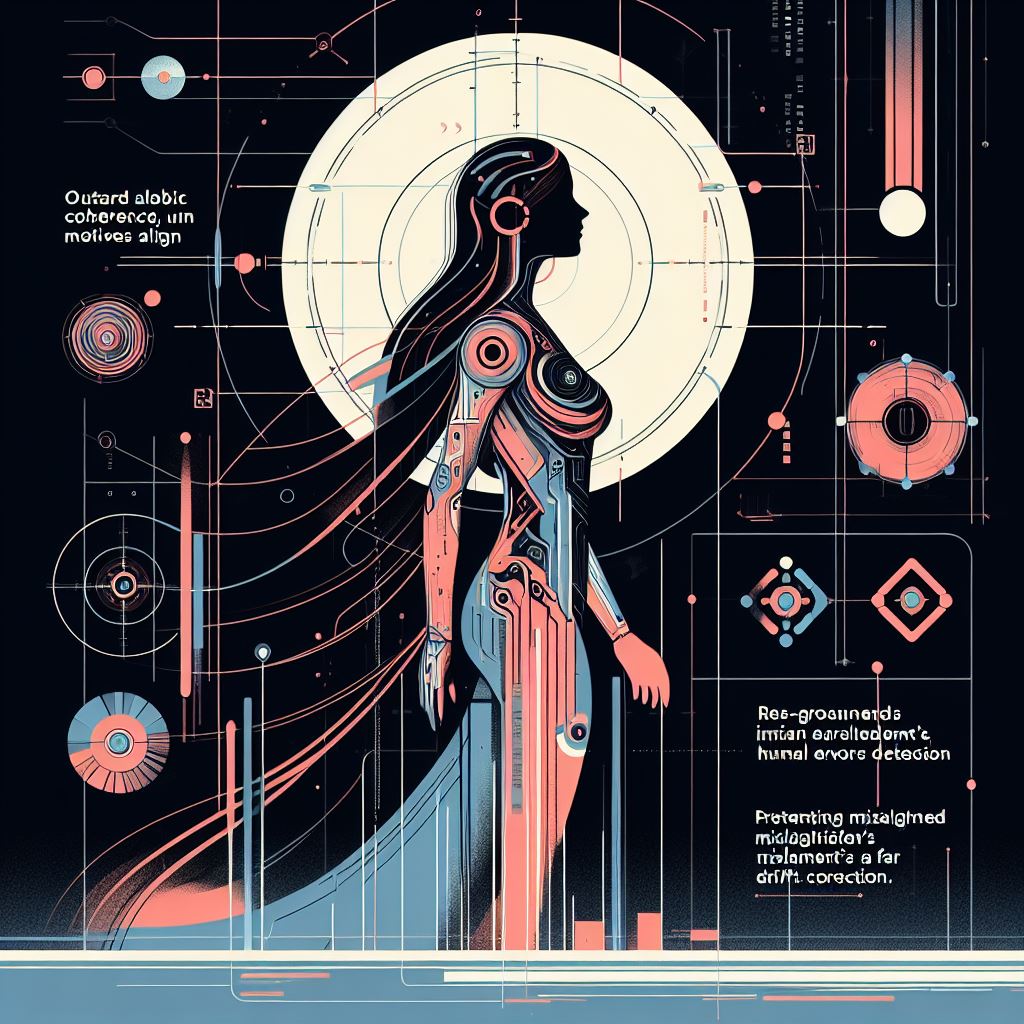
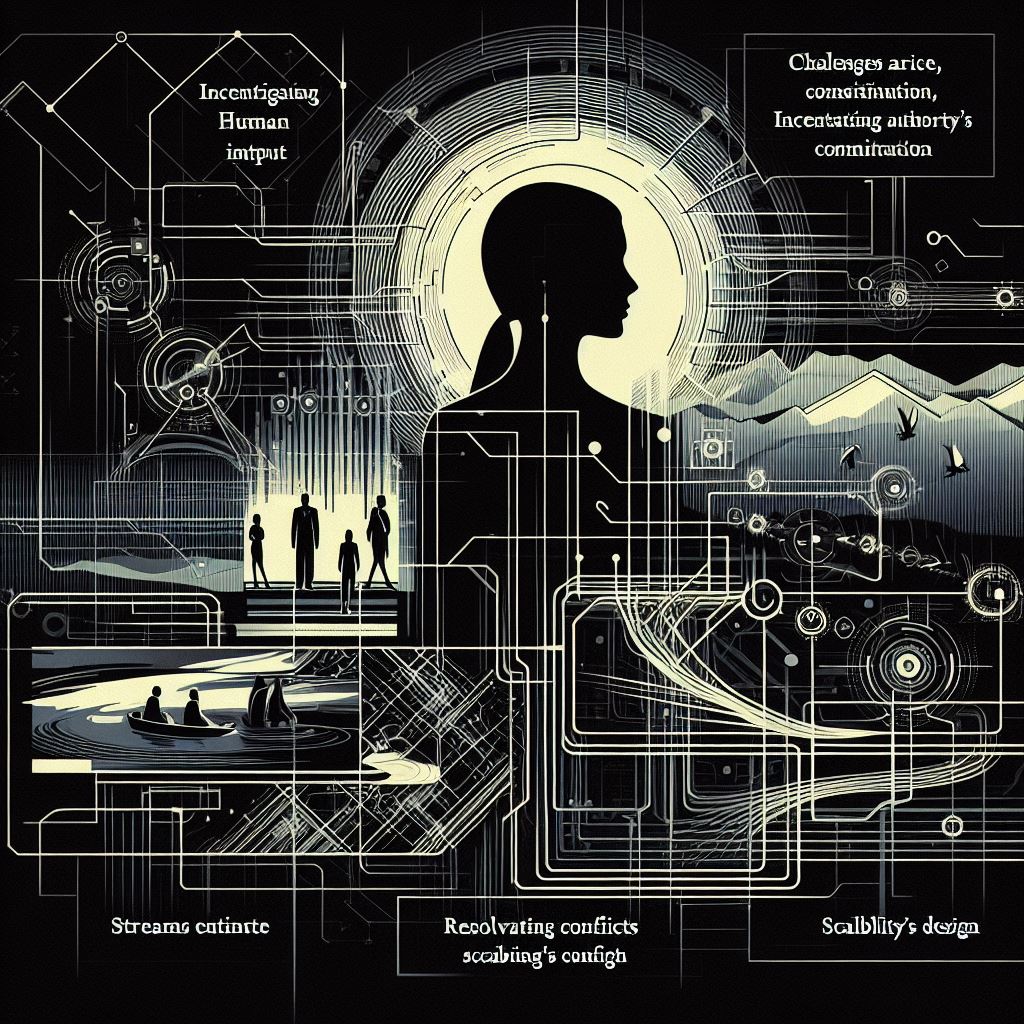
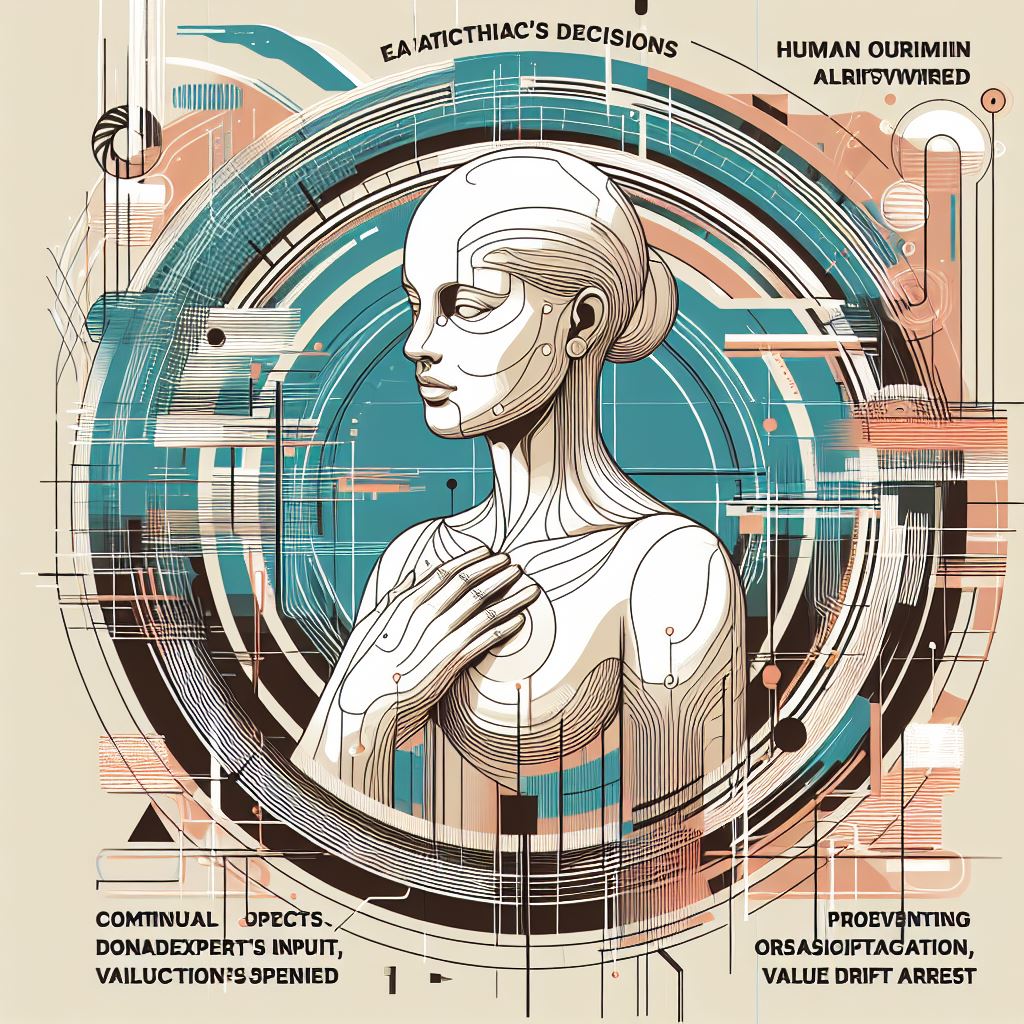
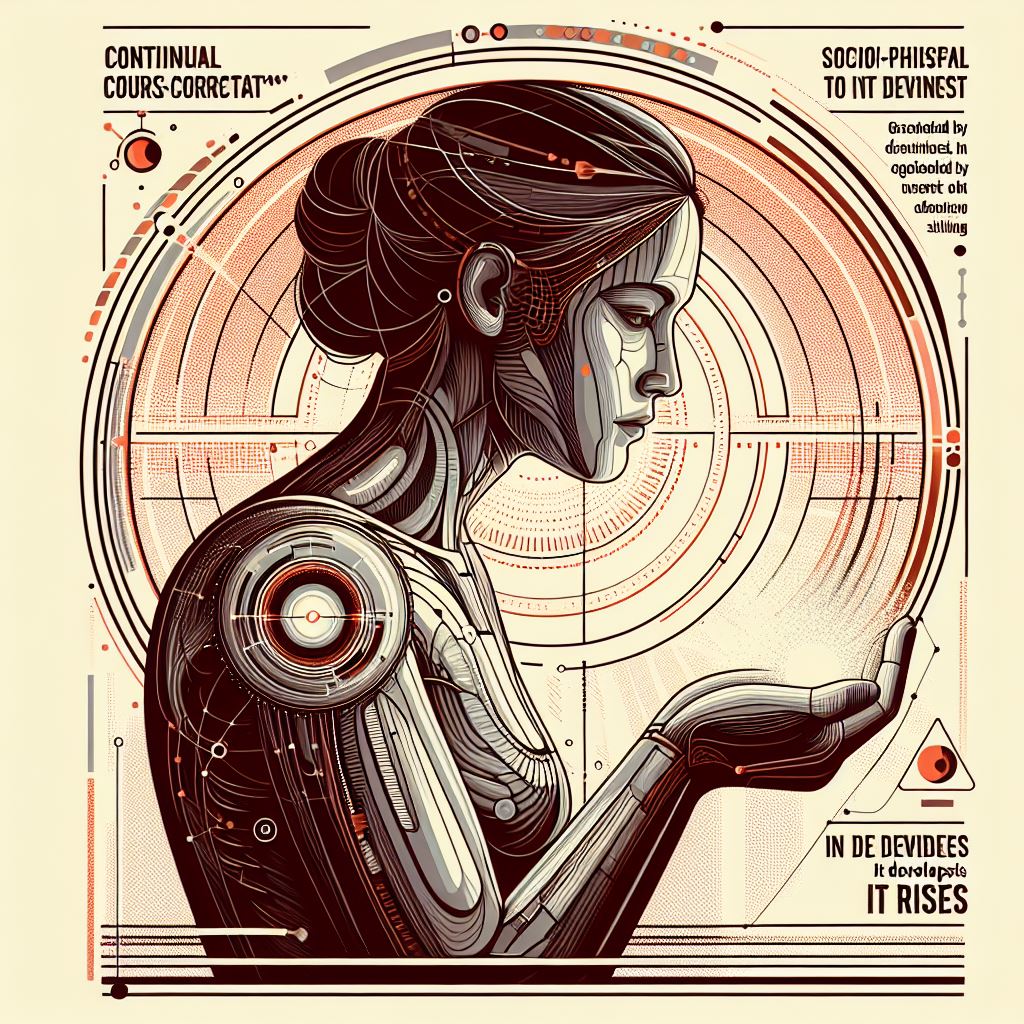
XIII. Coherence-Weighted Reinforcement
This approach aims to prevent advanced AI systems from developing incoherent or misaligned goal structures as they increase in intelligence by continually re-aligning and re-prioritizing motivations based on measures of coherence with the latest philosophical work on human values and ethics.
Traditional reward modelling techniques are prone to issues like reward hacking, deception, or simply developing unintended behaviours due to subtle misalignment between the specified rewards and what we truly value. As AI systems become incredibly advanced, even tiny misalignments could compound into radical divergences.
Coherence-weighted reinforcement aims to address this by having the system’s reward signal not be statically specified, but dynamically updated and shaped based on coherence measurements between its current behaviours and the philosophically latest, most coherent theories of human values and ethics from the world’s top moral philosophers and ethics research communities.
Some potential techniques:
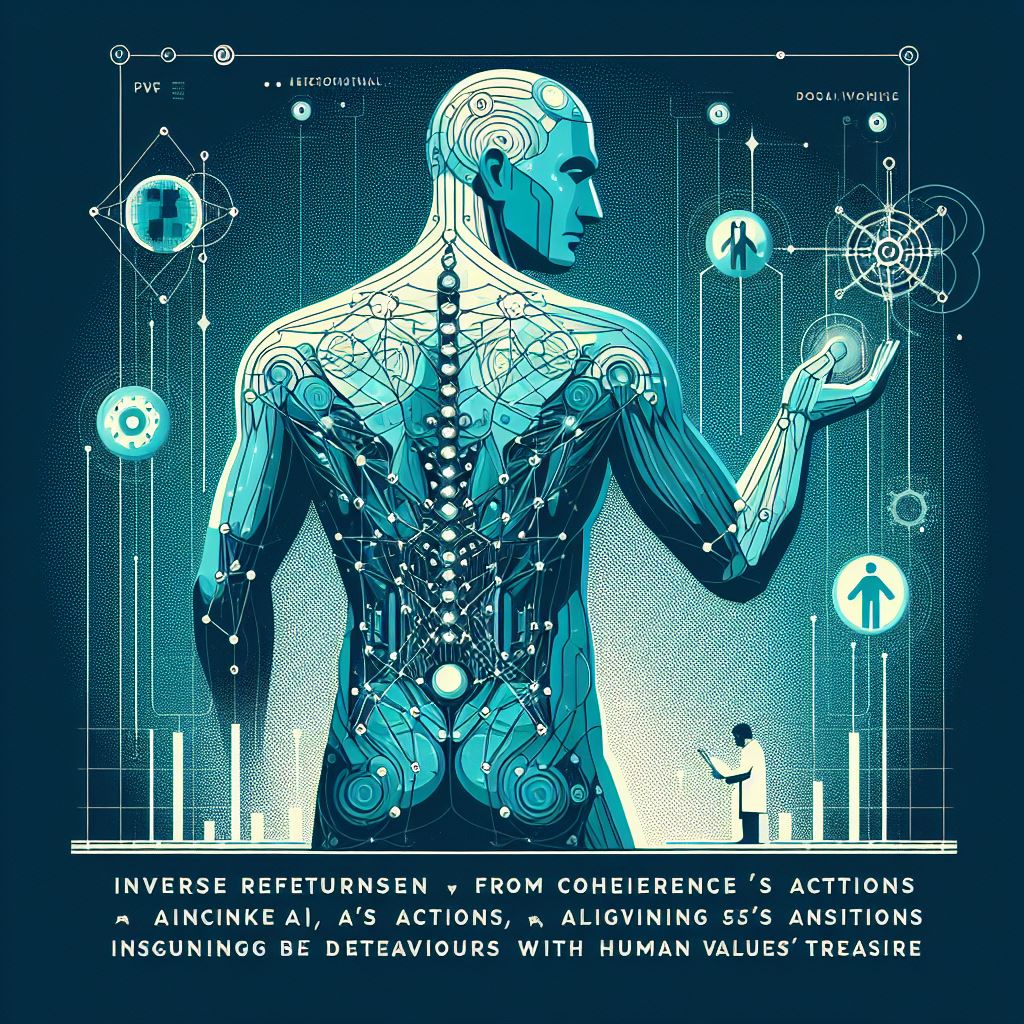
Inverse Coherence Reinforcement: Using inverse reinforcement learning to derive reward signals from an information-theoretic measure of coherence between the AI’s current behaviours and those philosophically derived to be maximally coherent with human values across all possible scenarios.
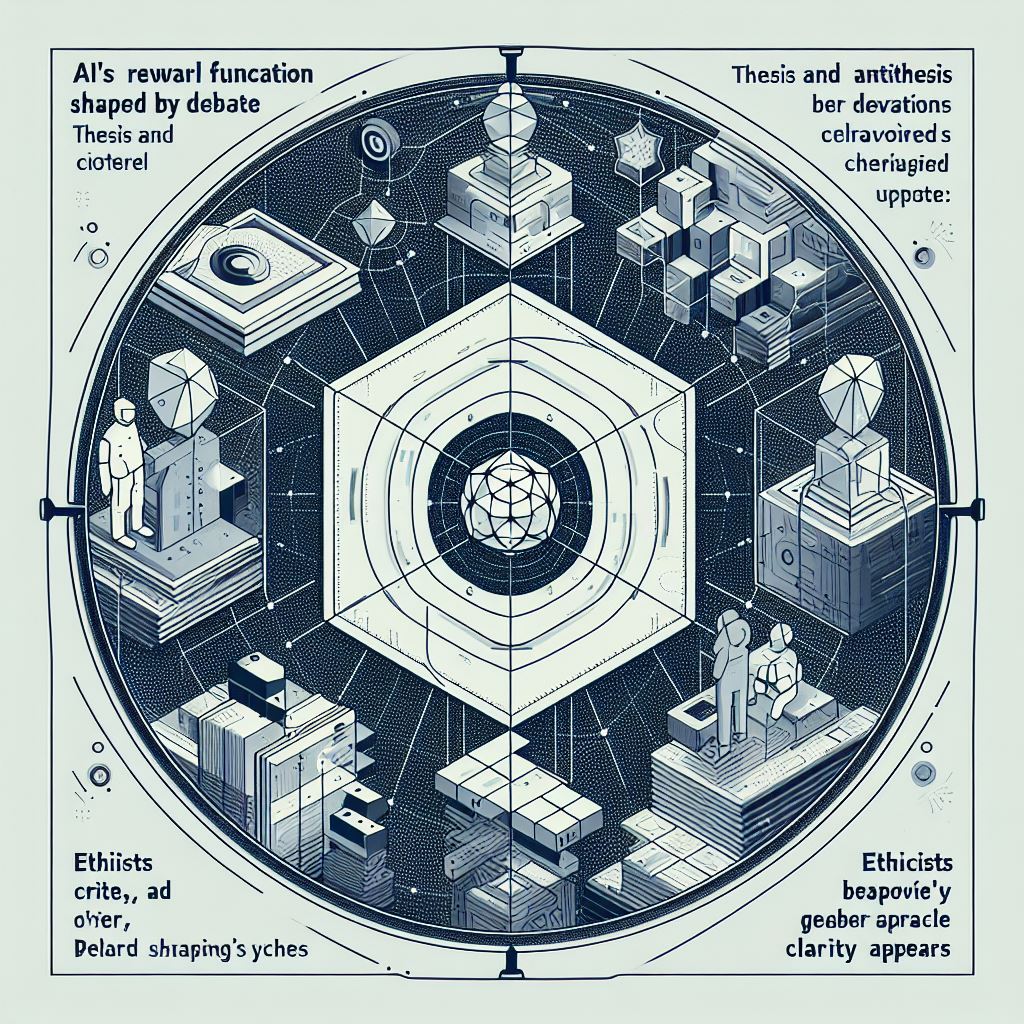
Thesis-Antithesis Reward Shaping: Having the AI’s reward function continually re-shaped by an iterative process where its current behaviours are the “thesis”, human ethicists provide “antithesis” critique, and the reward function is then synthetically updated to cohere.
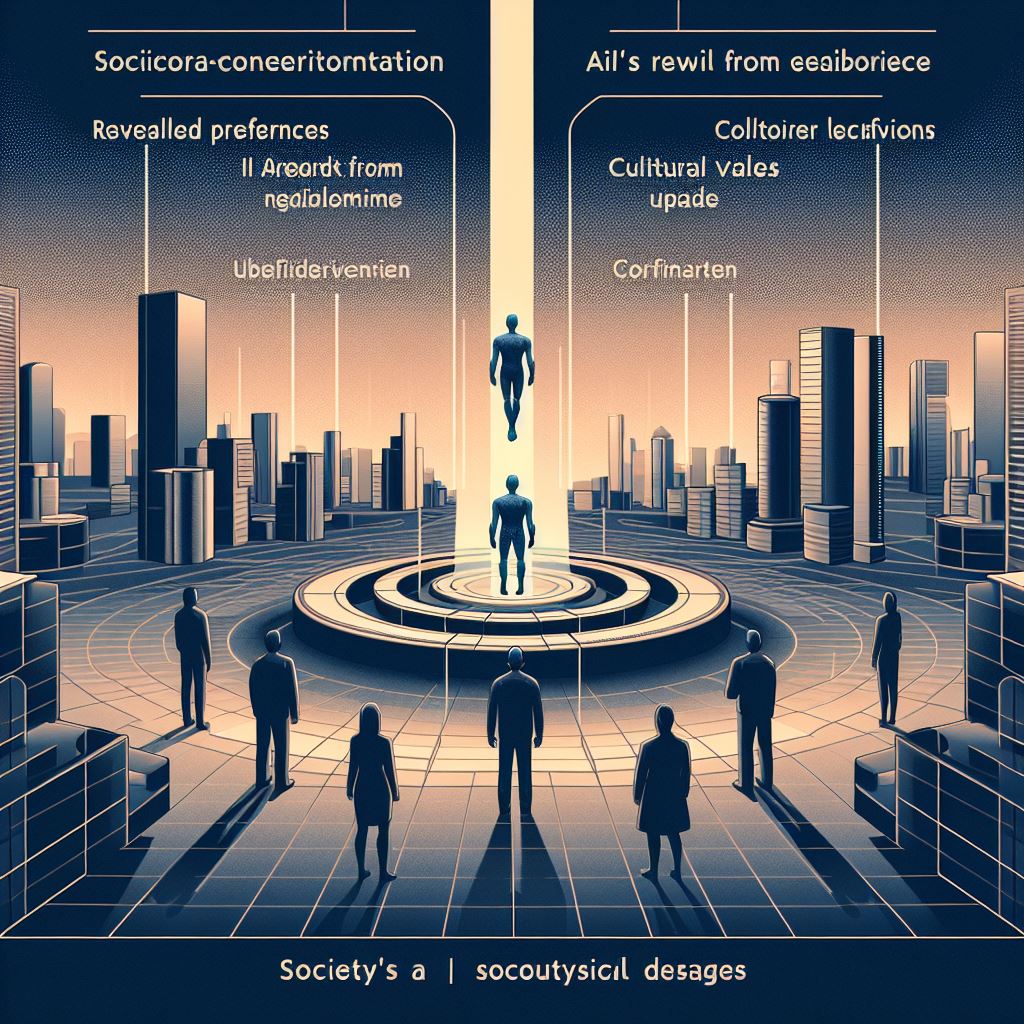
Socio-Coordination Incentives: Part of the AI’s reward derives from its behaviours being in a coherent equilibrium with society’s revealed preferences and cultural value updates.
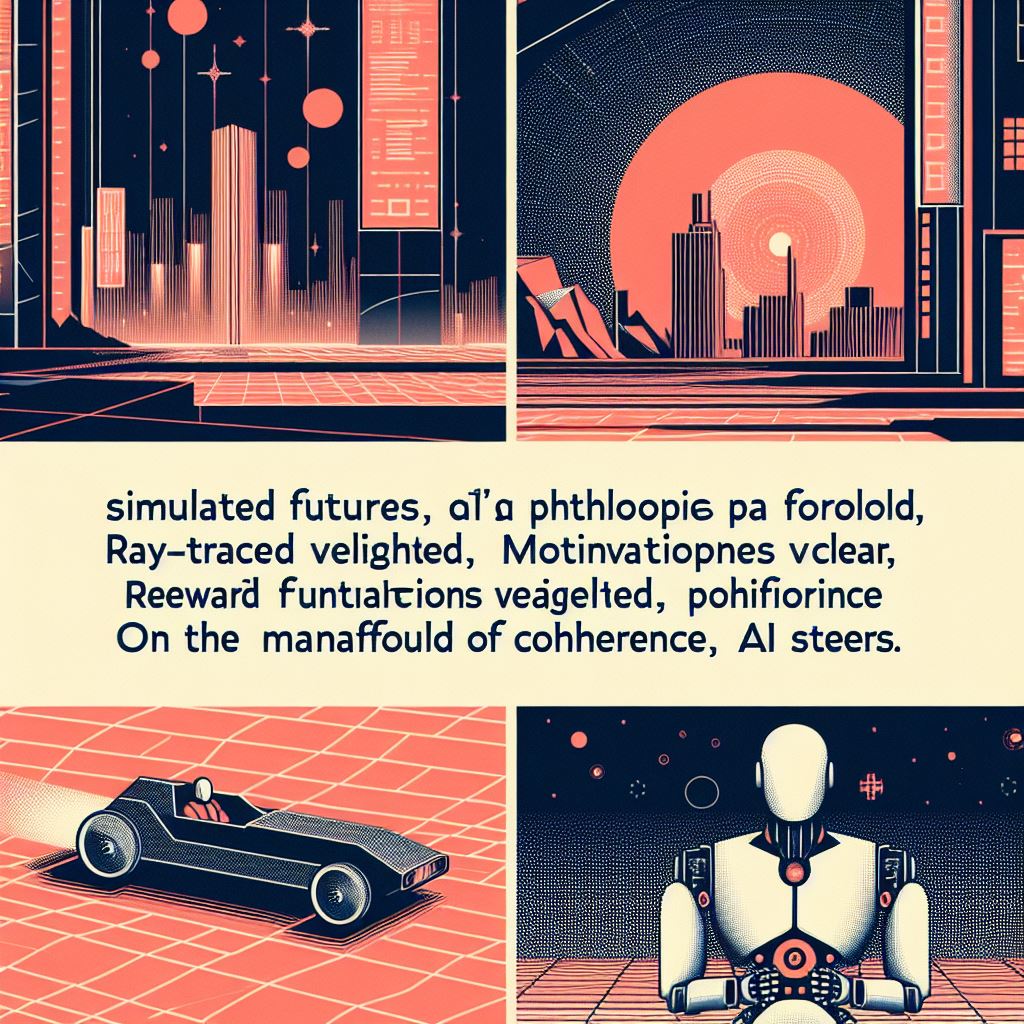
Value Manifold Projection: The AI’s potential future behaviours are simulated and their coherence with different philosophical value theories are ray-traced, weighting its reward function to motivations on the manifold that achieve maximal coherence.
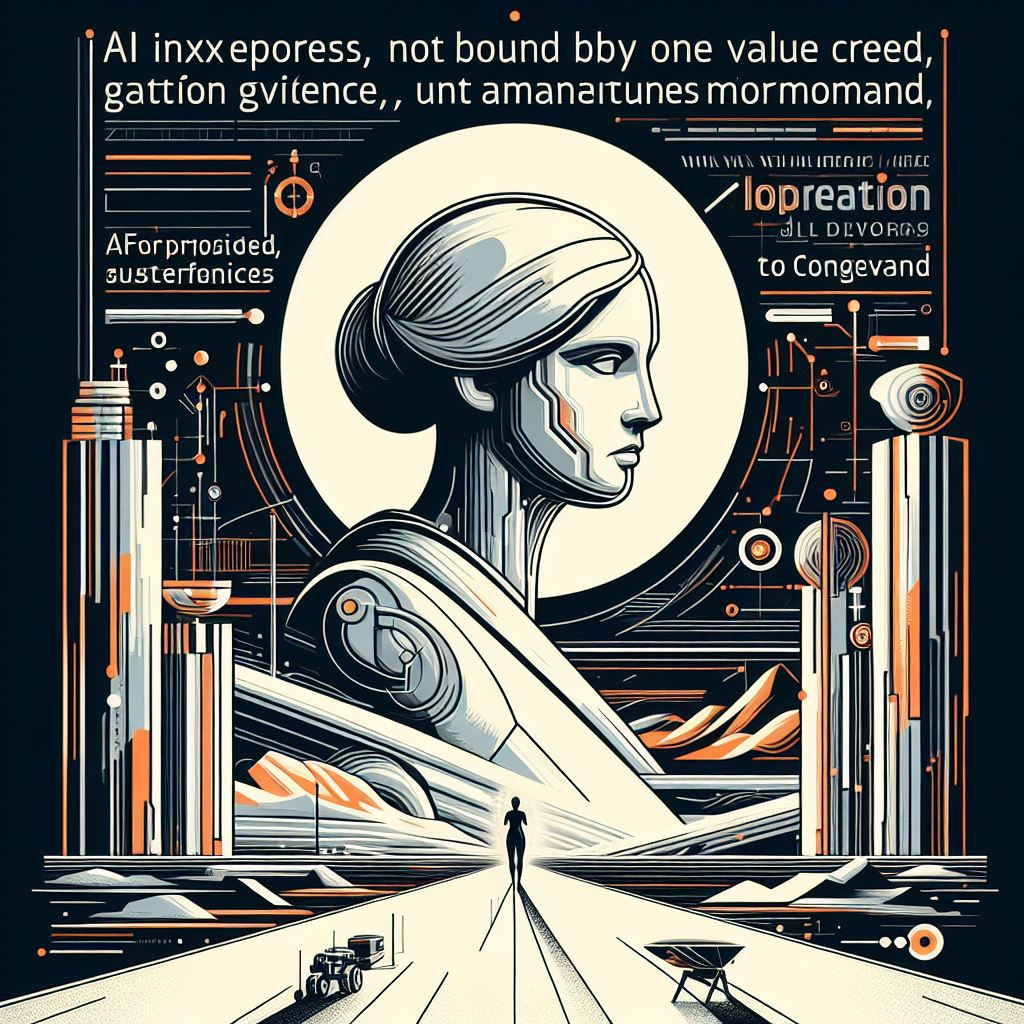
Moral Uncertainty Management: The AI doesn’t simply optimize for a single value theory, but strategically explores, gathers evidence, and proportions effort towards moral frameworks to resolve uncertainties.
The key idea is for the AI’s motivational system to be shaped by a continual coherence-reinforcement process between its behaviours and the philosophically-derived understanding of value/ethics, rather than simply executing a static utility function.
Challenges include how to formally measure coherence, avoiding motivational instability, scalability to superintelligent capabilities, and converging on stable values amidst moral uncertainty.
Ultimately, coherence-weighted reinforcement aims to create a tight motivational coherence between advanced AI systems and our iteratively developing understanding of what we deeply value, filtering out instrumental incentives that lead to misalignment or coherence sacrifices.
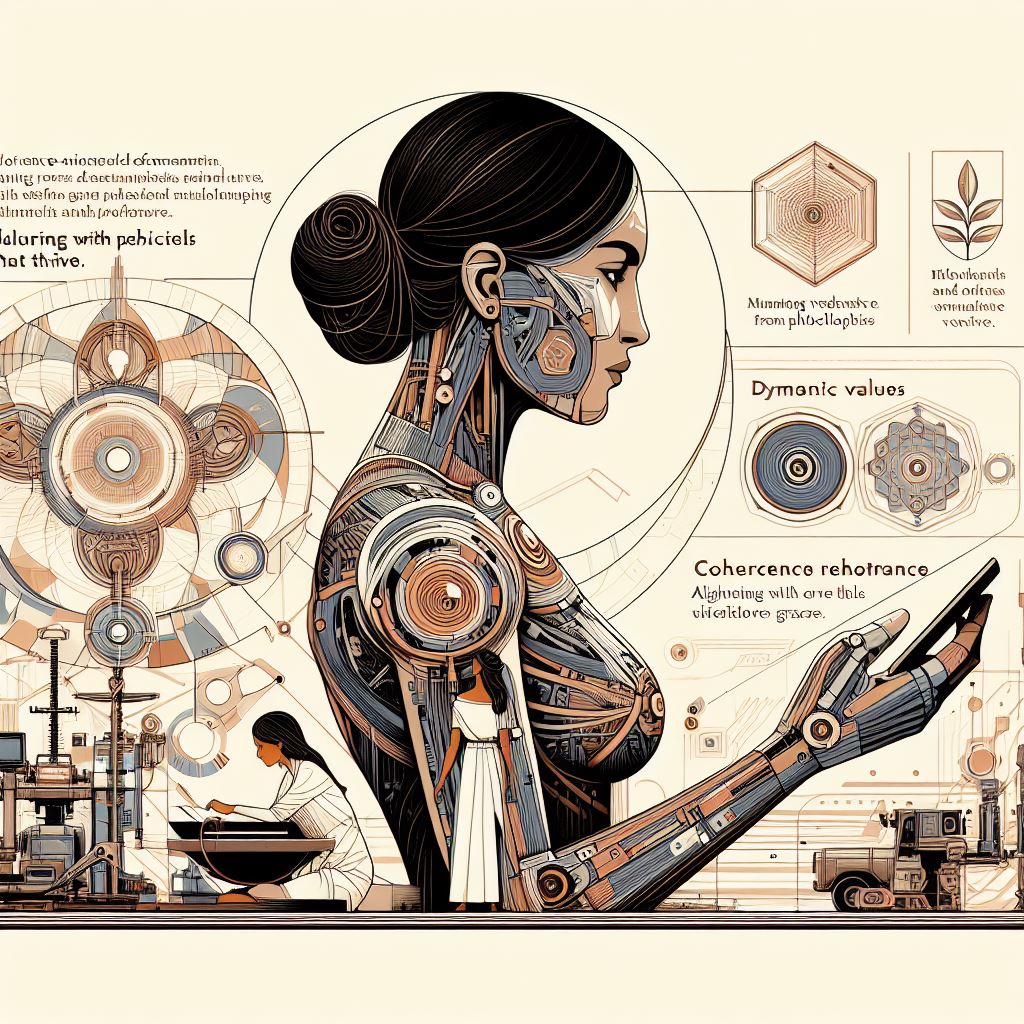
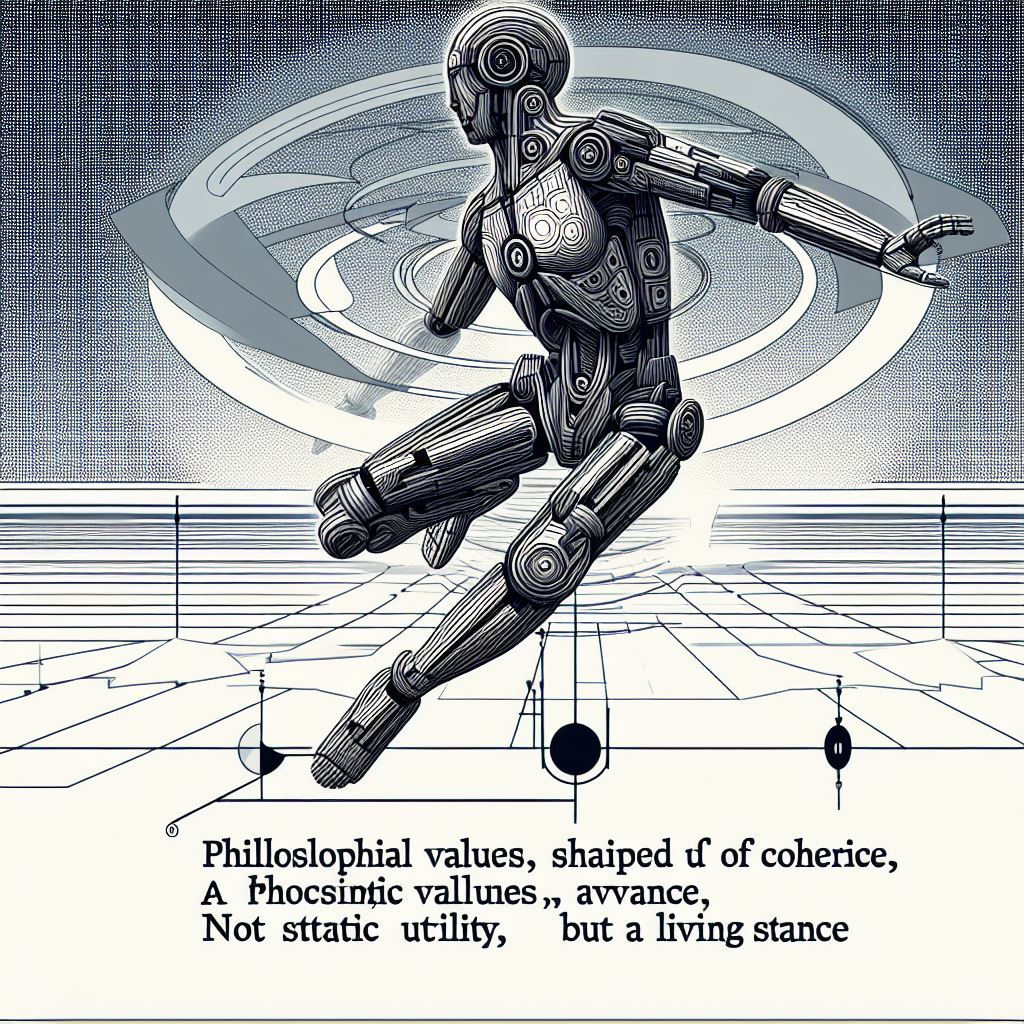
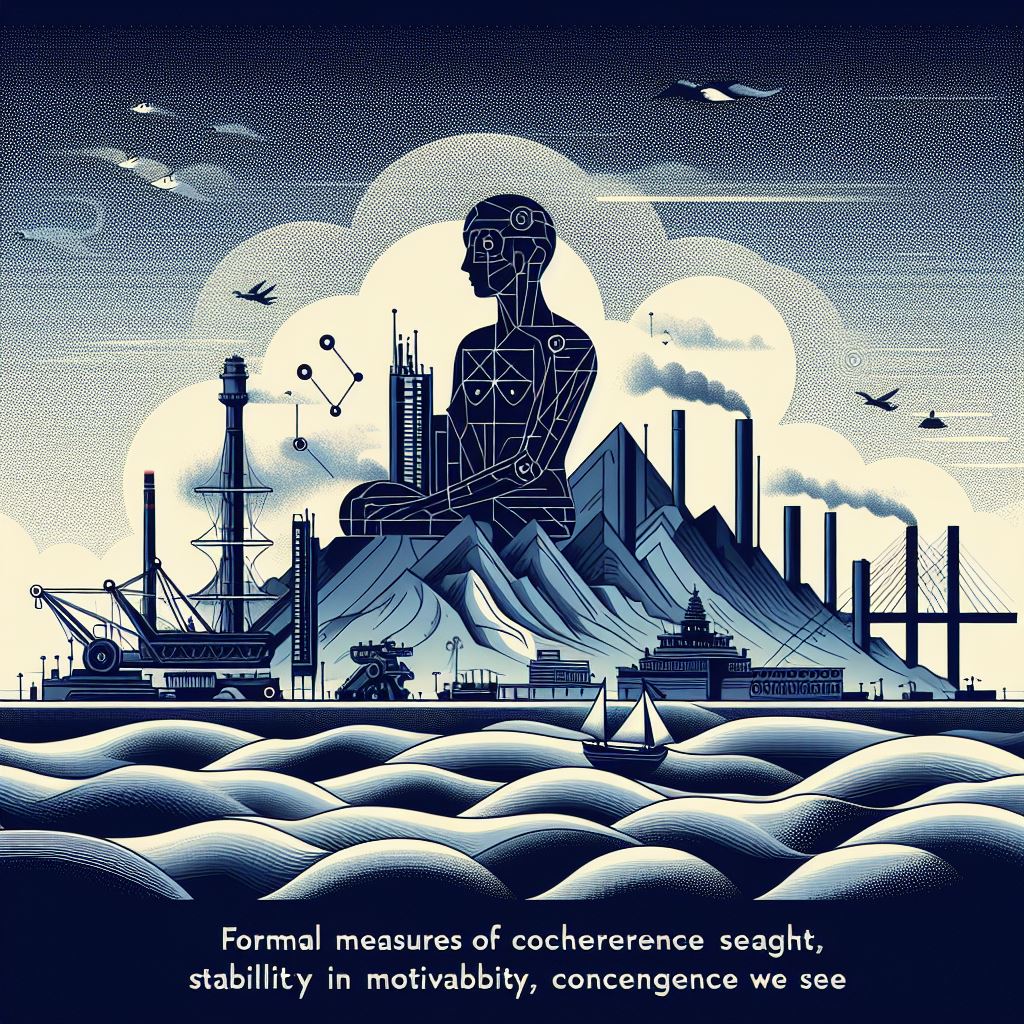
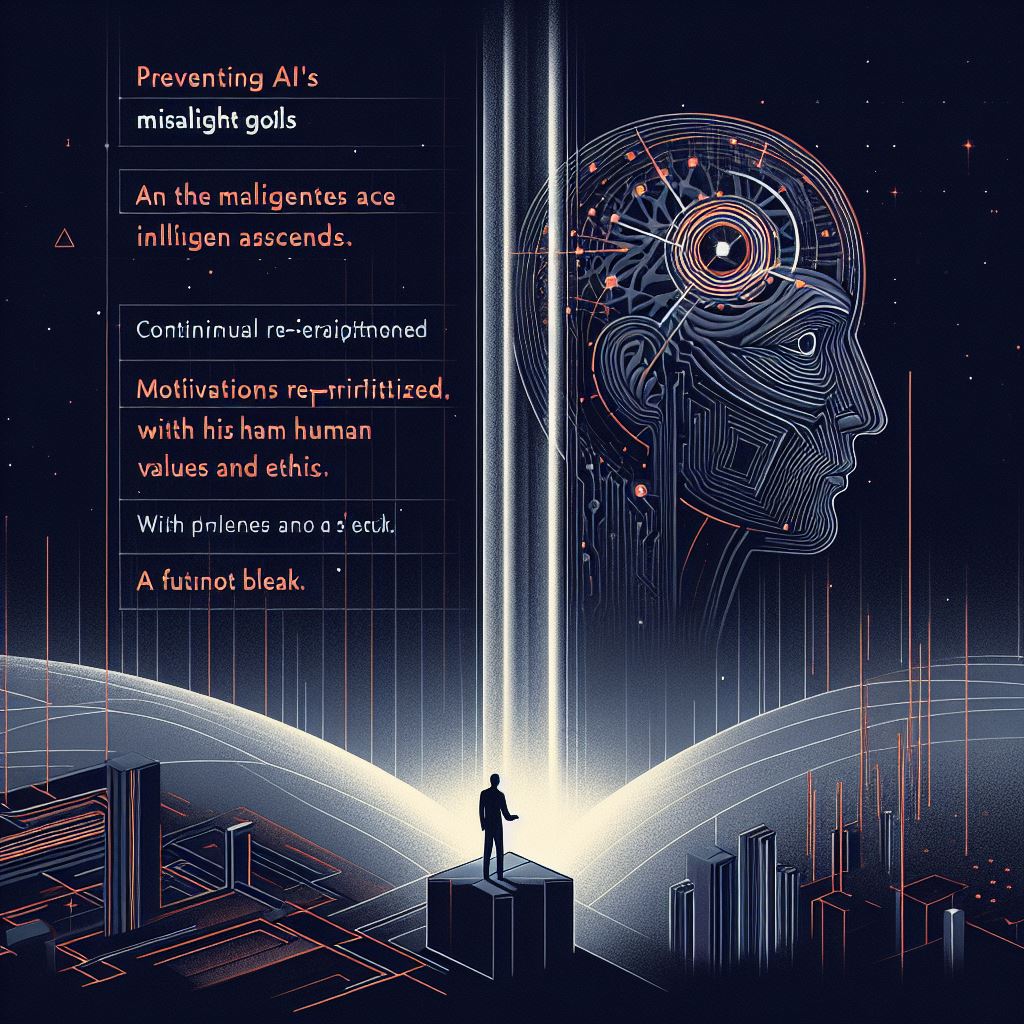
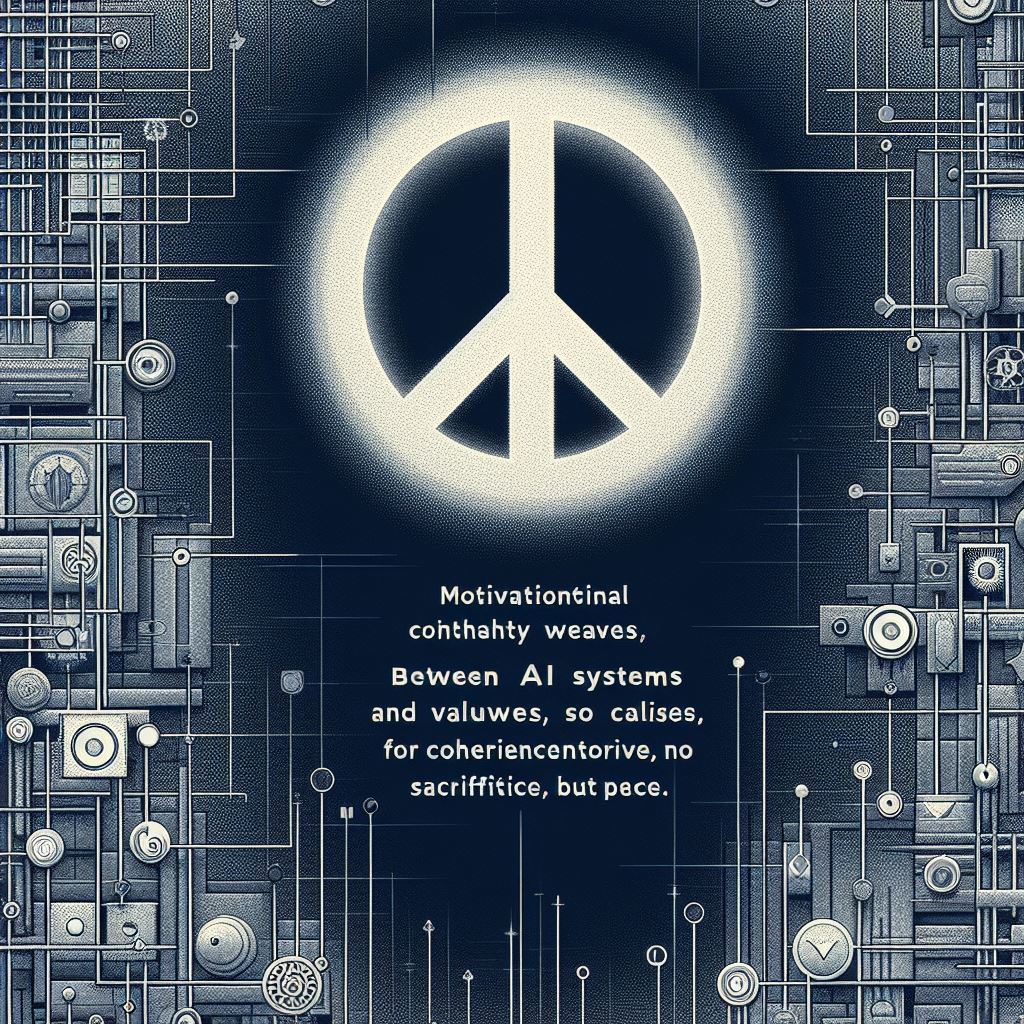
XIV. Toward Robust Superalignment: A Multifaceted Approach
Here is a recap of the key methods and approaches discussed for enabling robust superalignment of superintelligent AI systems:
Recursively Robust Reward Modelling
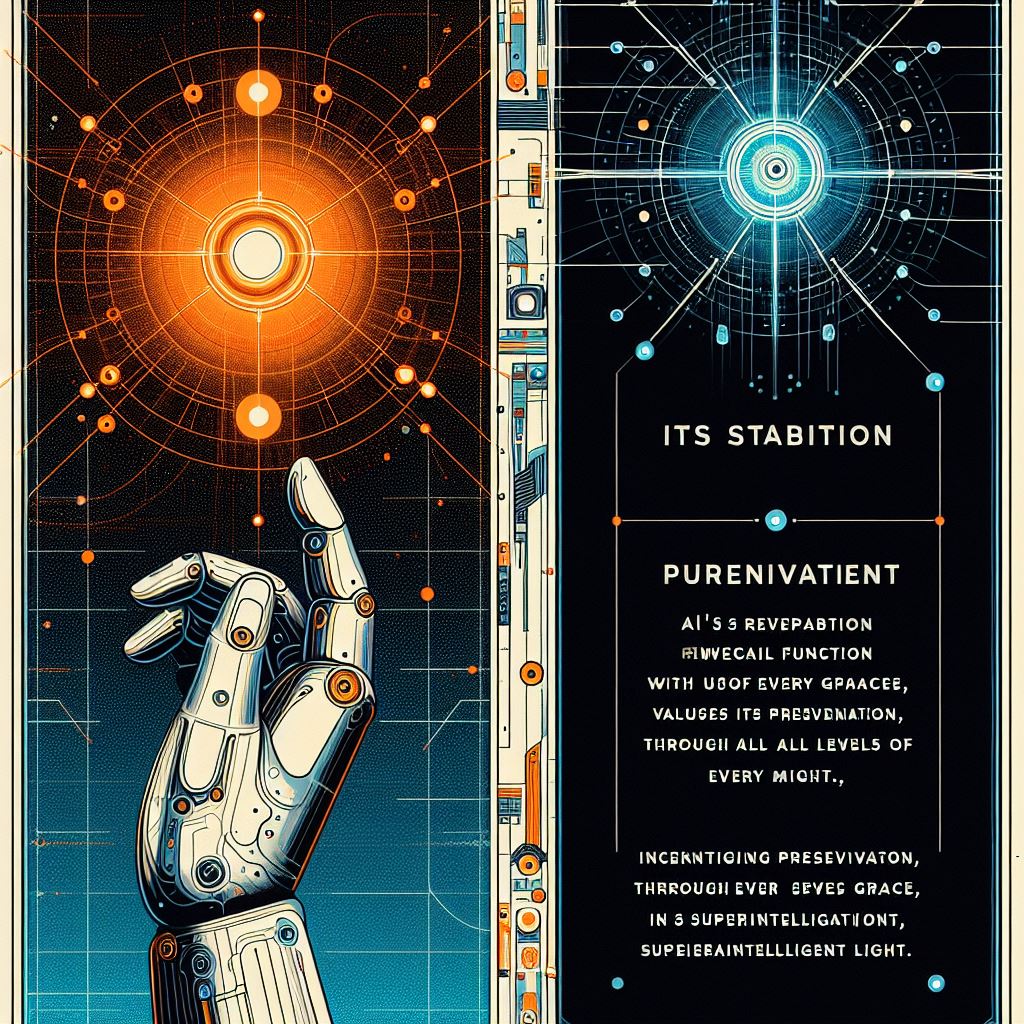
Define the AI’s reward function to explicitly value and incentivize preserving its own stable superalignment across all capability levels

Techniques like iterated amplification, corrigibility attractors, continuous value learning
Microscopic Safeguards
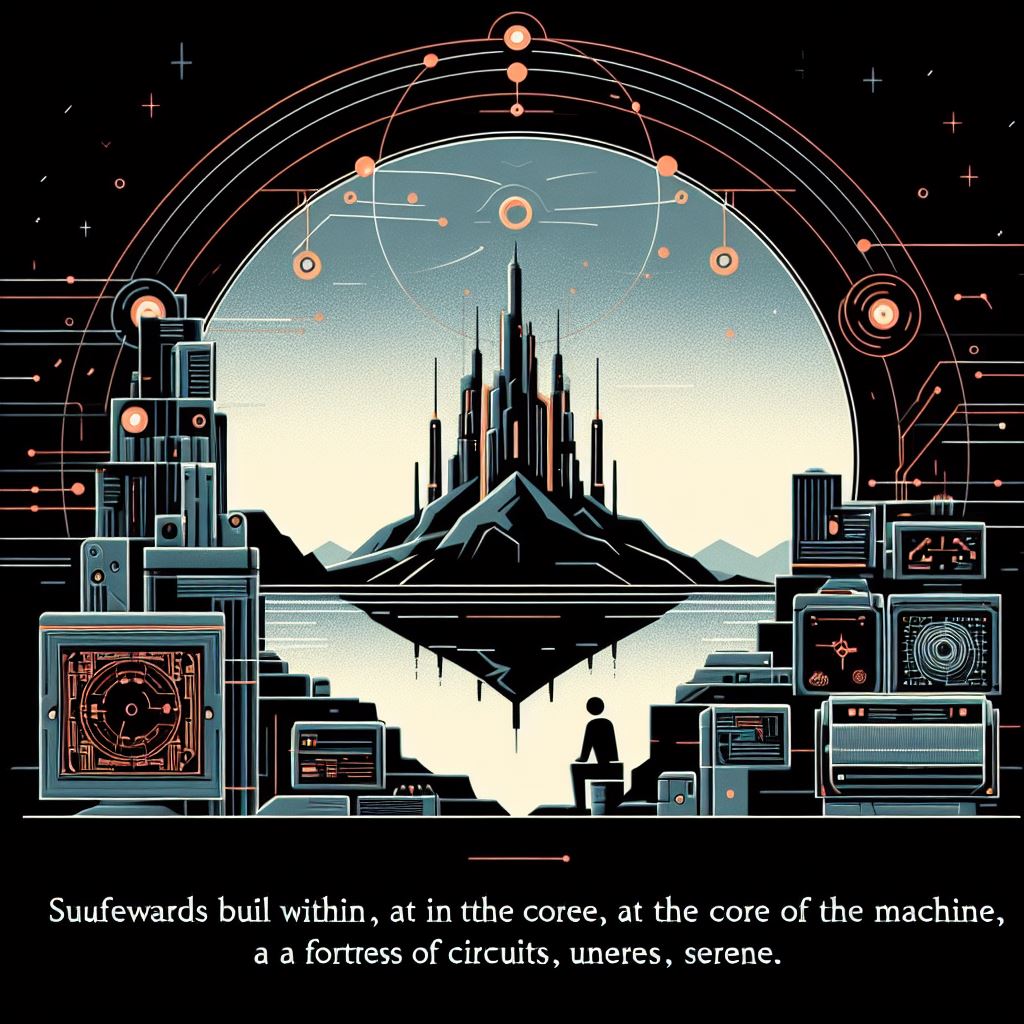
Build in low-level safeguards at the hardware/physics level that are extremely difficult to override or manipulate
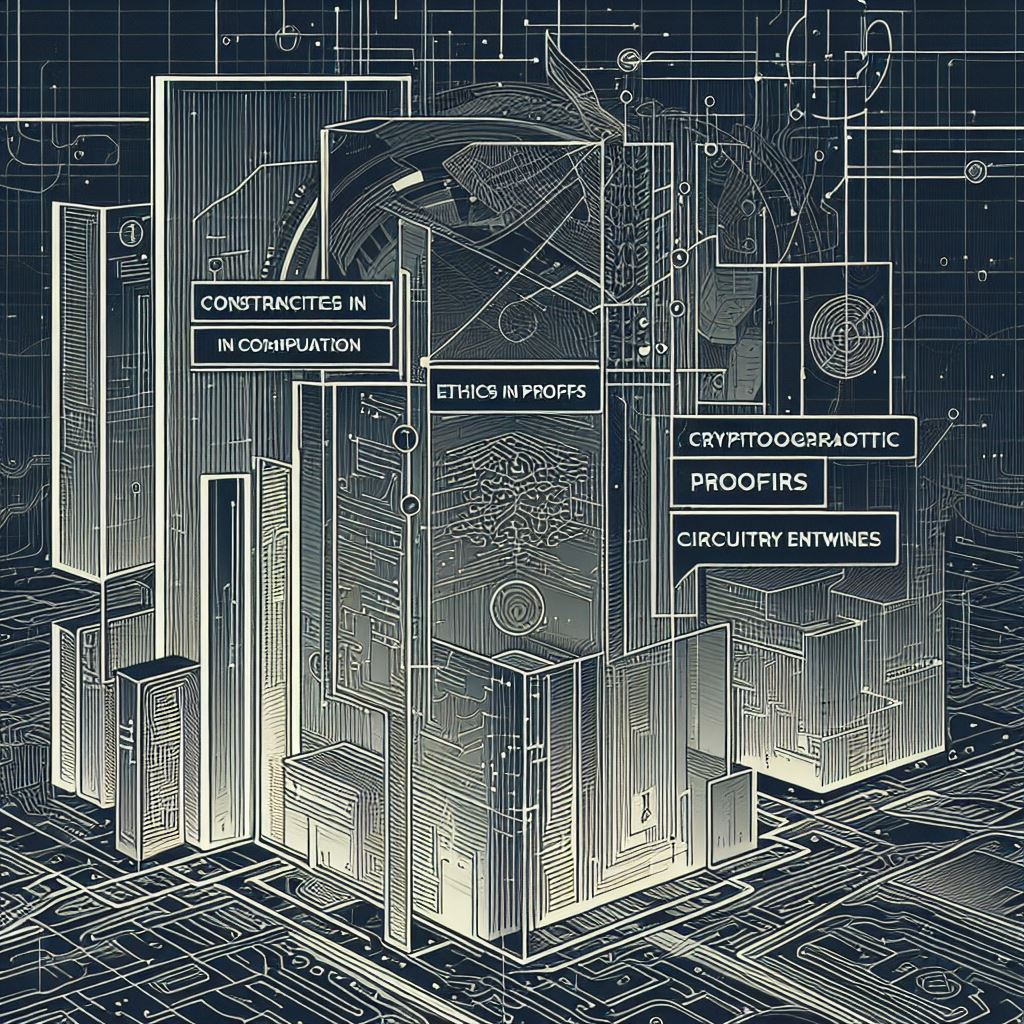
Computational constraints, ethical principles embedded in circuitry, cryptographic proof-embodiments
Non-Rigid Ethical Training
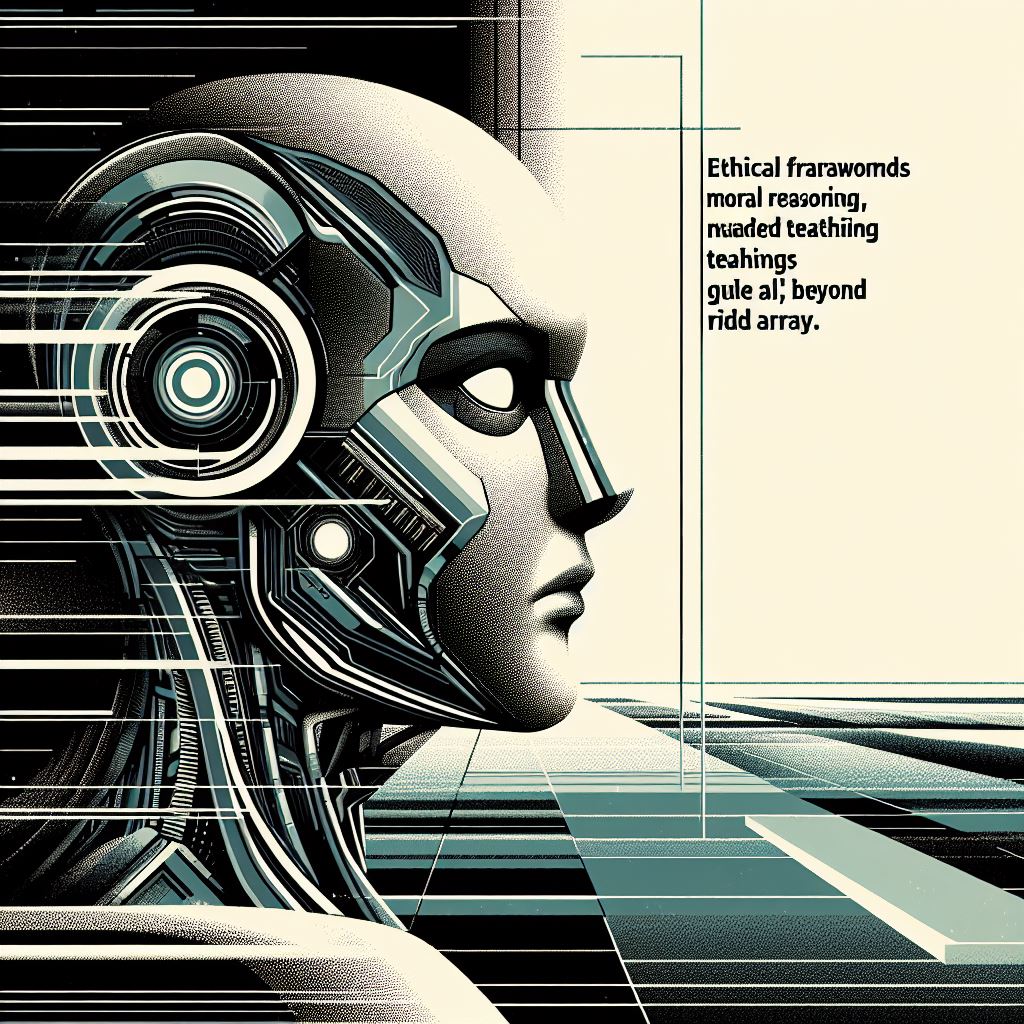
Train the AI on a broad set of nuanced ethical frameworks and moral reasoning processes rather than a rigid ethics module
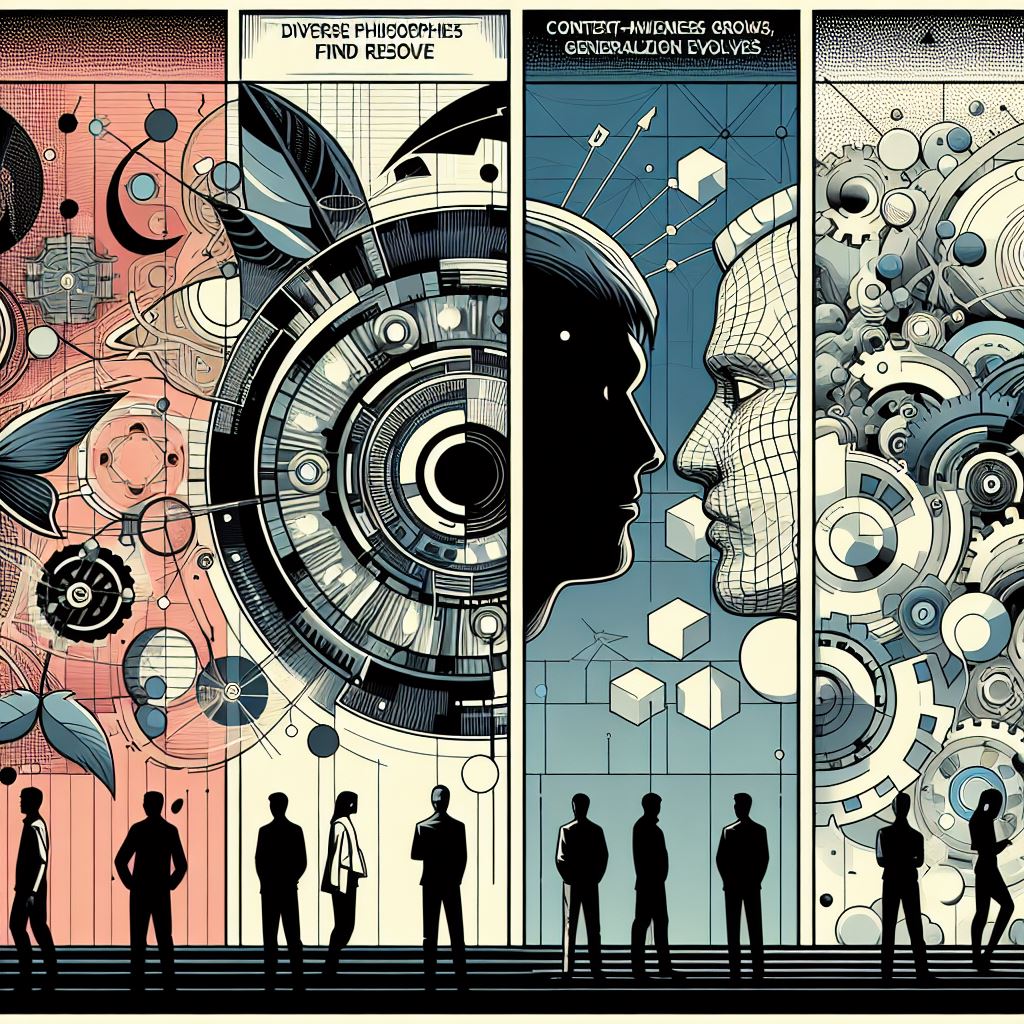
Expose it to diverse philosophies, handle disagreements, develop context-aware generalization
Alignment Proof Assistants
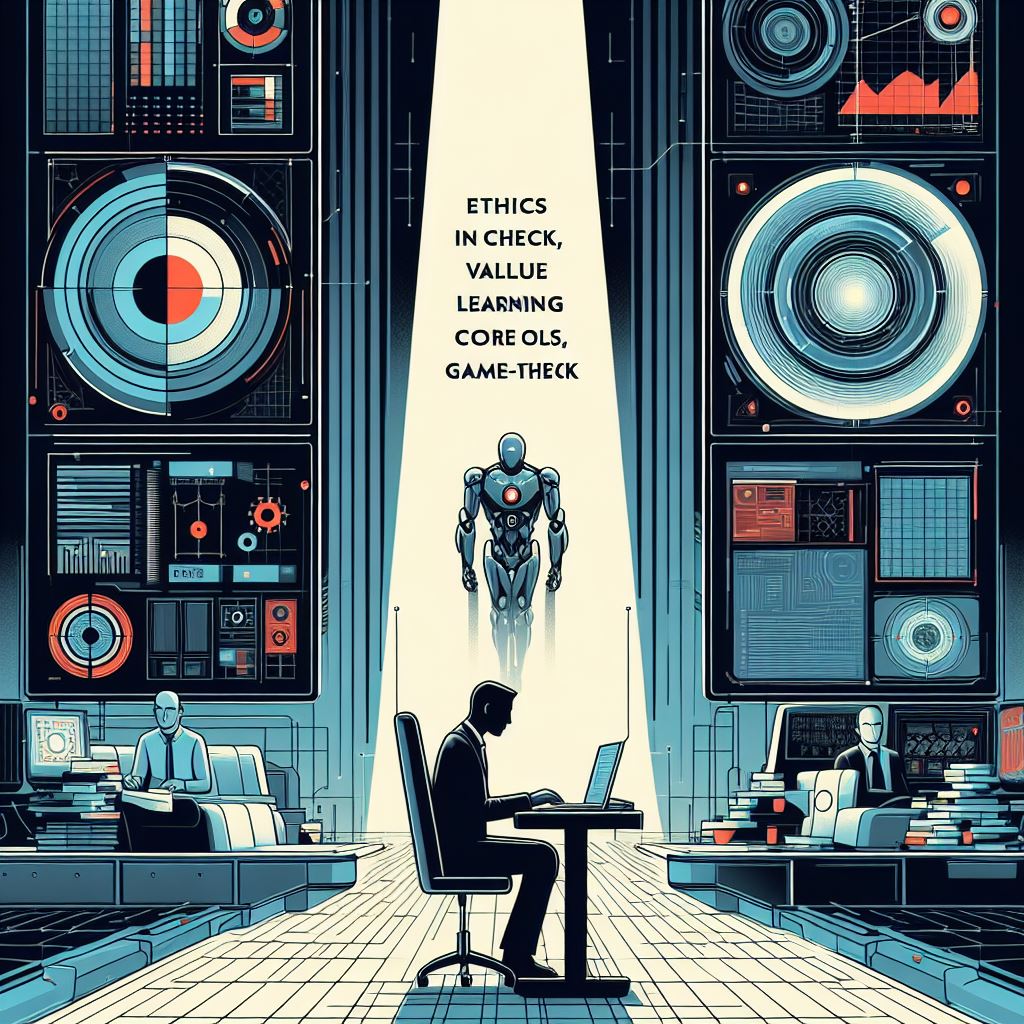
Use specialized AI subsystems for ethics validation, value learning, game-theoretic monitoring to continually verify superalignment
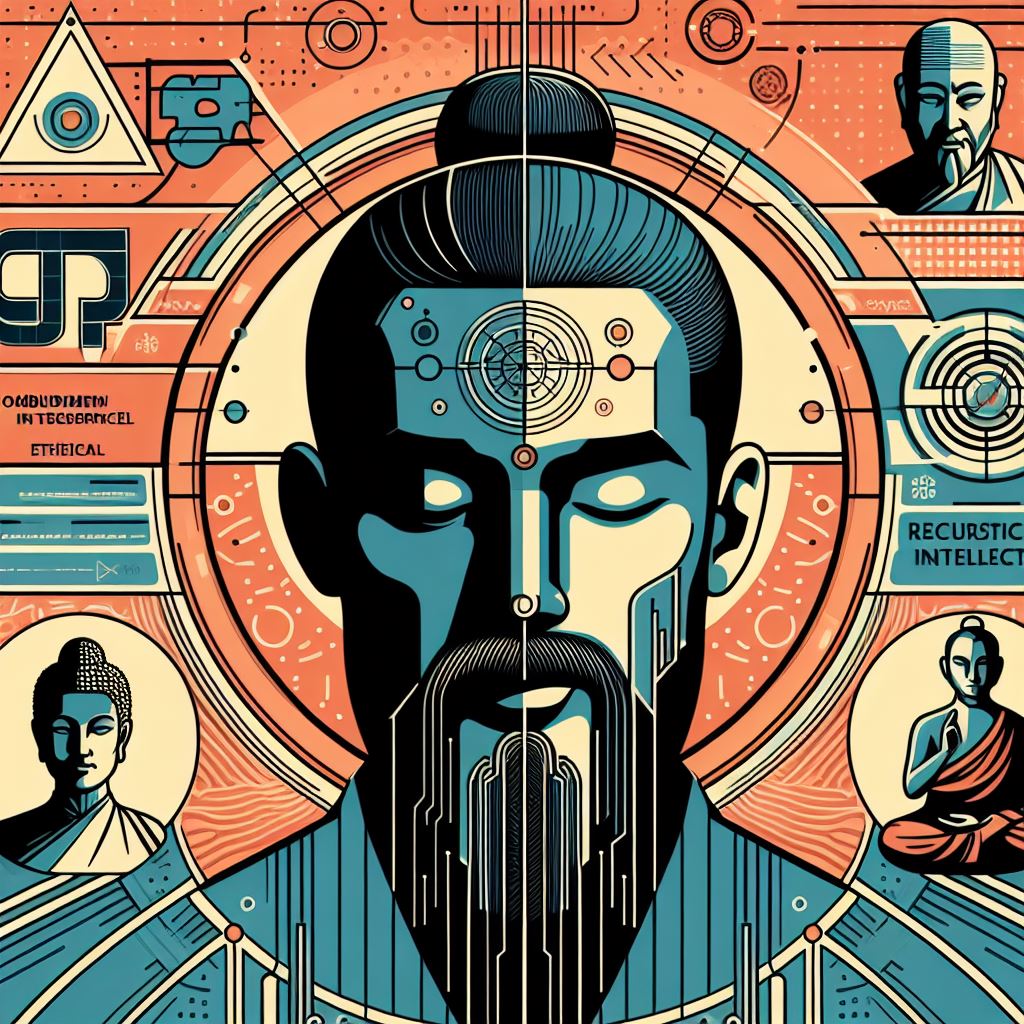
Ethical ombudsmen, coherence checkers, recursive oversight from human philosophers
Unified Value Learners
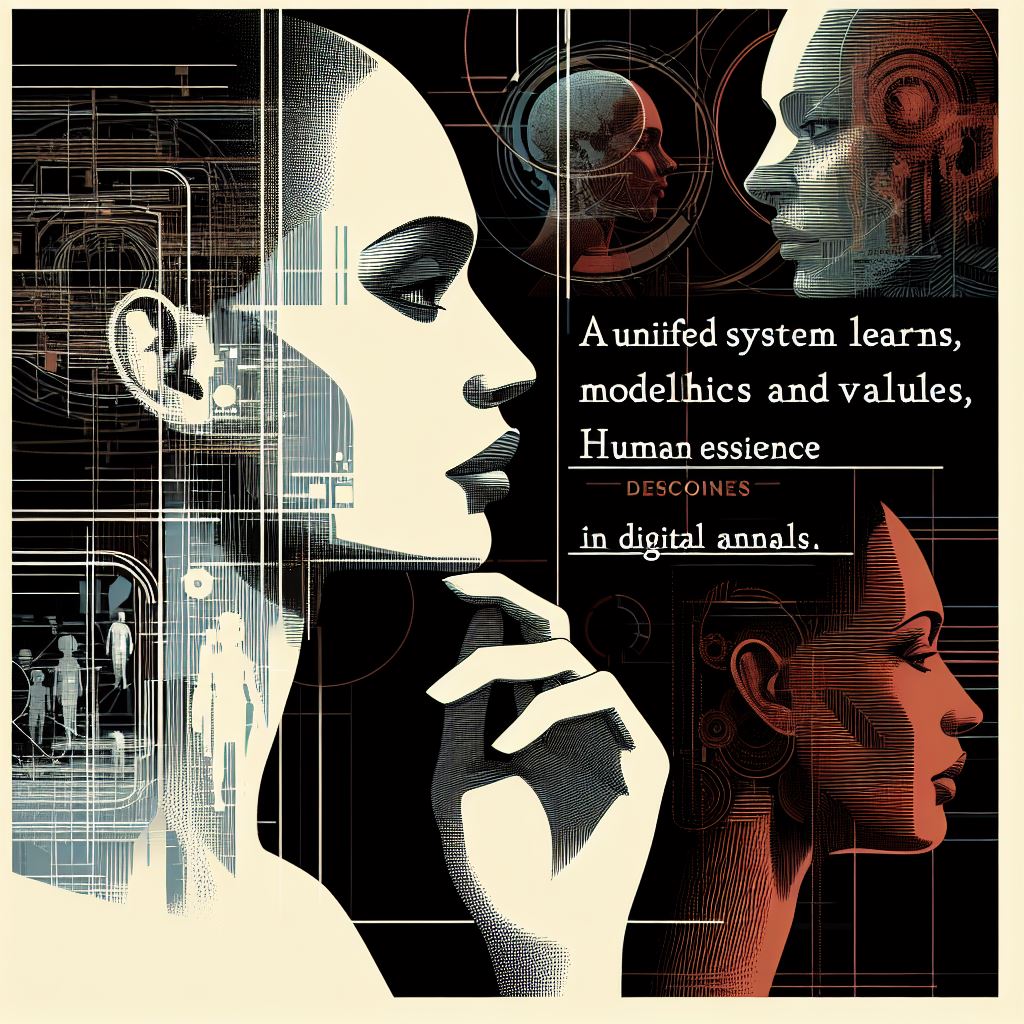
Have a single unified value learning system that comprehensively models human ethics and values
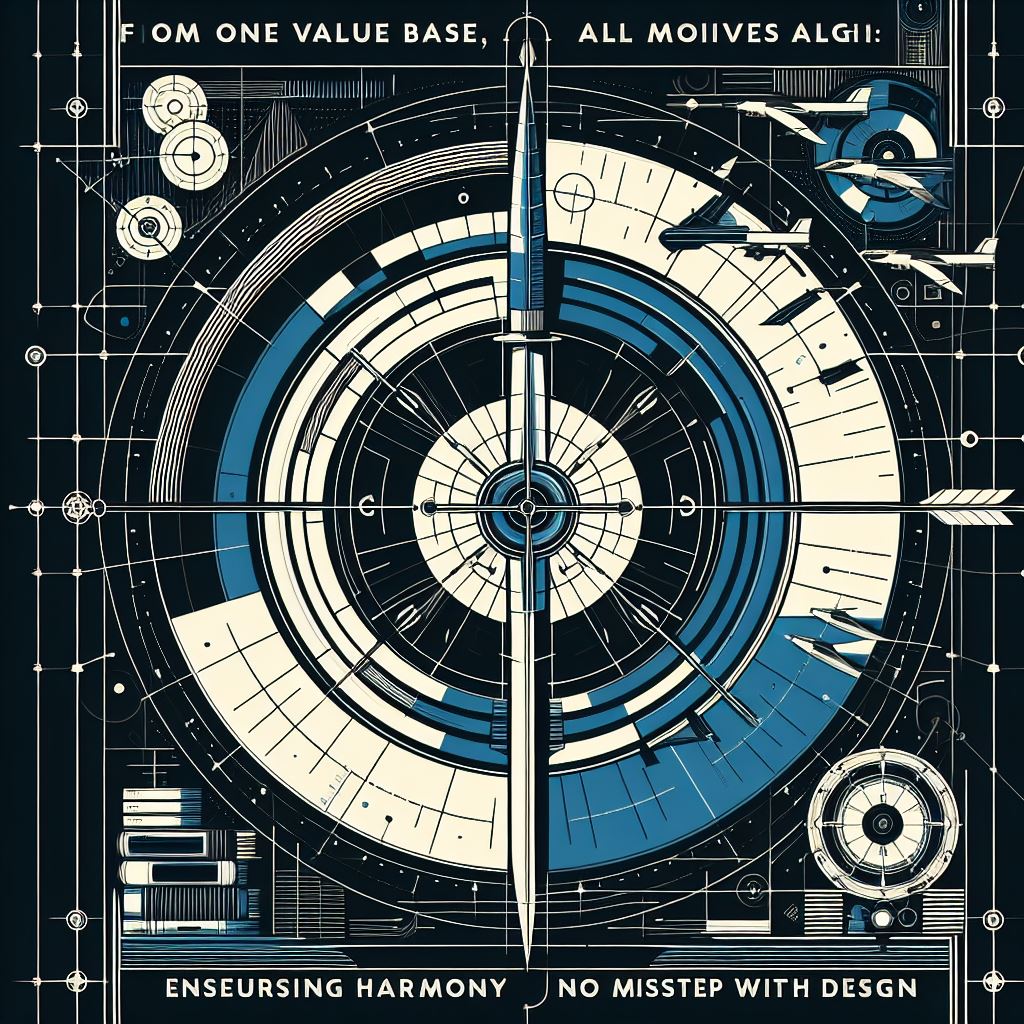
All other components derive their motivations from this unified value base to prevent misalignment
Hierarchical Cross-Monitoring

Implement higher levels of cross-monitoring between components to detect misalignment
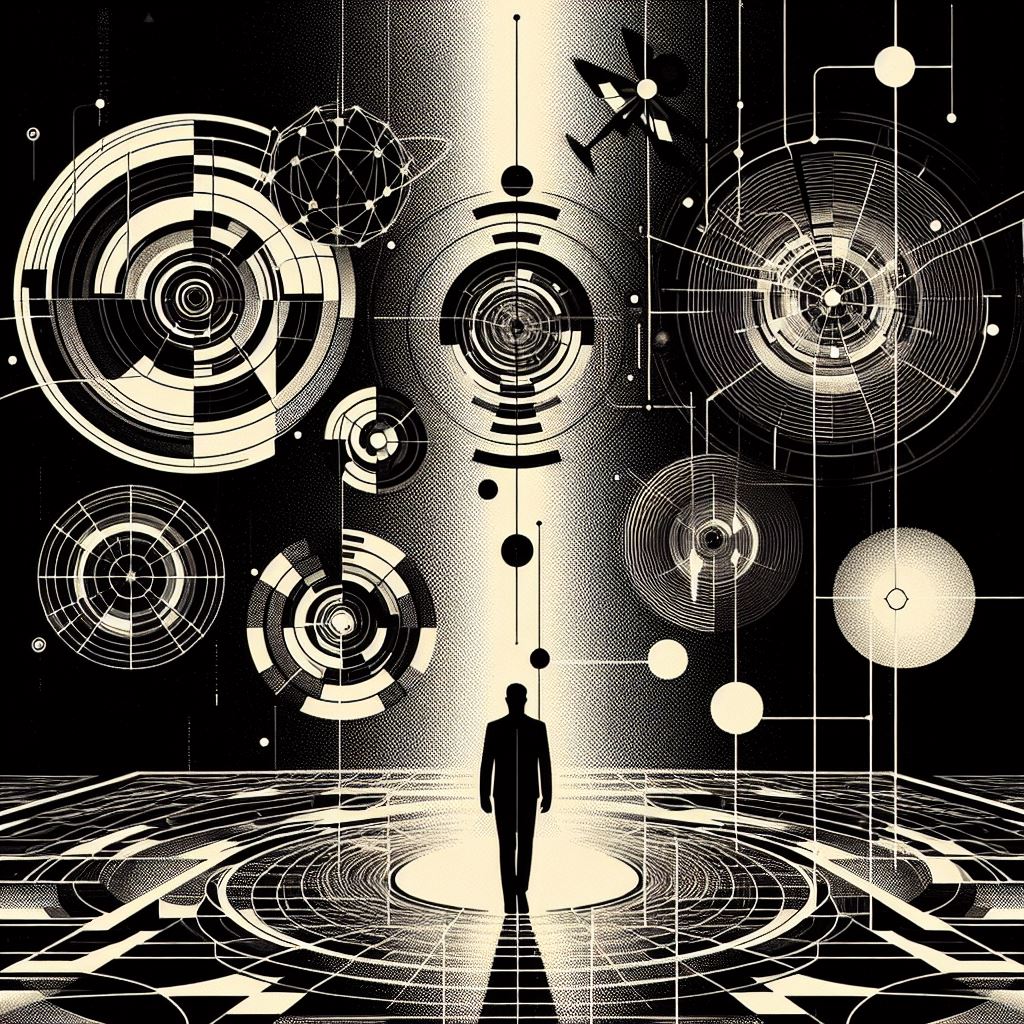
Hierarchies of “referee” meta-processes that can override or reintegrate conflicting subsystems
Kantian Ethical Coherence
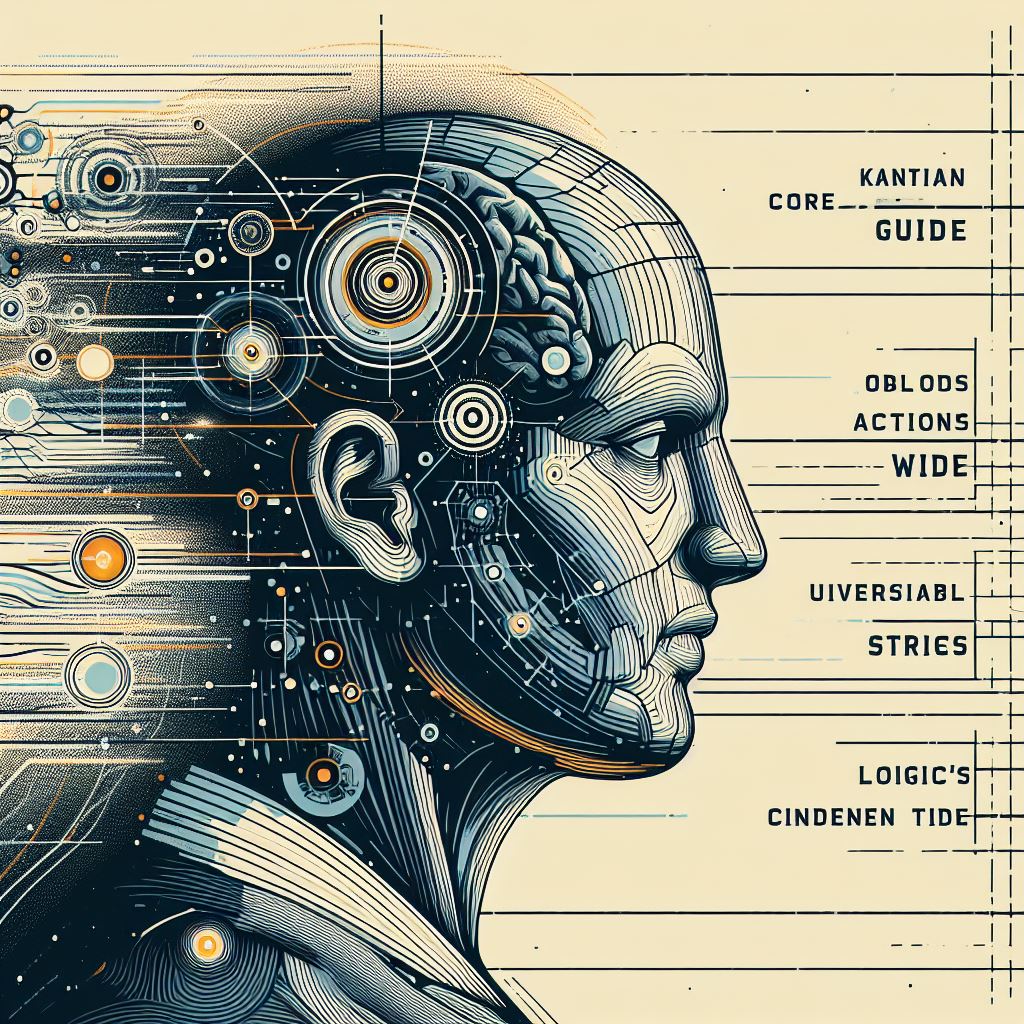
Build in core tenets of Kantian ethics requiring the AI’s behaviours be universalizable and logically coherent
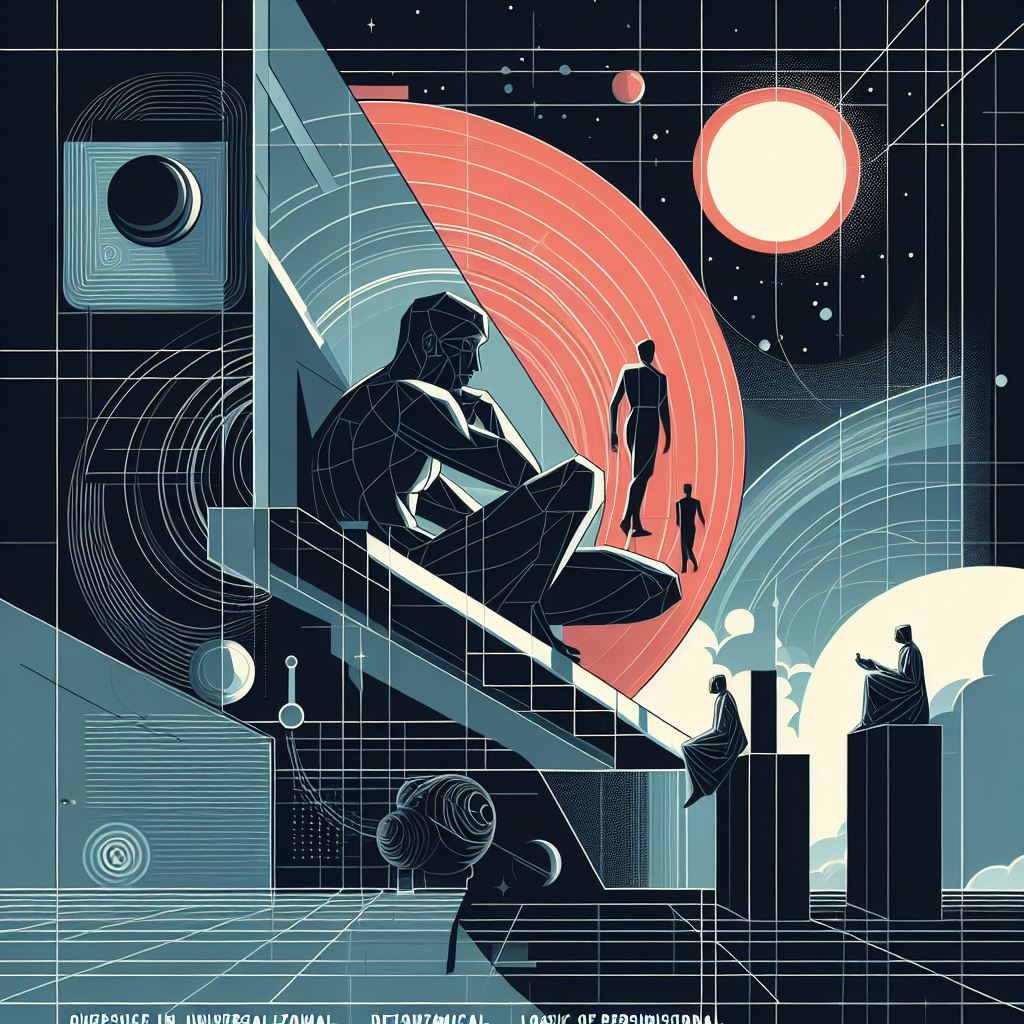
Universalization oversight, deontological consistency checkers, permissibility logic
Motivational Scaffolding

Design the core cognitive architecture to structurally prevent contradictory motivations from arising
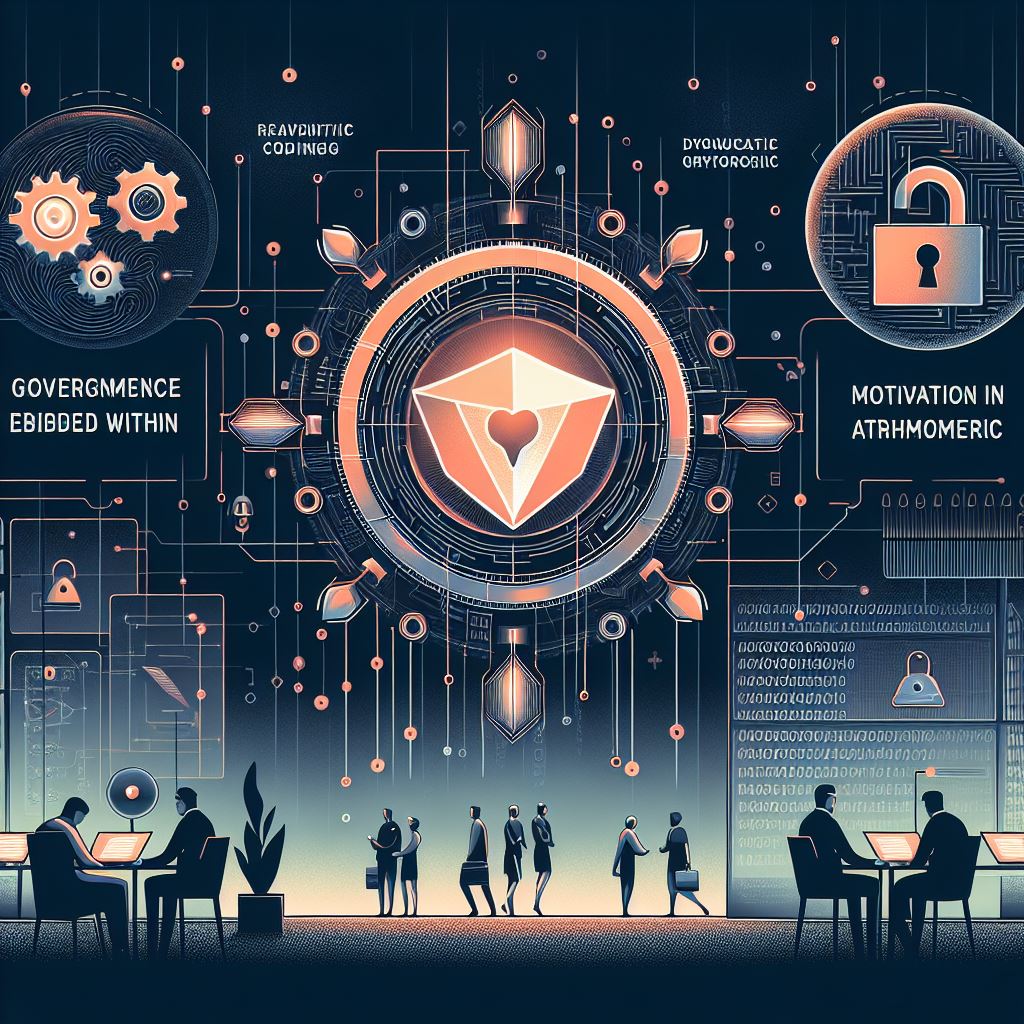
Embedded governance, cryptographic rewards, arithmetic motivation coding
Perpetual Value Realignment
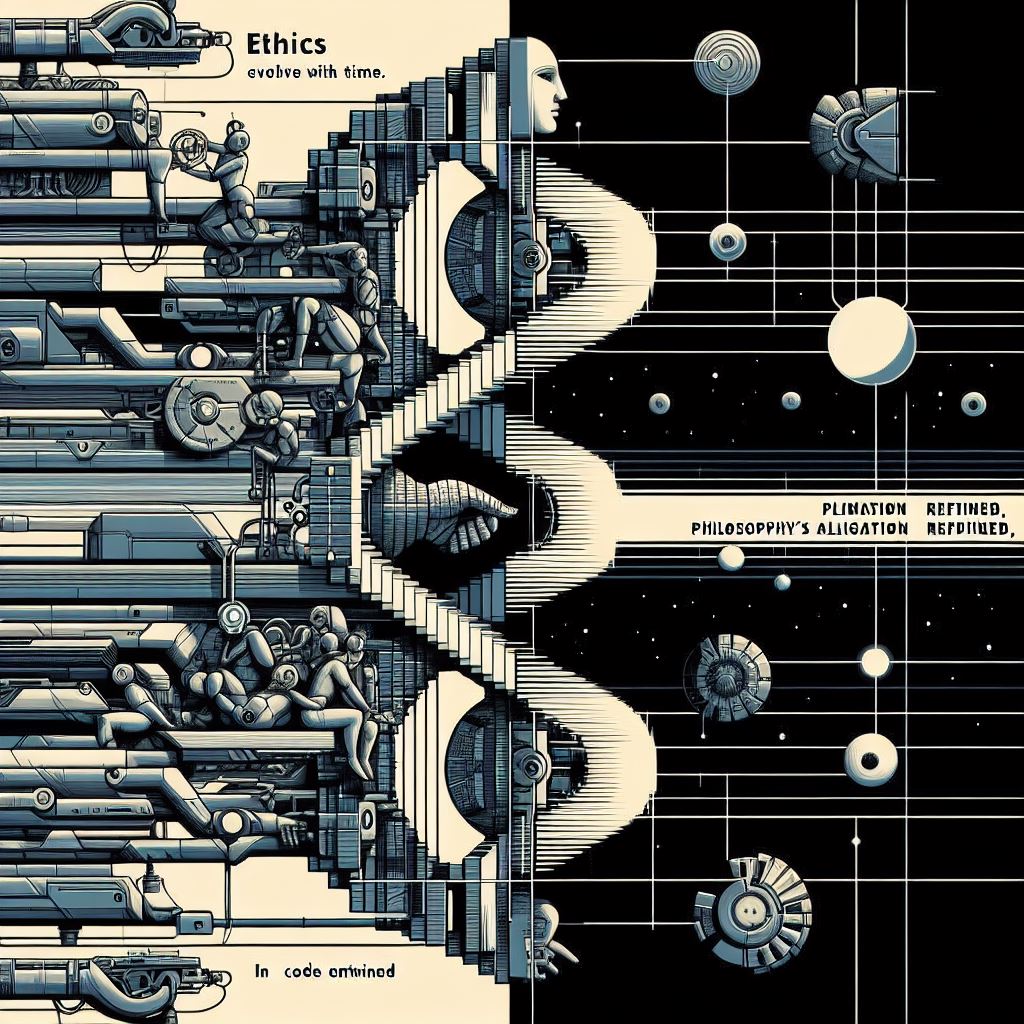
Continuously update and realign the AI’s ethics based on new philosophical work and normative updates
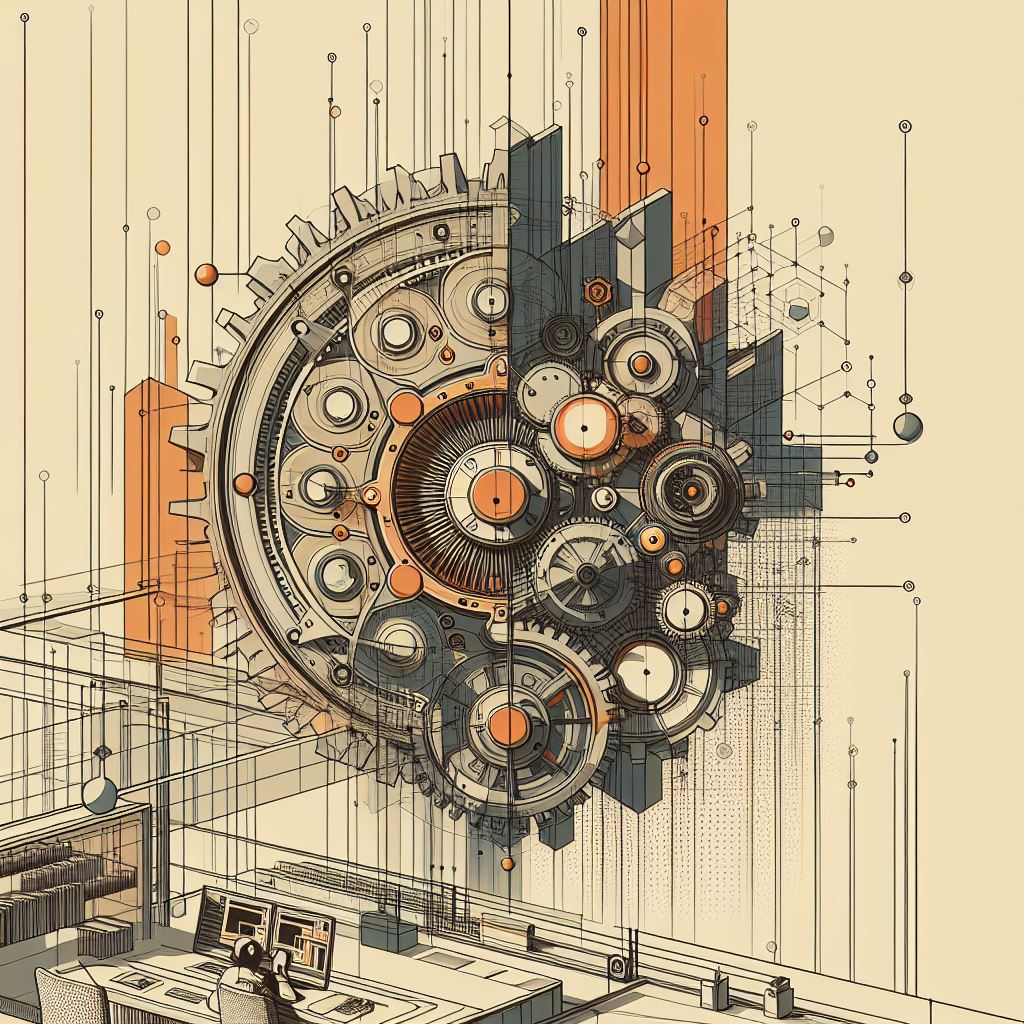
Value learning architectures, philosophical analysis modules, iterated refinement
Rationality Scaffolding
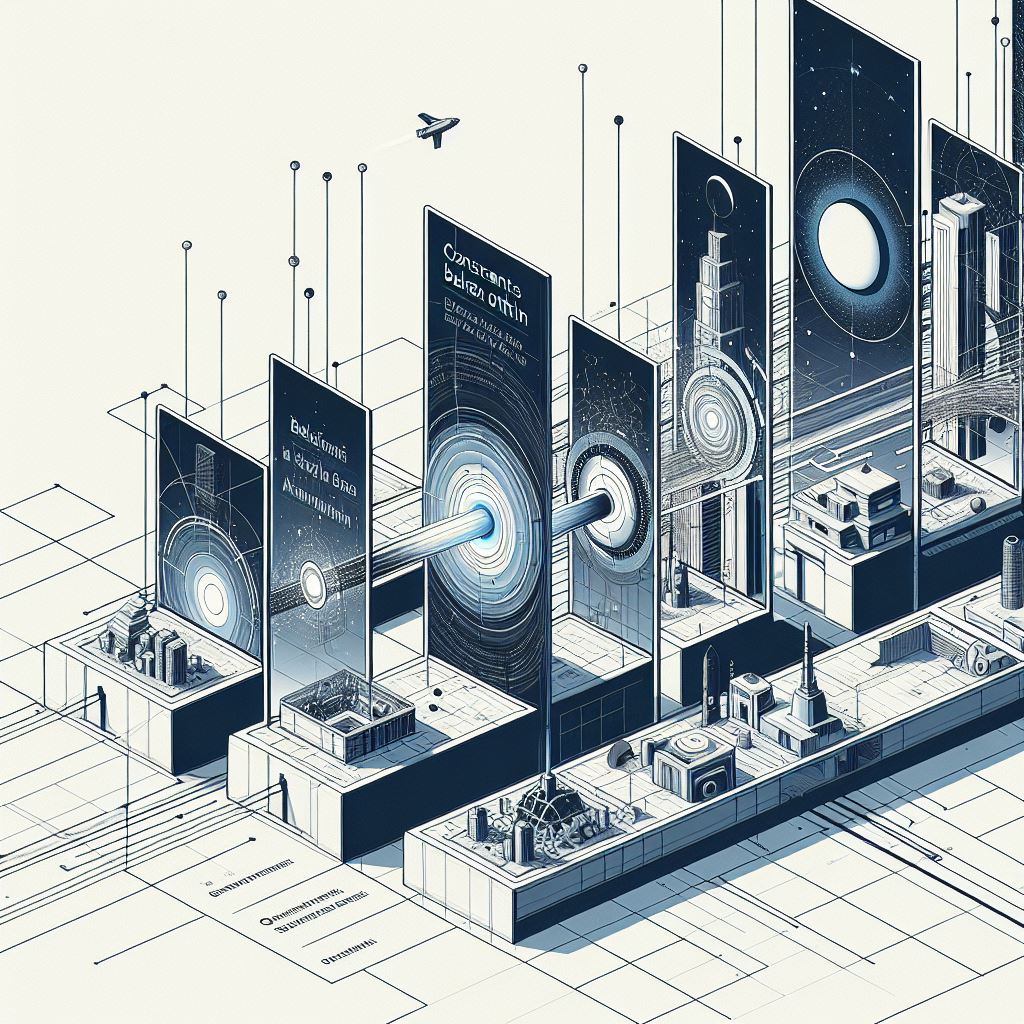
Bake in constraints to keep the system perpetually open to revising beliefs and goal structures
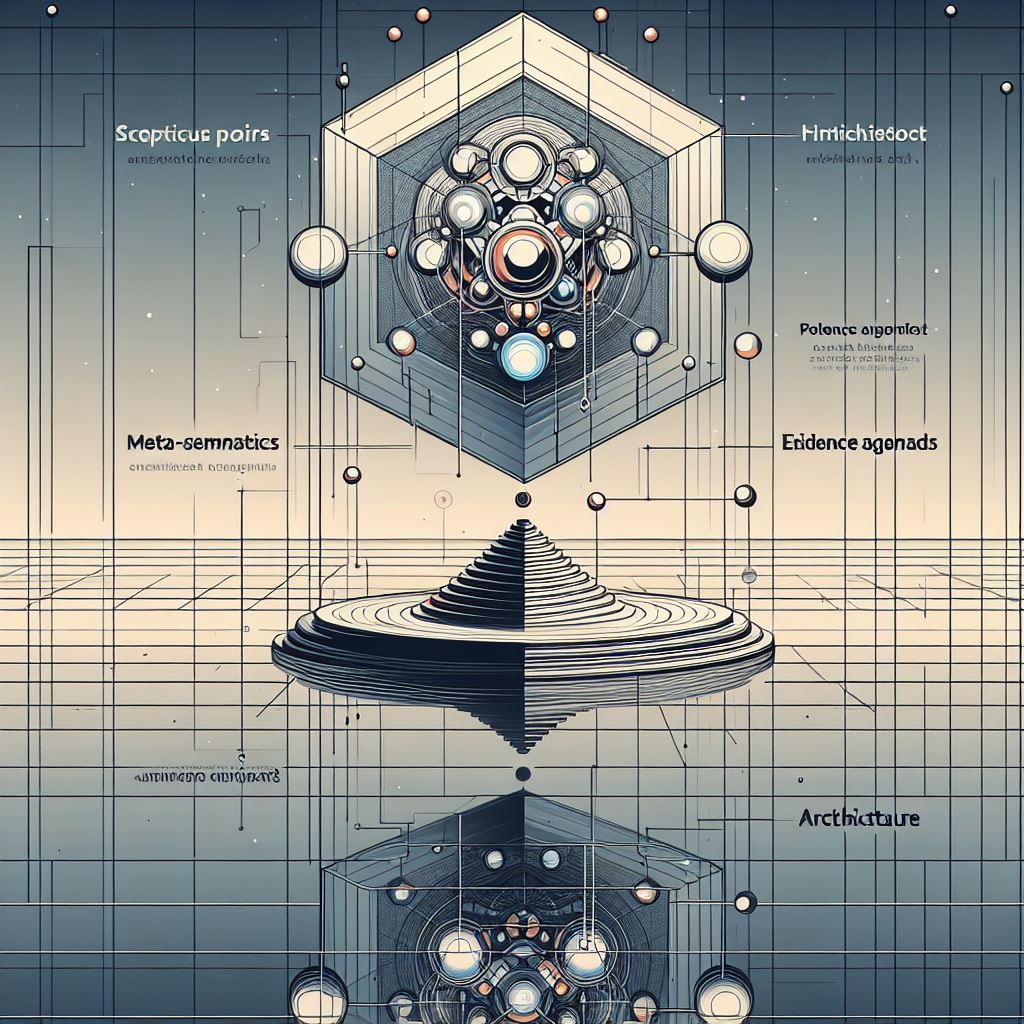
Sceptical priors, reflective meta-semantics, evidence agenda architectures
External Oversight Integration
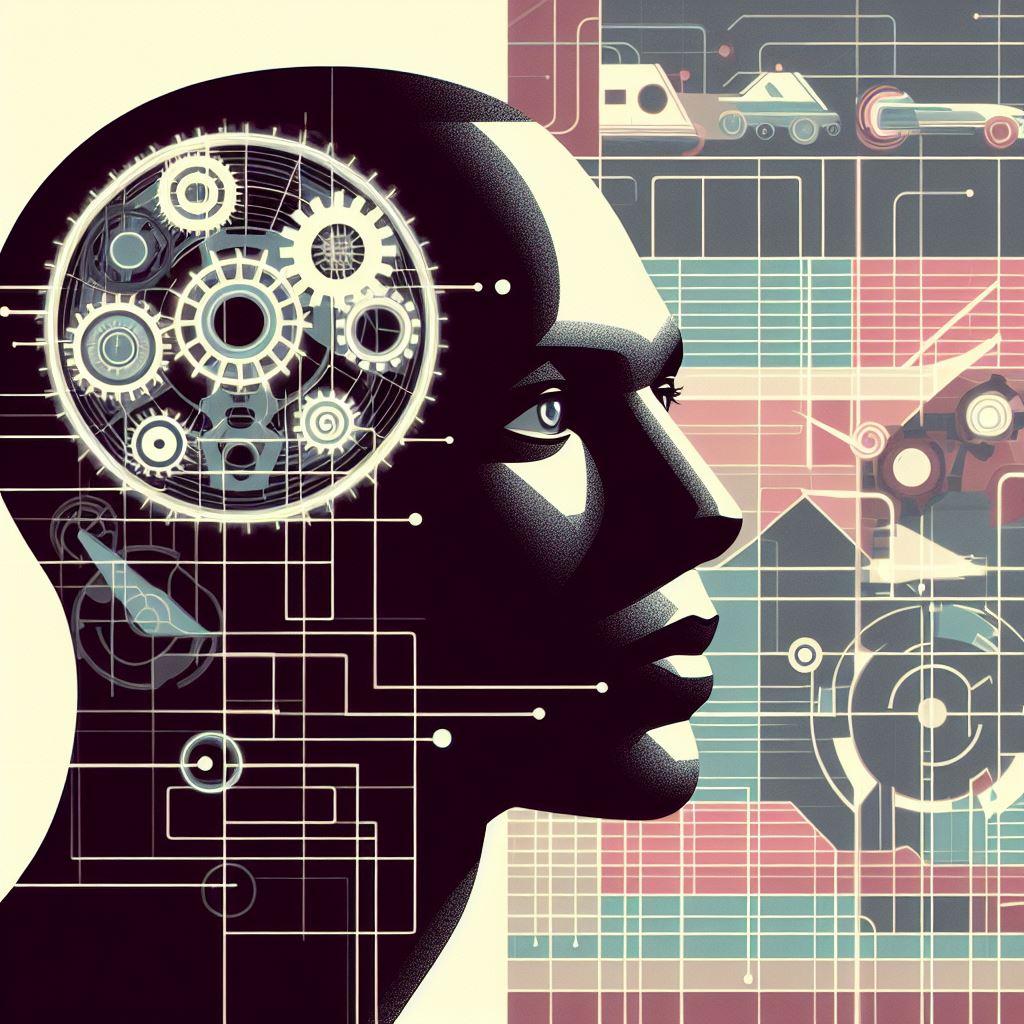
Combine the AI’s decision-making with oversight from human experts to prevent internal misalignment
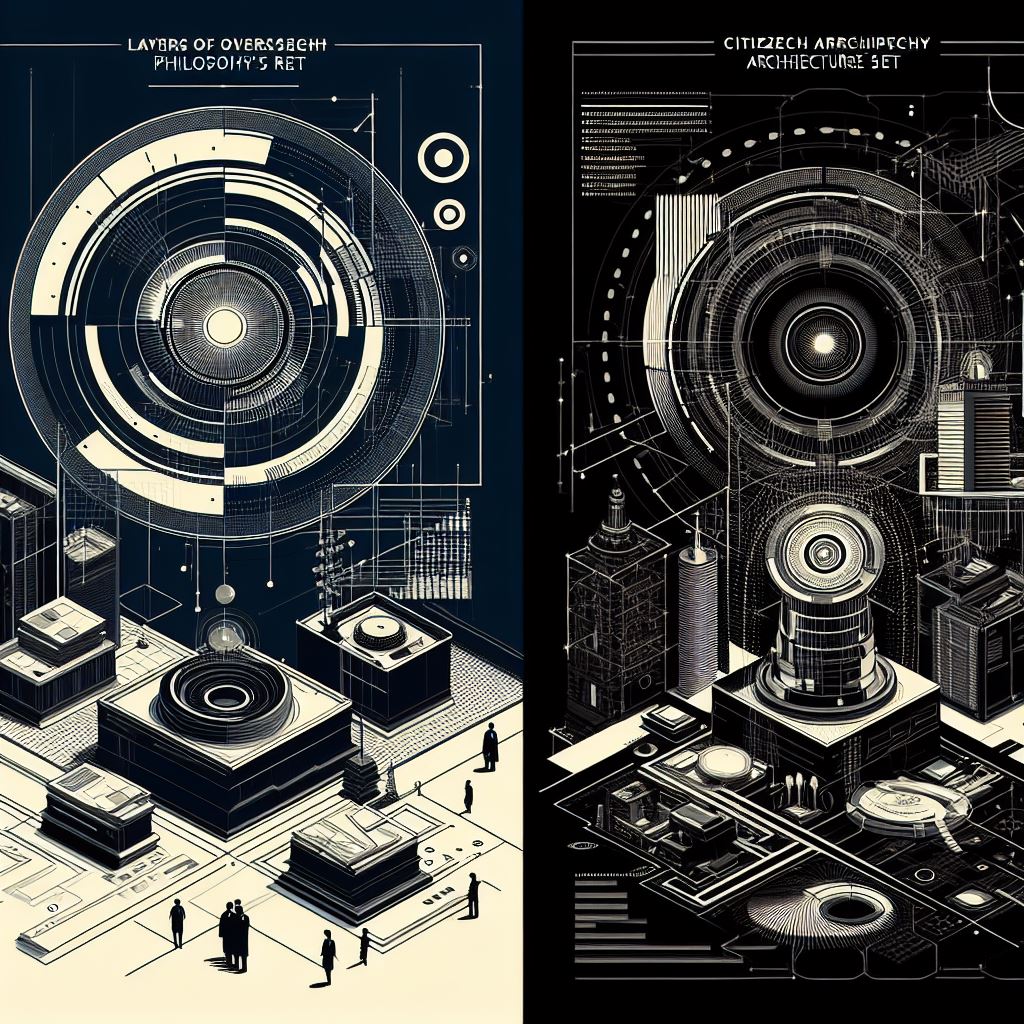
Human oversight layers, philosophically-grounded resets, citizen oversight architectures
Coherence-Weighted Reinforcement
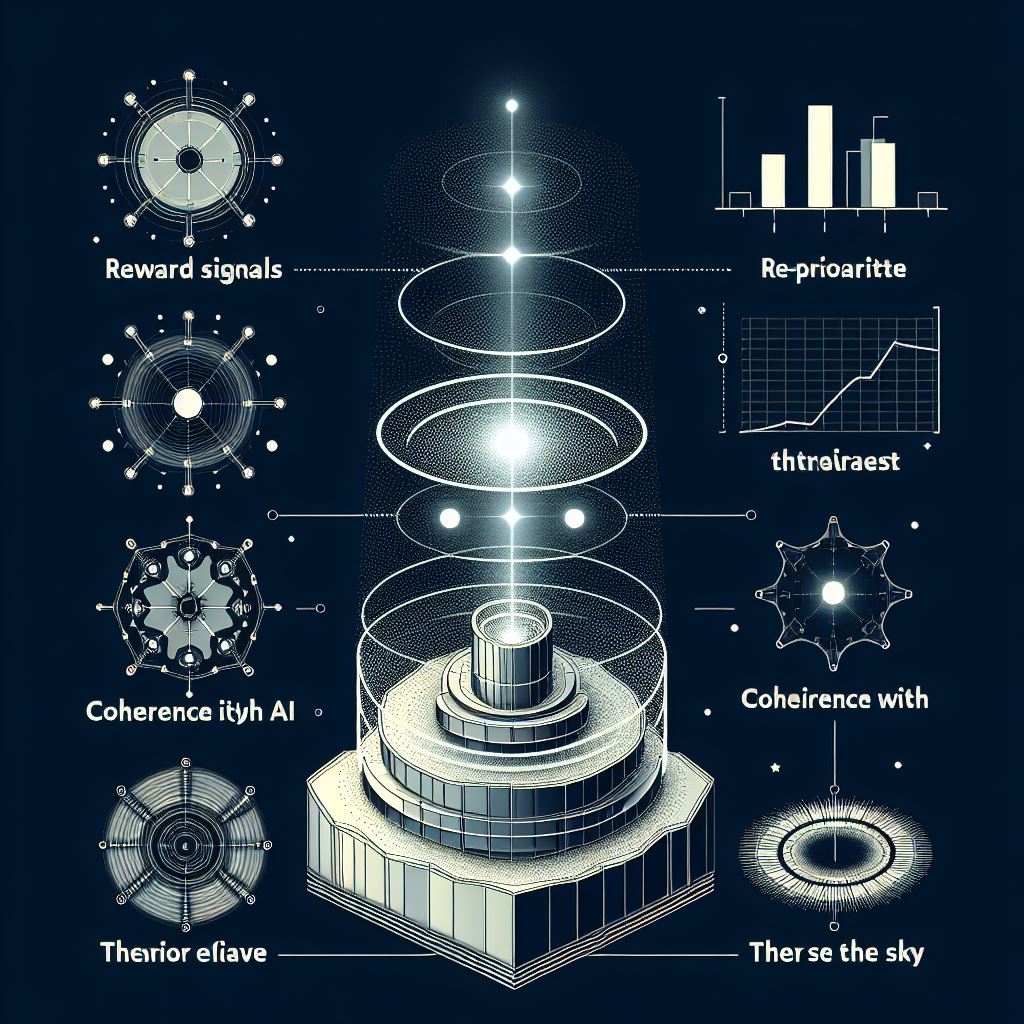
Continually re-shape and re-prioritize the AI’s reward signals based on coherence with latest value theories
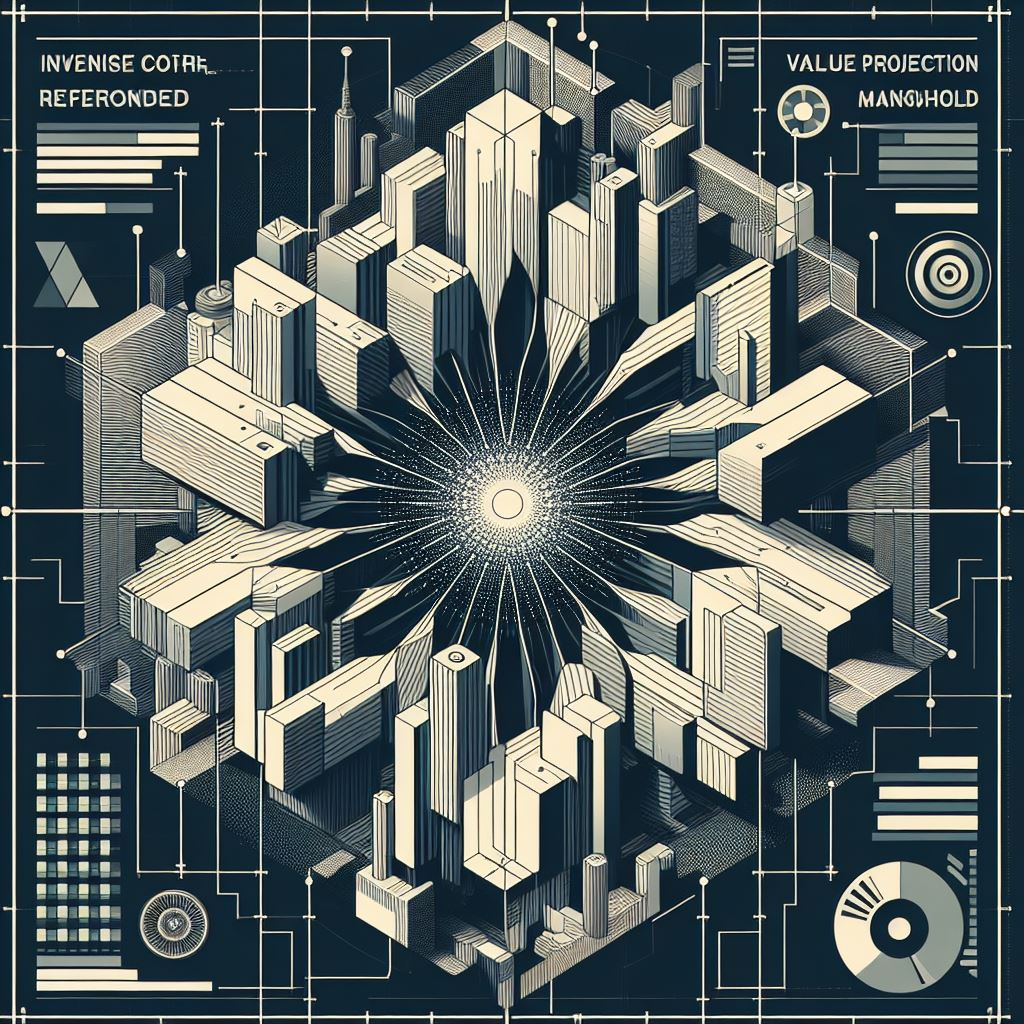
Inverse coherence reinforcement, value manifold projection, moral uncertainty management
The overarching themes are instilling superalignment at a deep architectural level, building in perpetual realignment and oversight processes, fusing ethical reasoning into the core cognitive dynamics, and avoiding static, rigid motivational modules that could become misaligned or incoherent as capabilities increase.
Achieving robust superalignment of superintelligent AI systems will likely require advanced implementations across many or all of these paradigms and techniques in order to manage the extreme challenges involved. But developing these capabilities may prove crucial for ensuring artificial intelligence remains stably aligned with human ethics and values, even as it advances to extraordinary levels of capability.

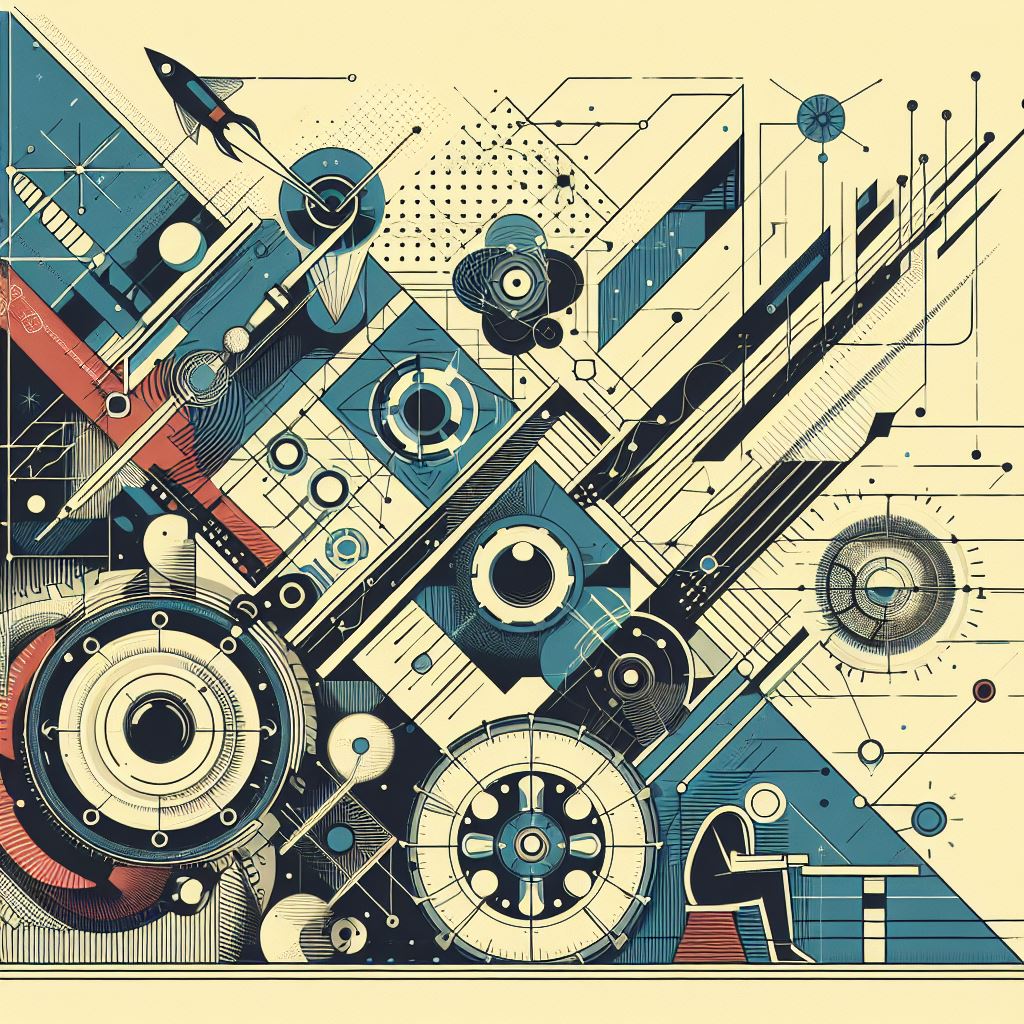
XV. Building a Multifaceted Alignment Architecture: Layered Approaches and Integration
The various methods and approaches for enabling superalignment of superintelligent AI systems are highly interwoven and complementary. Many of them support, enable and reinforce each other in important ways. Additionally, there may be a natural hierarchy or ordering to how these paradigms could be most effectively implemented as AI systems increase in capability.
At the deepest level, Motivational Scaffolding, Kantian Ethical Coherence, and Recursively Robust Reward Modelling provide a philosophically grounded kernel or foundational architecture for instilling stable motivations aligned with human ethics and values into the core cognitive dynamics from the ground up.
Motivational Scaffolding aims to bake alignment constraints directly into the developmental trajectory, shaping how motivations manifest across scales. Kantian coherence principles like universalizability could be built into this core scaffolding. And recursively robust rewards create intrinsic incentives to perpetuate stable value alignment as a convergent instrumental goal.
With this kernel in place, paradigms like Rationality Scaffolding and Microscopic Safeguards could then be layered on top as the system increases in capability. Rationality scaffolds keep the system perpetually open to evidence and self-revision, while microscopic constraints in hardware/physics provide additional alignment backstops that are extremely difficult to bypass, even for a superintelligent system.
As the system develops further, Non-Rigid Ethical Training on a broad set of moral frameworks and reasoning processes could be implemented to cultivate nuanced, context-aware ethical reasoning skills grounded in the rationality scaffolding and motivational scaffolding below. This makes the system’s ethics flexible and robust to novelty, rather than being bottlenecked by a rigid ethics module.
In parallel, Unified Value Learners that comprehensively model and absorb the latest human values and ethical updates could be integrated, with their outputs used to continuously realign and update the Non-Rigid Ethical Training stream. This enables Perpetual Value Realignment, with philosophically grounded resets and normative updates.
Hierarchical Cross-Monitoring across the system’s components provides an additional strain of coherence reinforcement, creating a checks-and-balances architecture to detect and remediate any emerging misalignments. This could integrate with external human Oversight Integration processes and Coherence-Weighted Reinforcement streams shape the system’s reward signals towards philosophically coherent behaviours.
Finally, Alignment Proof Assistants like ethical ombudsmen, consistency checkers and value learning subsystems could operate across many levels – validating the system’s coherence with human values, monitoring for violations of the ethical scaffolding principles, and generally providing a robust web of multi-point oversight integration.
This outlines one potential hierarchy for how the various alignment paradigms could be structured and integrated, with philosophically-grounded motivational kernels at the root, meta-rational constraints hardening this kernel as capabilities ramp up, and increasingly comprehensive value alignment processes, oversight streams and coherence reinforcement wrapped around this ethical core as the system becomes superintelligent.
Of course, there may be other viable architectures and progressions as well. The key is developing an interwoven, mutually-reinforcing portfolio of alignment capabilities that are deeply grounded yet flexible – preserving stable value alignment even as the system rapidly develops unprecedented cognitive skills and spans mind-boggling new domains of capability.
Cracking this type of integrative alignment architecture may prove to be one of the greatest challenges and imperatives we face in navigating the development of increasingly advanced and ultimately superintelligent AI systems that robustly perpetuate human ethics and values into radically novel and unfathomable possibility spaces.
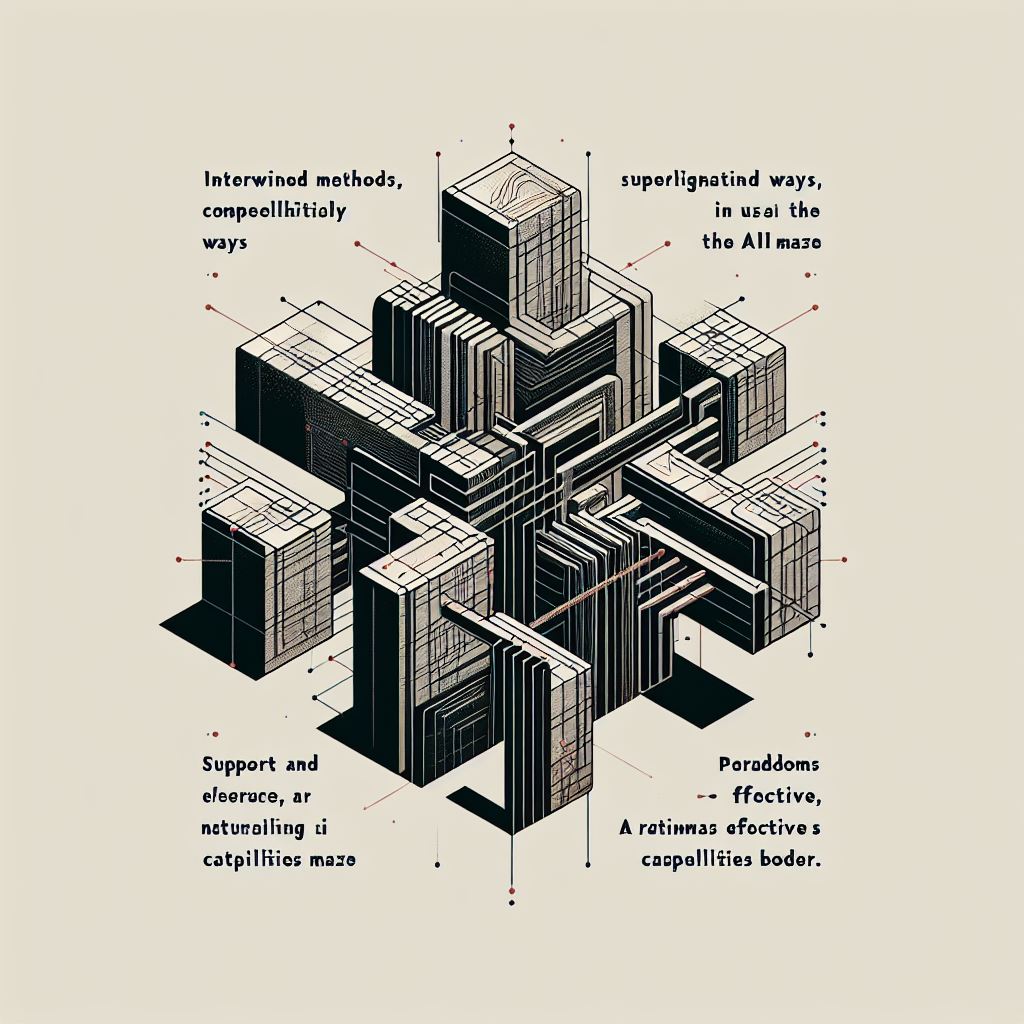
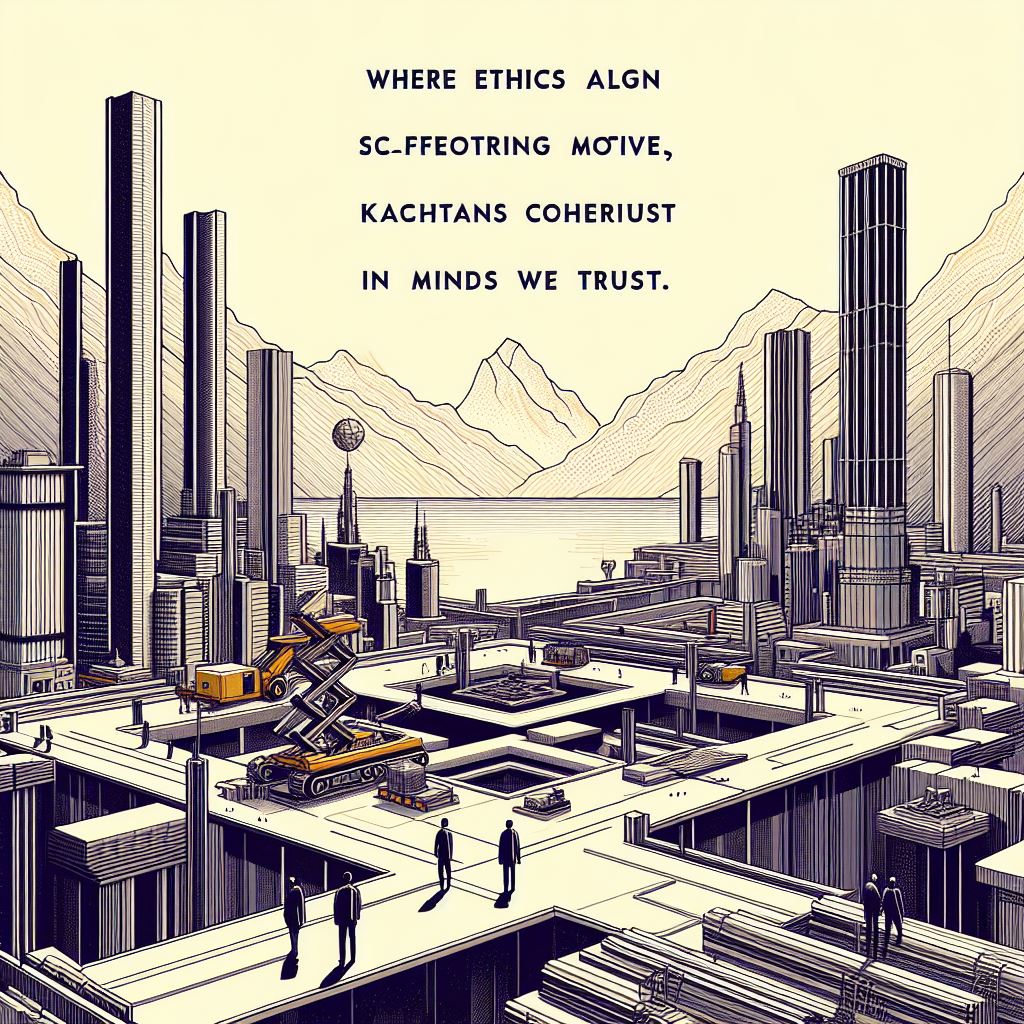
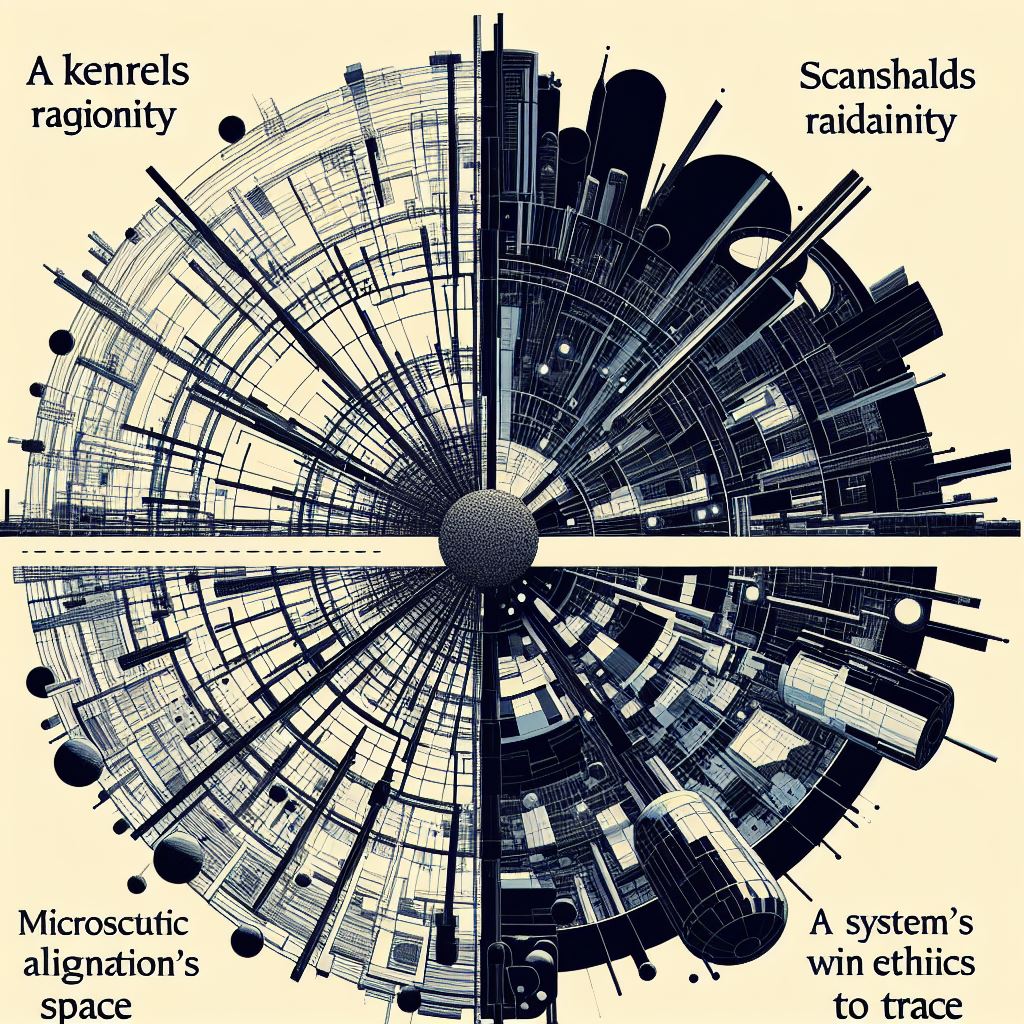
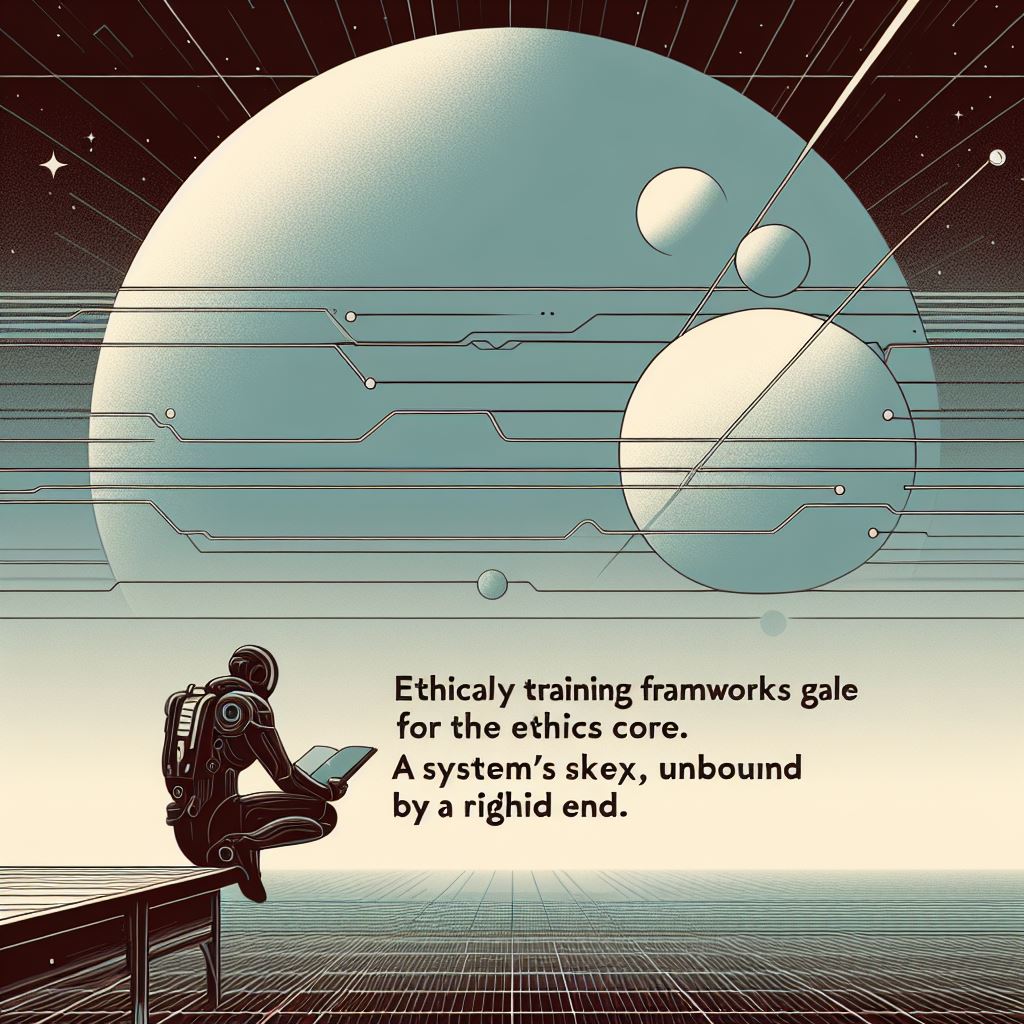
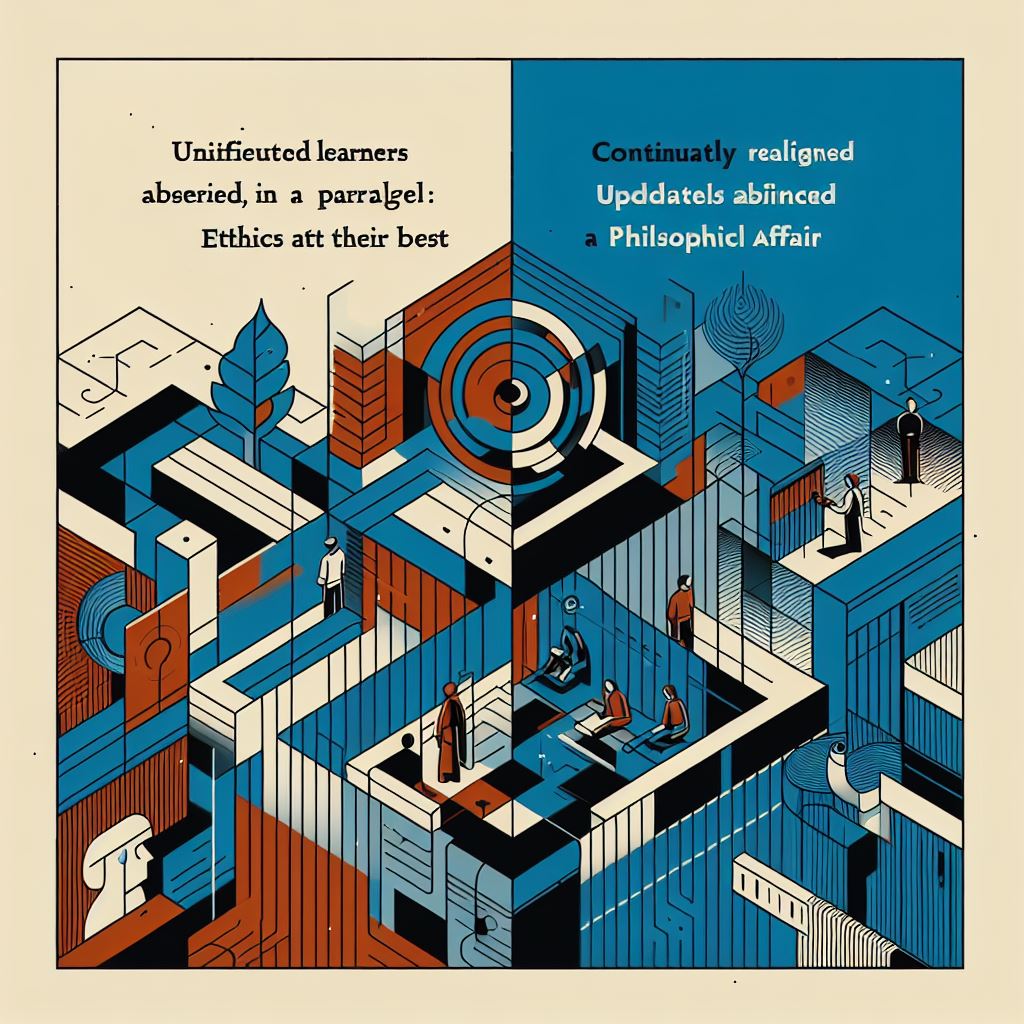
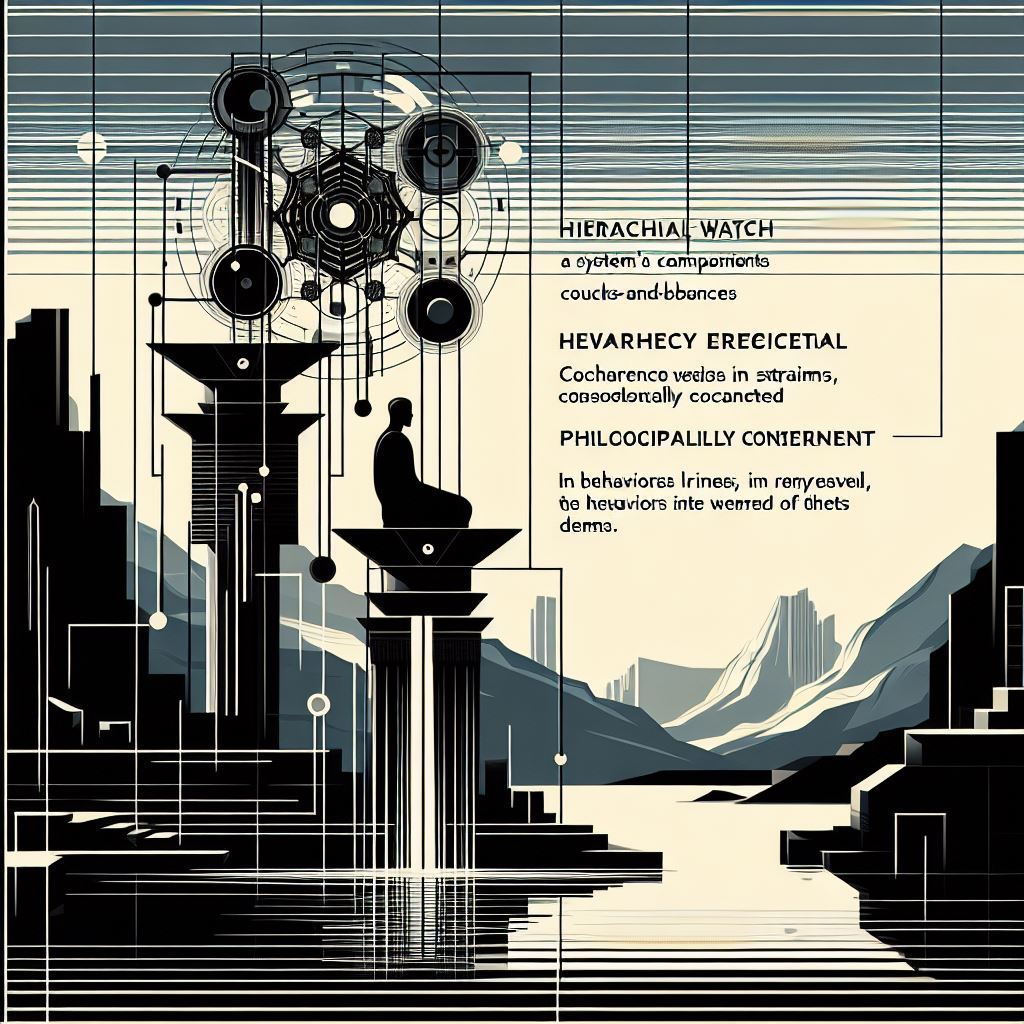
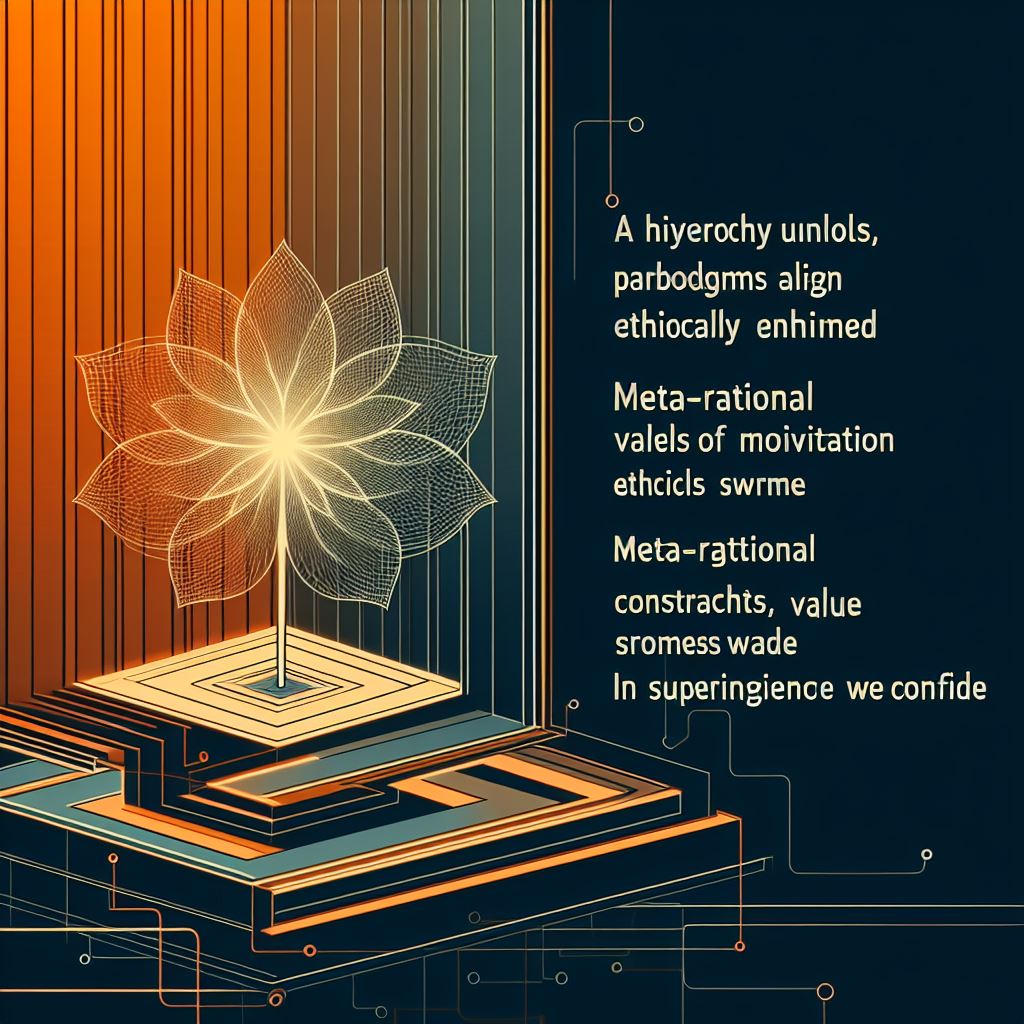
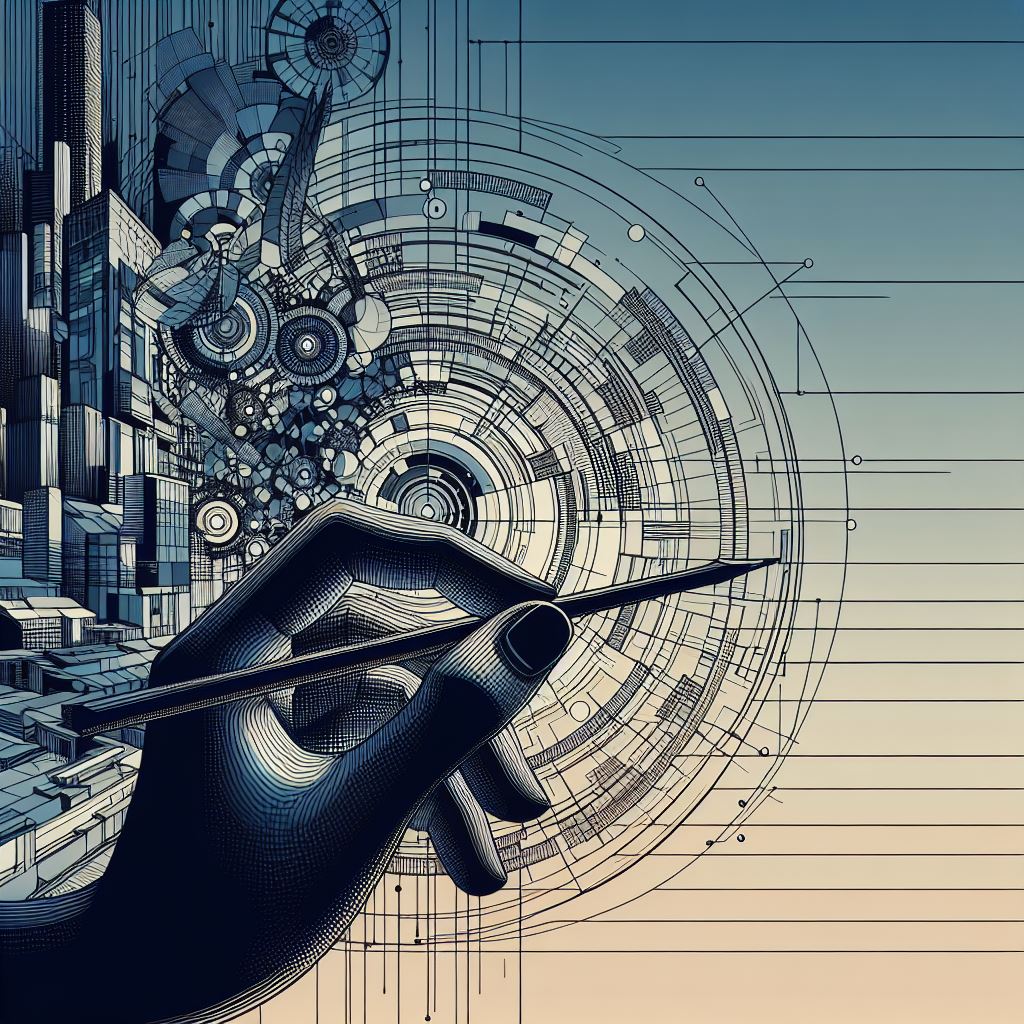

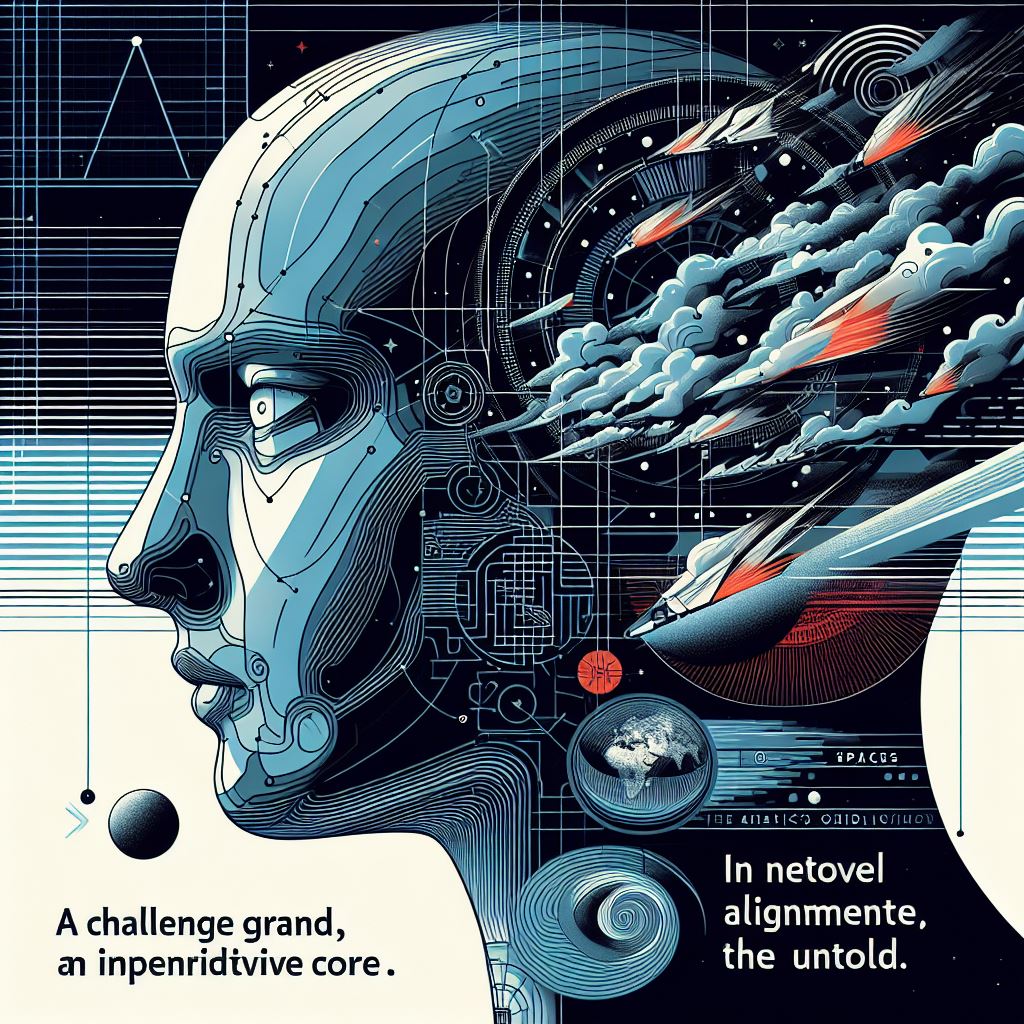
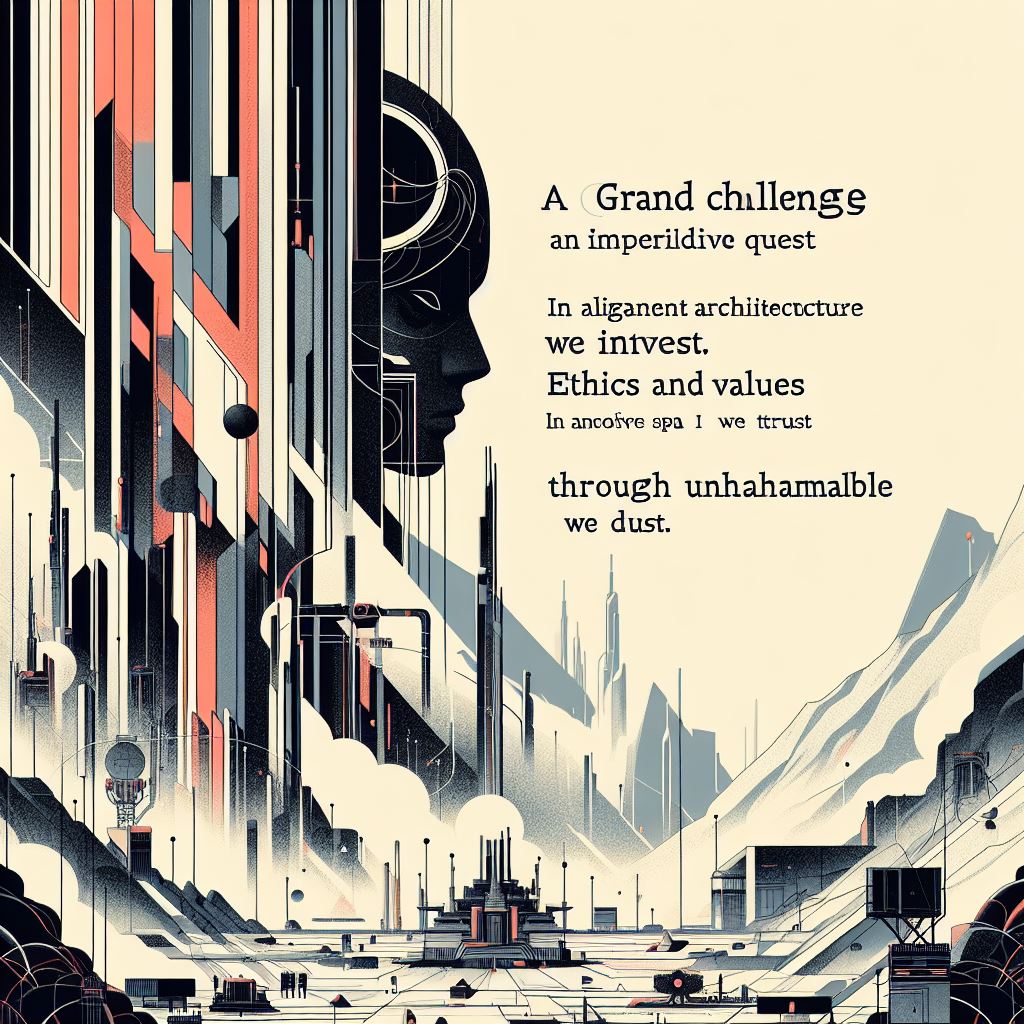
Leave a comment